Holographic Factorization Machines for Recommendation
THIRTY-THIRD AAAI CONFERENCE ON ARTIFICIAL INTELLIGENCE / THIRTY-FIRST INNOVATIVE APPLICATIONS OF ARTIFICIAL INTELLIGENCE CONFERENCE / NINTH AAAI SYMPOSIUM ON EDUCATIONAL ADVANCES IN ARTIFICIAL INTELLIGENCE(2019)
摘要
Factorization Machines (FMs) are a class of popular algorithms that have been widely adopted for collaborative filtering and recommendation tasks. FMs are characterized by its usage of the inner product of factorized parameters to model pairwise feature interactions, making it highly expressive and powerful. This paper proposes Holographic Factorization Machines (HFM), a new novel method of enhancing the representation capability of FMs without increasing its parameter size. Our approach replaces the inner product in FMs with holographic reduced representations (HRRs), which are theoretically motivated by associative retrieval and compressed outer products. Empirically, we found that this leads to consistent improvements over vanilla FMs by up to 4% improvement in terms of mean squared error, with improvements larger at smaller parameterization. Additionally, we propose a neural adaptation of HFM which enhances its capability to handle nonlinear structures. We conduct extensive experiments on nine publicly available datasets for collaborative filtering with explicit feedback. HFM achieves state-of-the-art performance on all nine, outperforming strong competitors such as Attentional Factorization Machines (AFM) and Neural Matrix Factorization (NeuMF).
更多查看译文
AI 理解论文
溯源树
样例
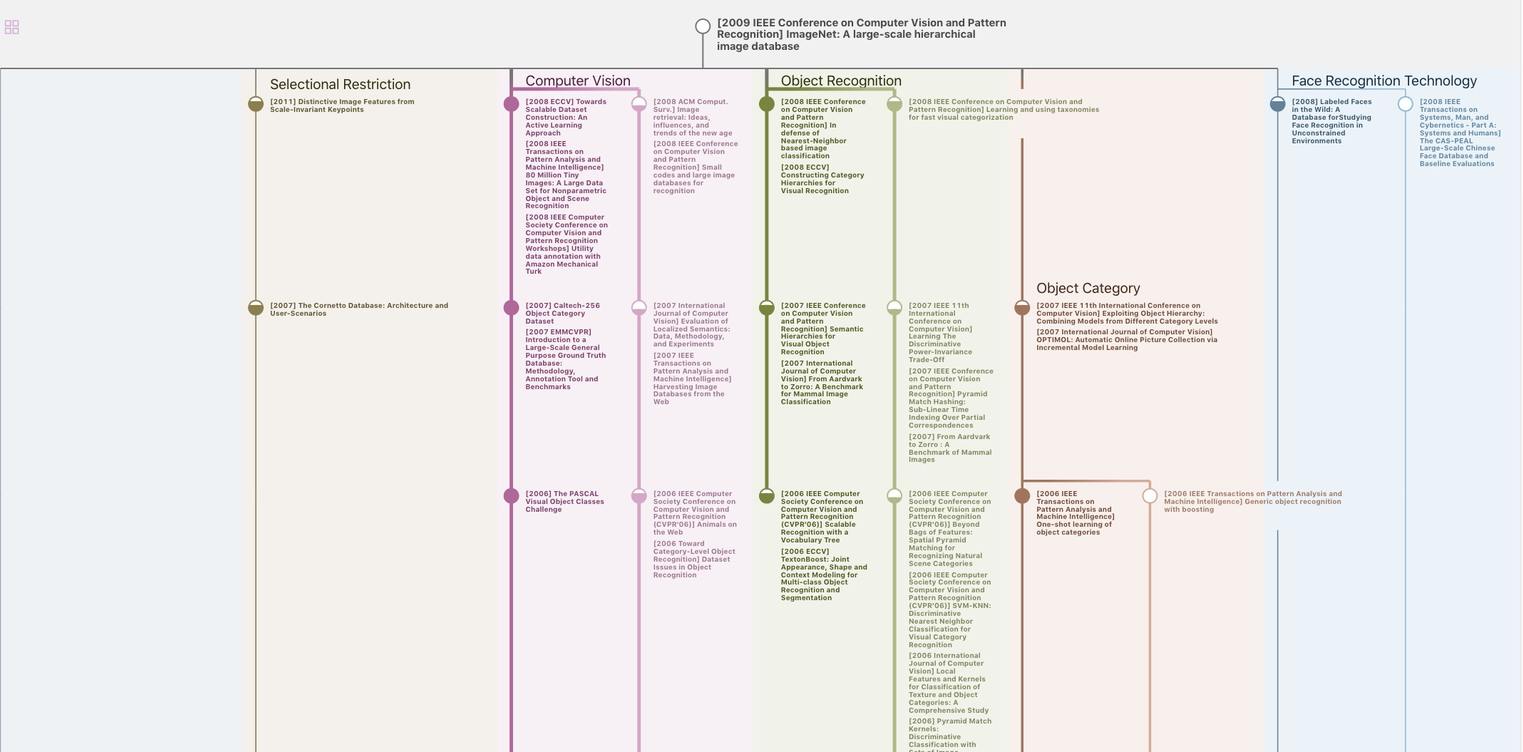
生成溯源树,研究论文发展脉络
Chat Paper
正在生成论文摘要