Designing neural network based decoders for surface codes
arXiv: Quantum Physics(2018)
摘要
Recent works have shown that small distance quantum error correction codes can be efficiently decoded by employing machine learning techniques like neural networks. Various techniques employing neural networks have been used to increase the decoding performance, however, there is no framework that analyses a step-by-step procedure in designing such a neural network based decoder. The main objective of this work is to present a detailed framework that investigates the way that various neural network parameters affect the decoding performance, the training time and the execution time of a neural network based decoder. We focus on neural network parameters such as the size of the training dataset, the structure, and the type of the neural network, the batch size and the learning rate used during training. We compare various decoding strategies and showcase how these influence the objectives for different error models. For the noiseless syndrome measurements, we reach up to 50% improvement against the benchmark algorithm (Blossom) and for the noisy syndrome measurements, we show efficient decoding performance up to 97 qubits for the rotated Surface code. Furthermore, we show that the scaling of the execution time (time calculated during the inference phase) is linear with the number of qubits, which is a significant improvement compared to existing classical decoding algorithms that scale polynomially with the number of qubits.
更多查看译文
AI 理解论文
溯源树
样例
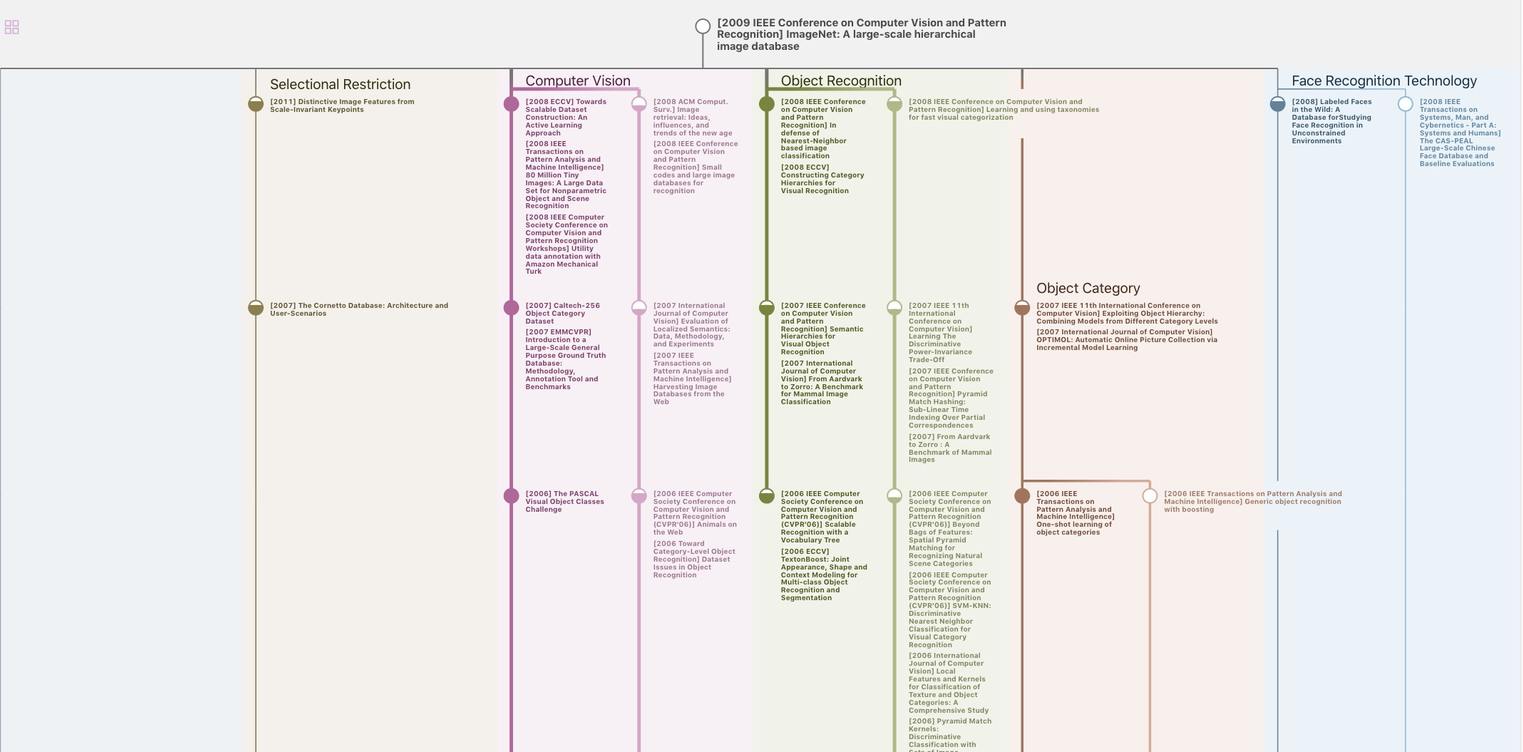
生成溯源树,研究论文发展脉络
Chat Paper
正在生成论文摘要