A Data-Driven Fuel Consumption Estimation Model for Airspace Redesign Analysis
2018 IEEE/AIAA 37th Digital Avionics Systems Conference (DASC)(2018)
摘要
A novel data-driven model for fast assessment of terminal airspace redesigns regarding system-level fuel burn is proposed in this paper. When given a terminal airspace design, the fuel consumption model calculates the fleet-wide fuel burn based on the departure/arrival profiles as specified in the design. Then, different airspace designs can be compared and optimized regarding their impact on fuel burn. The fuel consumption model is developed based on the Multilayer Perceptron Neural Network (MLPNN). The model is trained and evaluated using Digital Flight Data Recorder (FDR) data from real operations. We demonstrate the proposed MLPNN method via a case study of Hong Kong airspace and compare its performance with two other regression methods, the robust linear regression (the least median of squares, LMS) method and the r-Insensitive support vector regression (SVR) method. Cross-validation results indicate that the MLPNN performs better than the other two regression methods, with a prediction accuracy of 96.02% on average. Finally, we use the proposed model to estimate the potential fuel burn savings on three standard arrival procedures in Hong Kong airspace. The results show that the proposed model is an effective tool to support fast evaluation of airspace designs focusing on fuel burn.
更多查看译文
关键词
fuel consumption,airspace design,Flight Data Recorder,Multilayer Perceptron Neural Networks,data-driven approach,Hong Kong airspace
AI 理解论文
溯源树
样例
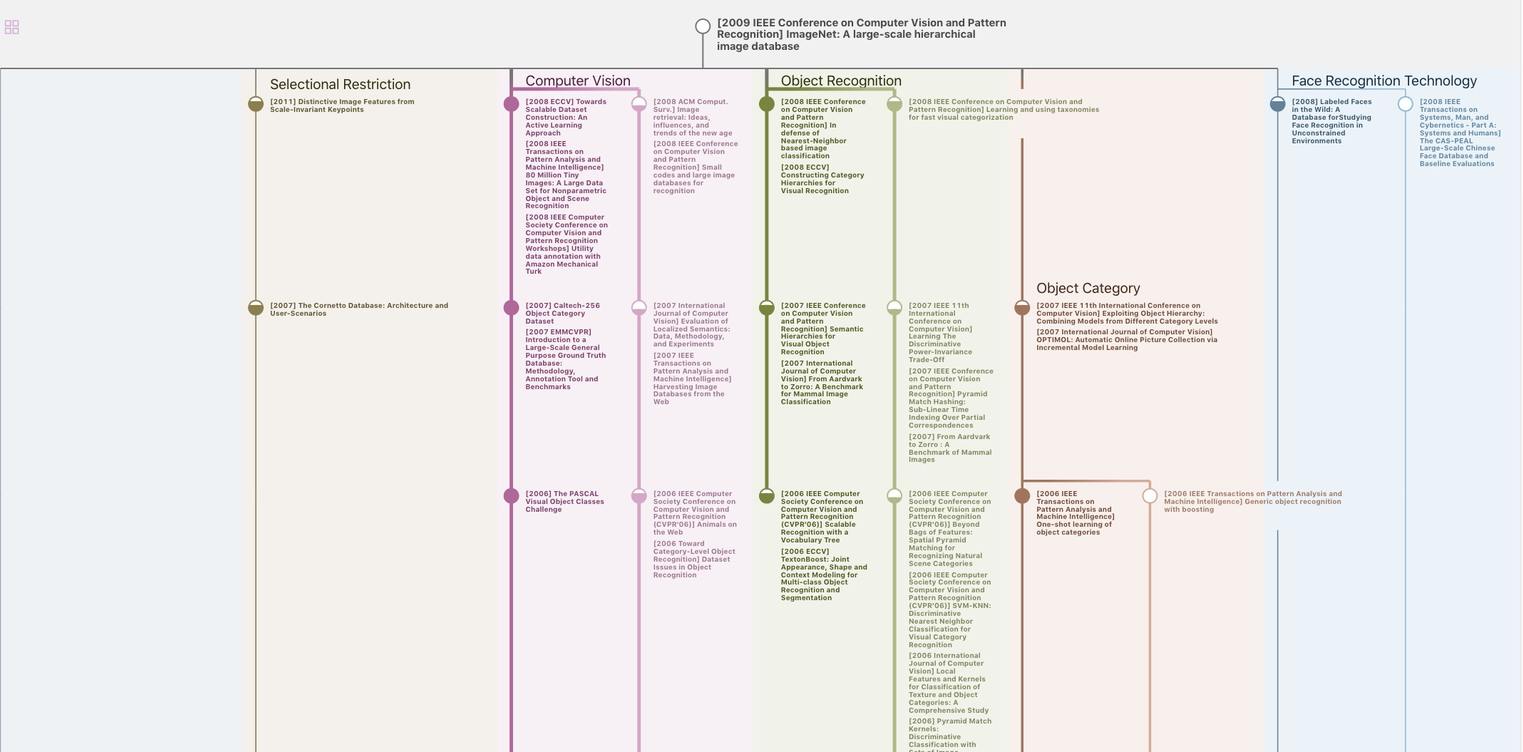
生成溯源树,研究论文发展脉络
Chat Paper
正在生成论文摘要