Factor Models for Portfolio Selection in Large Dimensions: The Good, the Better and the Ugly
JOURNAL OF FINANCIAL ECONOMETRICS(2021)
摘要
This paper injects factor structure into the estimation of time-varying, large-dimensional covariance matrices of stock returns. Existing factor models struggle to model the covariance matrix of residuals in the presence of time-varying conditional heteroskedasticity in large universes. Conversely, rotation-equivariant estimators of large-dimensional time-varying covariance matrices forsake directional information embedded in market-wide risk factors. We introduce a new covariance matrix estimator that blends factor structure with time-varying conditional heteroskedasticity of residuals in large dimensions up to 1000 stocks. It displays superior all-around performance on historical data against a variety of state-of-the-art competitors, including static factor models, exogenous factor models, sparsity-based models, and structure-free dynamic models. This new estimator can be used to deliver more efficient portfolio selection and detection of anomalies in the cross-section of stock returns.
更多查看译文
关键词
dynamic conditional correlations, factor models, multivariate GARCH, Markowitz portfolio selection, nonlinear shrinkage
AI 理解论文
溯源树
样例
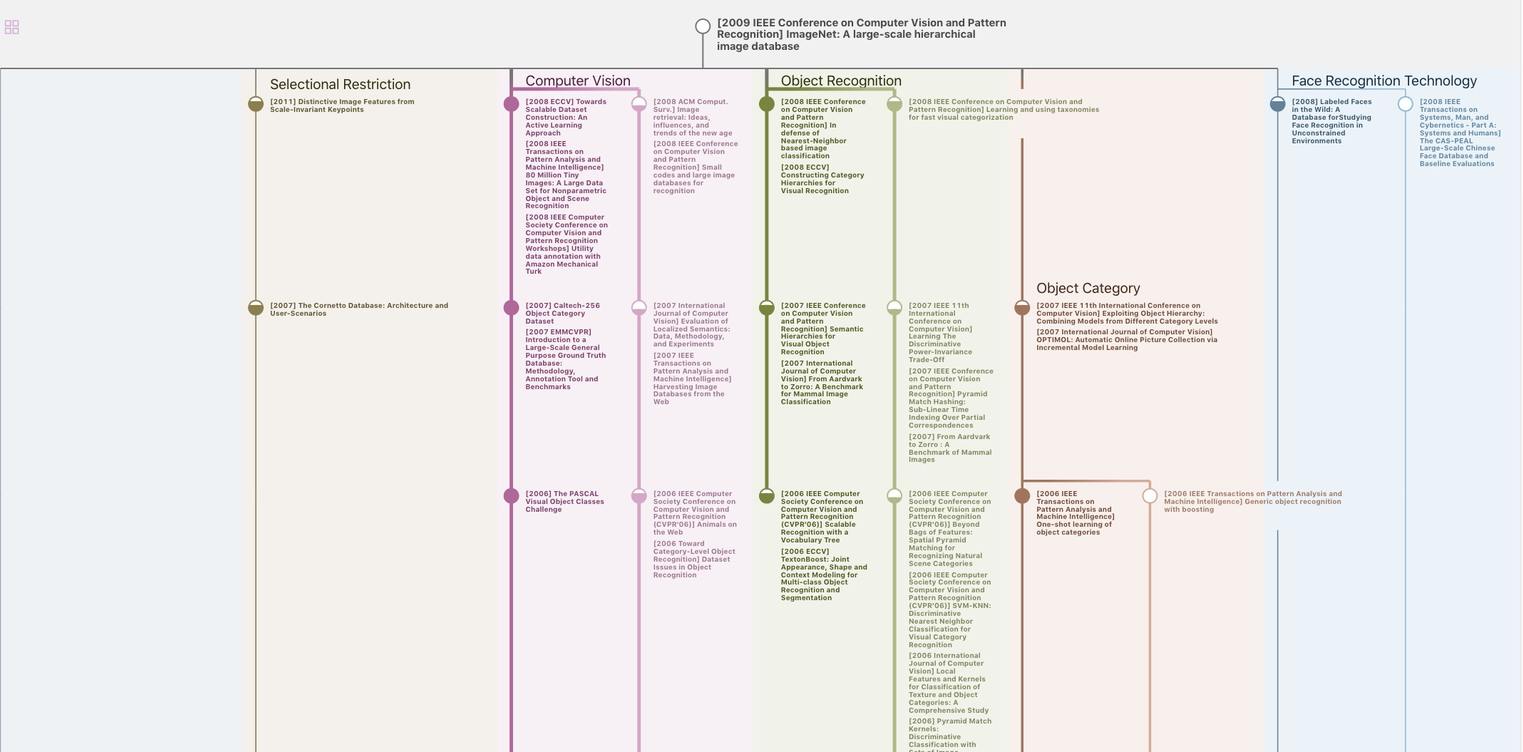
生成溯源树,研究论文发展脉络
Chat Paper
正在生成论文摘要