Postevent Reconnaissance Image Documentation Using Automated Classification
JOURNAL OF PERFORMANCE OF CONSTRUCTED FACILITIES(2019)
摘要
Reconnaissance teams are charged with collecting perishable data after a natural disaster. In the field, these engineers typically record their observations through images. Each team takes many views of both exterior and interior buildings and frequently collects associated metadata that reflect information represented in images, such as global positioning system (GPS) devices, structural drawings, timestamp, and measurements. Large quantities of images with a wide variety of contents are collected. The window of opportunity is short, and engineers need to provide accurate and rich descriptions of such images before the details are forgotten. In this paper, an automated approach is developed to organize and document such scientific information in an efficient and rapid manner. Deep convolutional neural network algorithms were successfully implemented to extract robust features of key visual contents in the images. A schema is designed based on the realistic needs of field teams examining buildings. A significant number of images collected from past earthquakes were used to train robust classifiers to automatically classify the images. The classifiers and associated schema were used to automatically generate individual reports for buildings.
更多查看译文
关键词
Natural disasters,Automation,Imaging techniques,Global navigation satellite systems,Team building,Building design,Data collection,Structural systems
AI 理解论文
溯源树
样例
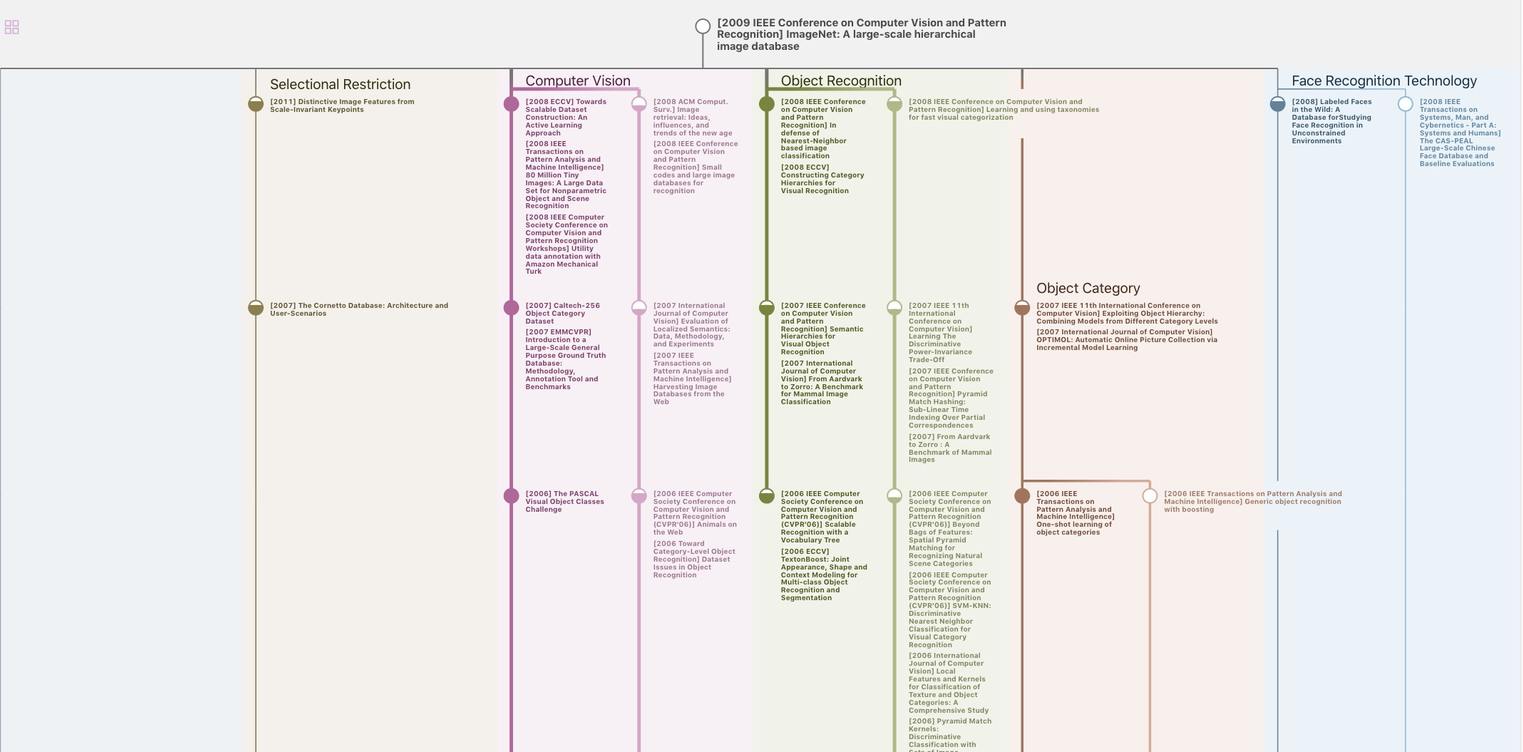
生成溯源树,研究论文发展脉络
Chat Paper
正在生成论文摘要