A Deep Learning Genome-Mining Strategy Improves Biosynthetic Gene Cluster Prediction
bioRxiv(2018)
摘要
Natural products represent a rich reservoir of small molecule drug candidates utilized as antimicrobial drugs, anticancer therapies, and immunomodulatory agents. These molecules are microbial secondary metabolites synthesized by co-localized genes termed Biosynthetic Gene Clusters (BGCs). The increase in full microbial genomes and similar resources has led to development of BGC prediction algorithms, although their precision and ability to identify novel BGC classes could be improved. Here we present a deep learning strategy (DeepBGC) that offers more accurate BGC identification and an improved ability to extrapolate and identify novel BGC classes compared to existing tools. We supplemented this with downstream random forest classifiers that accurately predicted BGC product classes and potential chemical activity. Application of DeepBGC to bacterial genomes uncovered previously undetectable BGCs that may code for natural products with novel biologic activities. The improved accuracy and classification ability of DeepBGC represents a significant step forward for in-silico BGC identification.
更多查看译文
关键词
DeepBGC,Bidirectional LSTM,pfam2vec,Biosynthetic Gene Clusters
AI 理解论文
溯源树
样例
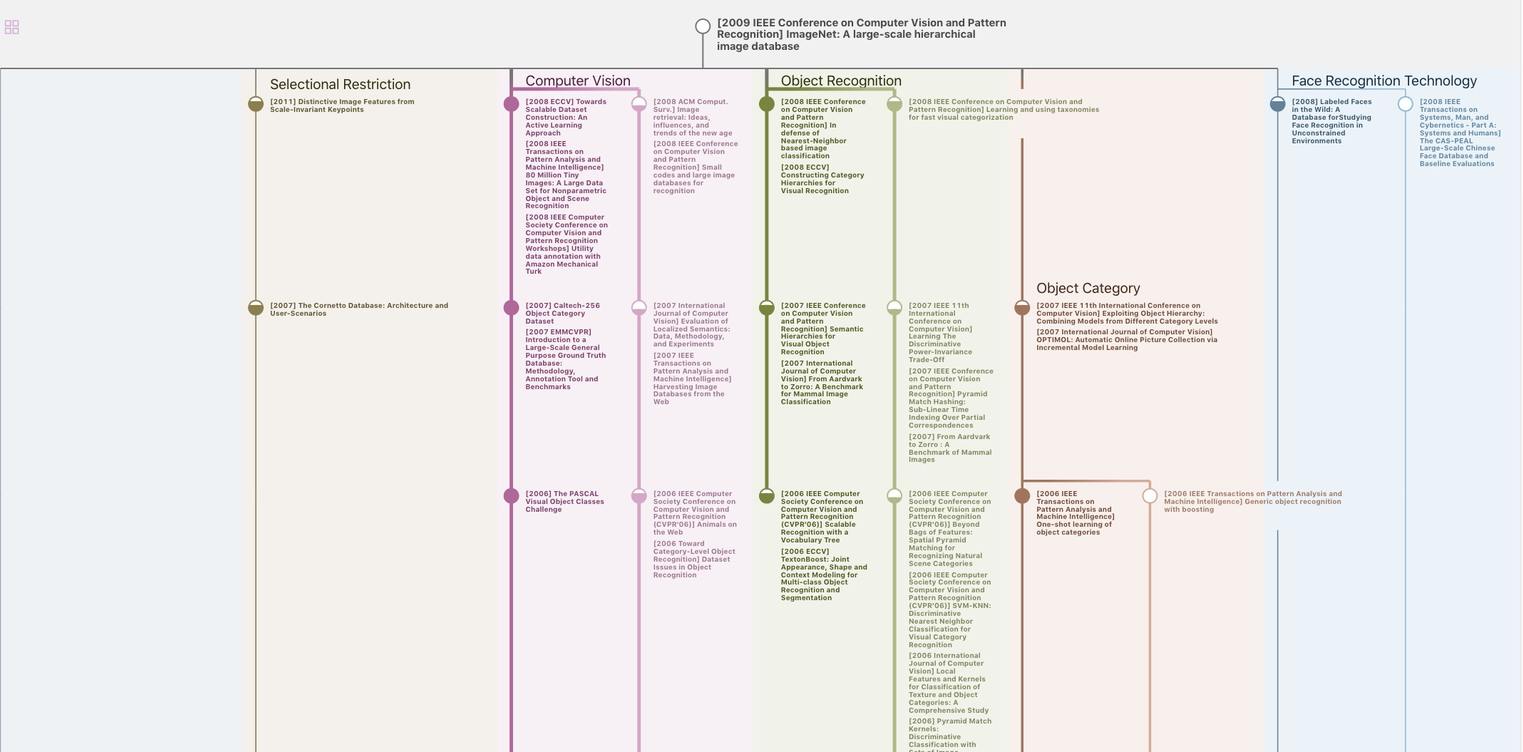
生成溯源树,研究论文发展脉络
Chat Paper
正在生成论文摘要