sGDML: Constructing accurate and data efficient molecular force fields using machine learning
Computer Physics Communications(2019)
摘要
We present an optimized implementation of the recently proposed symmetric gradient domain machine learning (sGDML) model. The sGDML model is able to faithfully reproduce global potential energy surfaces (PES) for molecules with a few dozen atoms from a limited number of user-provided reference molecular conformations and the associated atomic forces. Here, we introduce a Python software package to reconstruct and evaluate custom sGDML force fields (FFs), without requiring in-depth knowledge about the details of the model. A user-friendly command-line interface offers assistance through the complete process of model creation, in an effort to make this novel machine learning approach accessible to broad practitioners. Our paper serves as a documentation, but also includes a practical application example of how to reconstruct and use a PBE0+MBD FF for paracetamol. Finally, we show how to interface sGDML with the FF simulation engines ASE (Larsen et al., 2017) and i-PI (Kapil et al., 2019) to run numerical experiments, including structure optimization, classical and path integral molecular dynamics and nudged elastic band calculations.
更多查看译文
关键词
Machine learning potential,Machine learning force field,Ab initio molecular dynamics,Path integral molecular dynamics,Coupled cluster calculations,Molecular property prediction,Quantum chemistry,Gradient domain machine learning
AI 理解论文
溯源树
样例
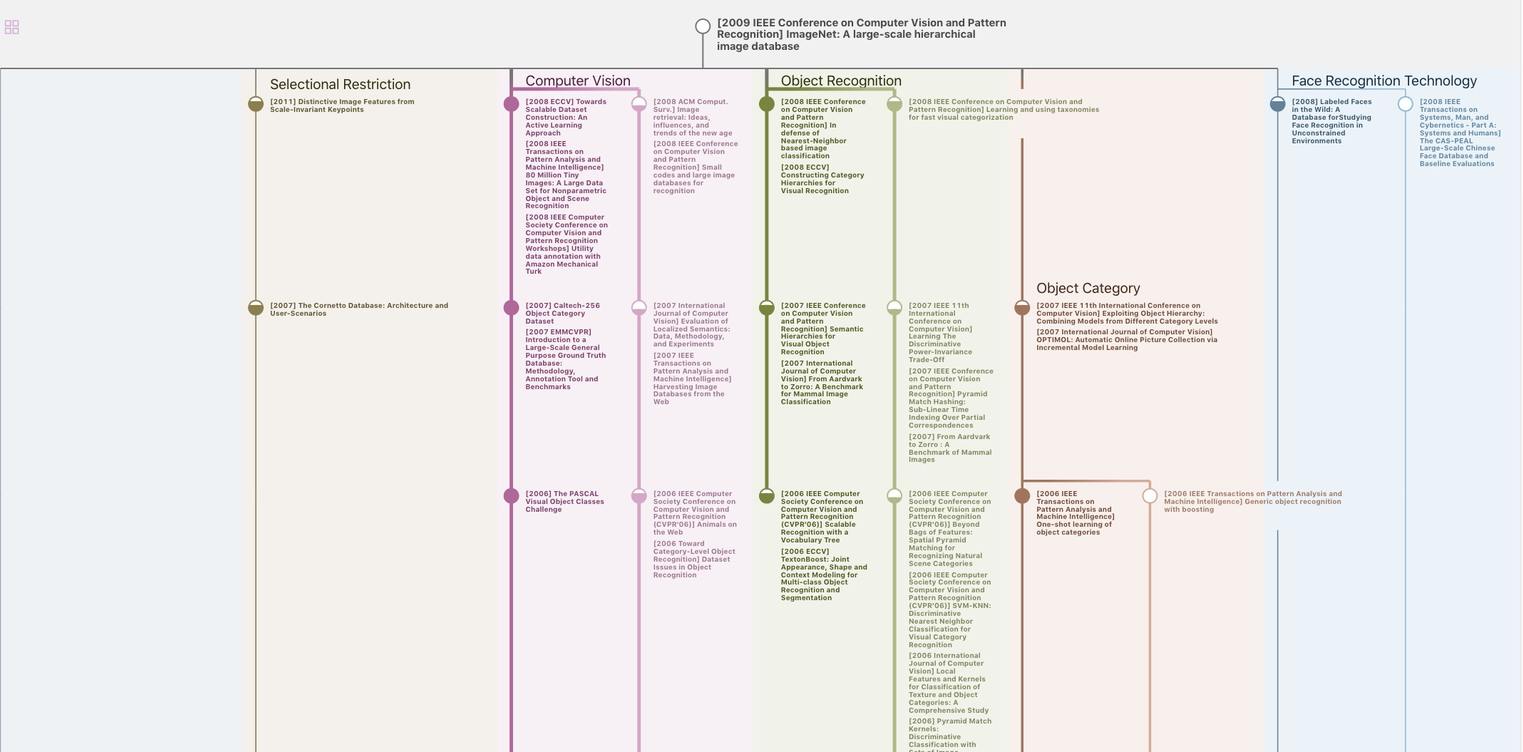
生成溯源树,研究论文发展脉络
Chat Paper
正在生成论文摘要