Multi-Fidelity Automatic Hyper-Parameter Tuning via Transfer Series Expansion
THIRTY-THIRD AAAI CONFERENCE ON ARTIFICIAL INTELLIGENCE / THIRTY-FIRST INNOVATIVE APPLICATIONS OF ARTIFICIAL INTELLIGENCE CONFERENCE / NINTH AAAI SYMPOSIUM ON EDUCATIONAL ADVANCES IN ARTIFICIAL INTELLIGENCE(2019)
摘要
Automatic machine learning (AutoML) aims at automatically choosing the best configuration for machine learning tasks. However, a configuration evaluation can be very time consuming particularly on learning tasks with large datasets. This limitation usually restrains derivative-free optimization from releasing its full power for a fine configuration search using many evaluations. To alleviate this limitation, in this paper, we propose a derivative-free optimization framework for AutoML using multi-fidelity evaluations. It uses many low-fidelity evaluations on small data subsets and very few high-fidelity evaluations on the full dataset. However, the low-fidelity evaluations can be badly biased, and need to be corrected with only a very low cost. We thus propose the Transfer Series Expansion (TSE) that learns the low-fidelity correction predictor efficiently by linearly combining a set of base predictors. The base predictors can be obtained cheaply from down-scaled and experienced tasks. Experimental results on real-world AutoML problems verify that the proposed framework can accelerate derivative-free configuration search significantly by making use of the multi-fidelity evaluations.
更多查看译文
AI 理解论文
溯源树
样例
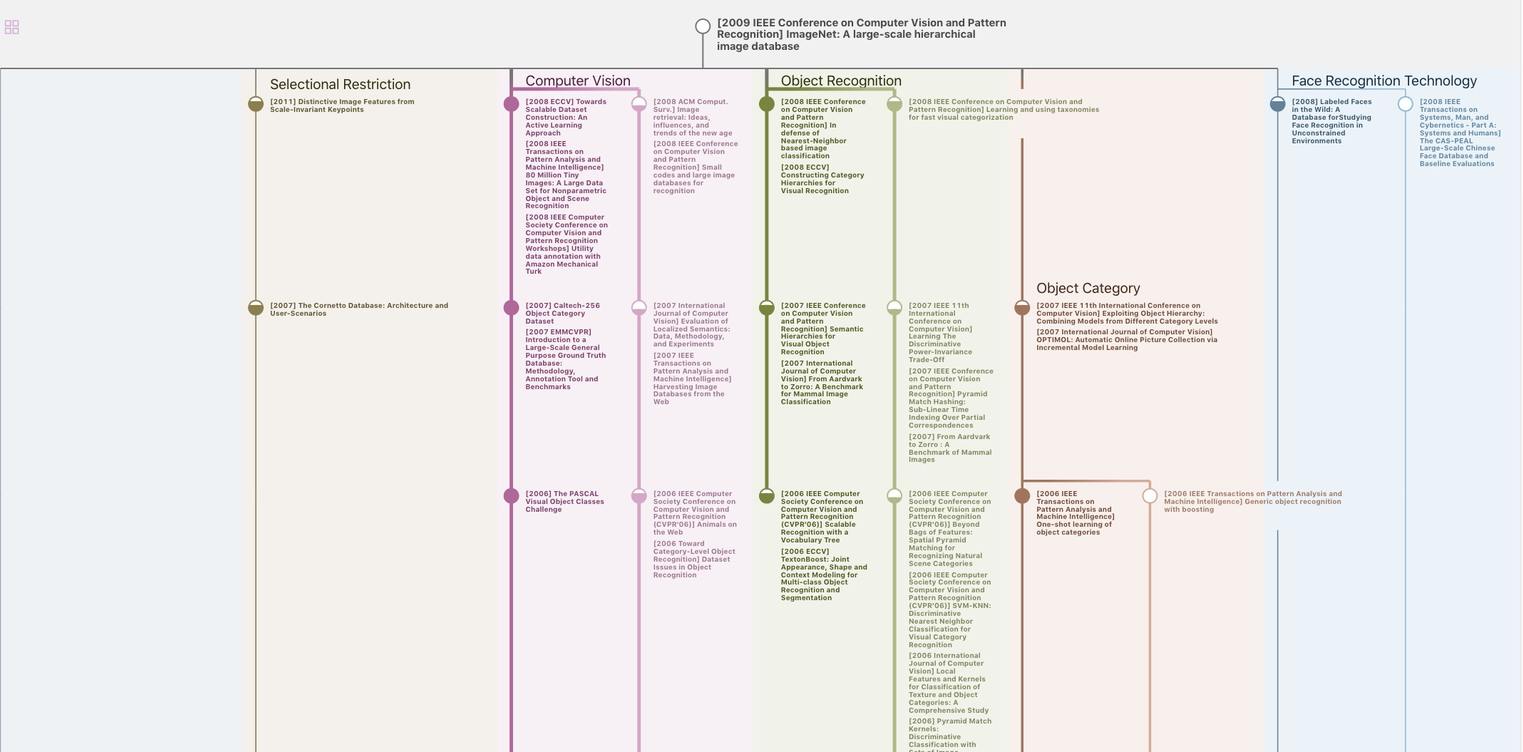
生成溯源树,研究论文发展脉络
Chat Paper
正在生成论文摘要