Landslide Susceptibility Modeling Based on GIS and Novel Bagging-Based Kernel Logistic Regression
APPLIED SCIENCES-BASEL(2018)
摘要
Landslides cause a considerable amount of damage around the world every year. Landslide susceptibility assessments are useful for the mitigation of the associated potential risks to local economic development, land use planning, and decision makers. The main aim of this study was to present a novel hybrid approach of bagging (B)-based kernel logistic regression (KLR), named the BKLR model, for spatial prediction of landslides in the Shangnan County, China. We first selected 15 conditioning factors for landslide susceptibility modeling. Then, the prediction capability of all conditioning factors was evaluated using the least square support vector machine method. Model validation and comparison were performed based on the area under the receiver operating characteristic curve and several statistical-based indexes, including positive predictive rate, negative predictive rate, sensitivity, specificity, kappa index, and root mean square error. Results indicated that the BKLR ensemble model outperformed and outclassed the KLR and the benchmark support vector machine model. Our findings overall confirmed that a combination of the meta model with a decision tree classifier based on a functional algorithm can decrease the over-fitting and variance problems of data, which could enhance the prediction power of the landslide model. The resultant susceptibility maps could be useful for hazard mitigation in the study area and other similar landslide-prone areas.
更多查看译文
关键词
landslide,meta classifier,prediction power,China
AI 理解论文
溯源树
样例
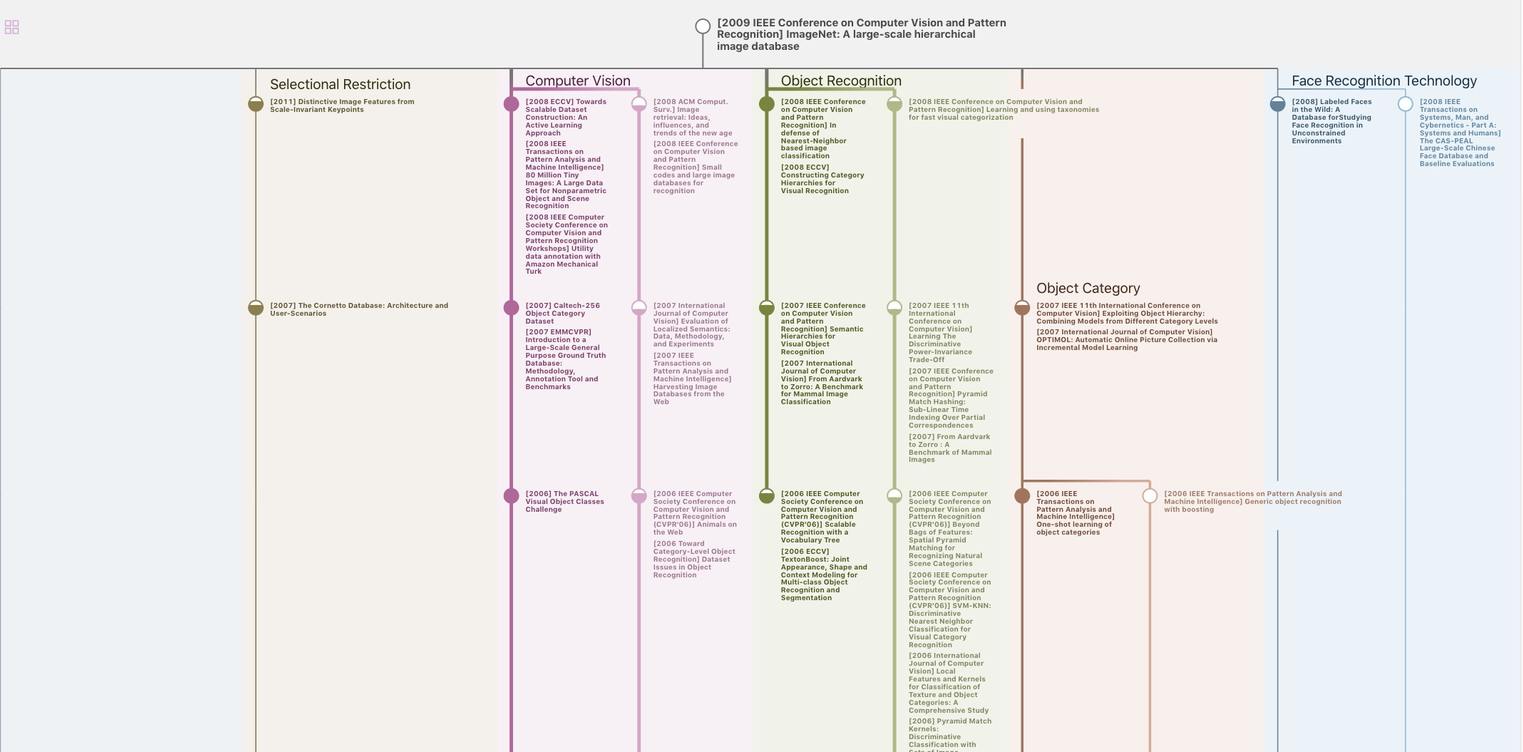
生成溯源树,研究论文发展脉络
Chat Paper
正在生成论文摘要