AB0322 Develop an master algorithm for drug withdraw strategy in reduction of adverse events with combination therapy- a machine learning model from the smart system of disease management (SSDM)
ANNALS OF THE RHEUMATIC DISEASES(2018)
摘要
Background Combination therapy with DMARDs for treating RA is considered as standard of care. However, certain rates of adverse events (AEs) are unavoidable. The stigma is which drug should be stopped first once AEs emerge. The decisions made by clinicians are always empirically. Objectives To develop an algorithm for decision making on drug withdraw sequence in face of adverse events with combination therapy based on data mining and machine learning from the SSDM. Methods SSDM is an interactive mobile disease management tool, including the doctors’ and patients’ application system (App). The patients can input medical records and perform self-evaluation via App. The data synchronises to the mobiles of authorised rheumatologists through cloud and advices could be delivered. In order to develop the master algorithm, abnormal white blood cell (WBC) counts in blood were first targeted. WBC and medication data was collected, extracted, validated, and then based on Bayesian networks, data mining, modelling, calculating, analysing were performed. WBC under 4,000/ml is defined as leukocytopenia (LP), and over 10,000/ml as infection predisposing (IP). Results From Jun 2014 to Jan 2018, 24,731 RA patients from 486 centres registered in SSDM. 6099 are male and 18 632 are female with mean age of 49.28±16.08 (18 to 99) years. 19 different drugs and 126 types of combination therapies are identified. Lab test results showed LP happened in 87 and IP in 123 treatment regiments. Among them we selected prednisone (Pred), leflunomide (LEF), methotrexate (MTX) and hydroxycholoqine (HCQ) as an example to develop a master algorithm based on Bayesian networks and learning model. Figure 1 shows Bayesian network and data processing, in which, quartet are correlating with 15 different regiments. Based on Bayesian method and network data, the calculation for LP and IP probabilities is generated through 32 modelling, and the algorithm for drug withdraw strategies are generated. Drug withdraw sequence for LP is HCQ, then LEF and then Pre, and the risks of LP are reduced by 64%, 52% and 26%, respectively. For IP, withdraw sequence is Pred, then MTX and then LEF, and the risks of IP are reduced by 57%, 63%, and 14%, respectively. Pred: prednisone; HCQ: hydroxycholoqine; MTX: methotrexate; LEF: leflunomide; LP: leukocytopenia; IP: infection predisposing Conclusions Big data system can be built using SSDM via empowering patient. Through data mining, networking, modelling, and Bayesian calculation, a master algorithm for drug withdraw strategy in reduction of adverse events with combination therapy is developed, which can be applied on the other AEs in SSDM and may replicated in other diseases. Following the continuing data inputs and machine leaning, an artificial intelligent system in assisting clinical decision making may be achieved. Limitations: This study only focus on rate of AE without considering the efficacy, without stratifying dosing. Disclosure of Interest None declared
更多查看译文
AI 理解论文
溯源树
样例
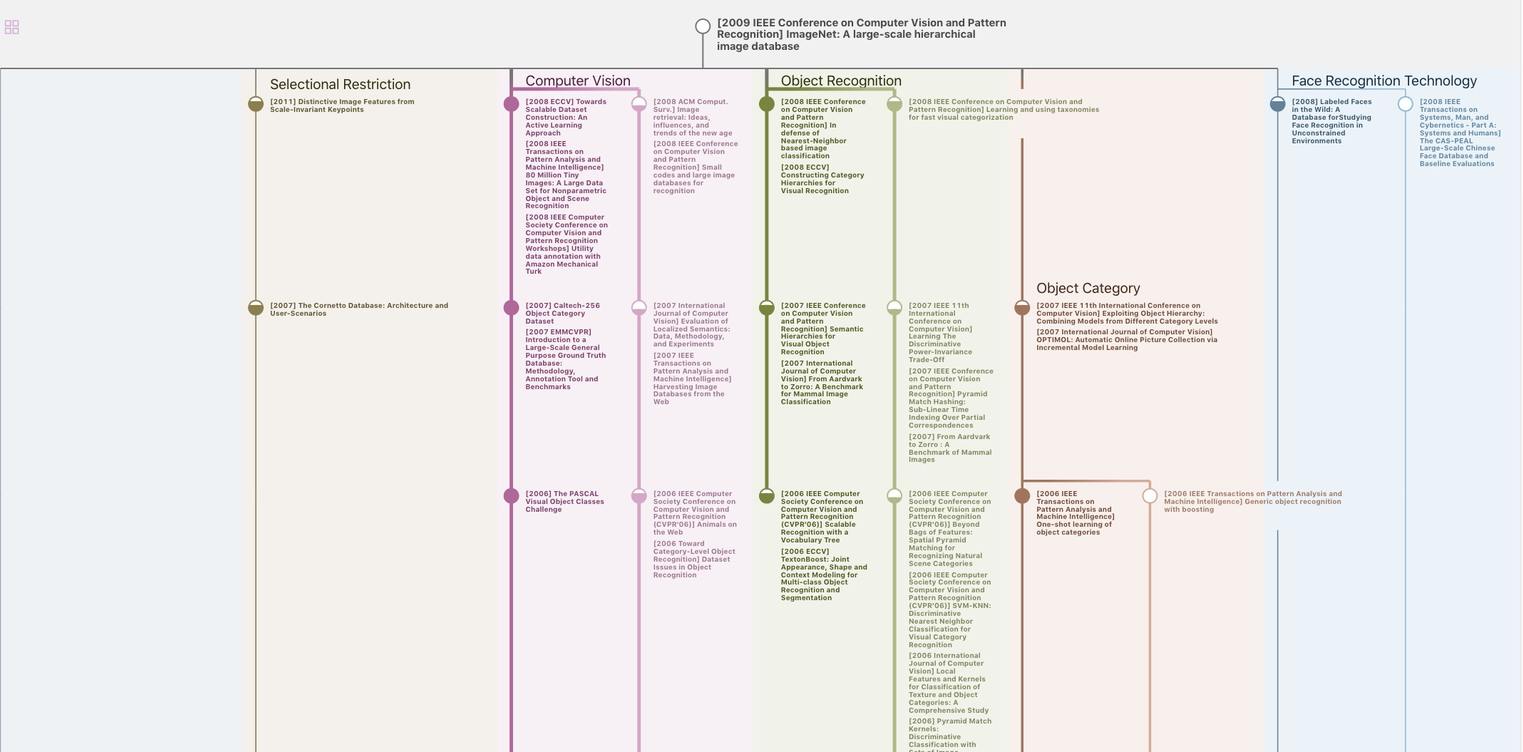
生成溯源树,研究论文发展脉络
Chat Paper
正在生成论文摘要