The ETS challenges: a machine learning approach to the evaluation of simulated financial time series for improving generation processes
arXiv: Computational Finance(2018)
摘要
This paper presents an evaluation framework that attempts to quantify the degree of realism of simulated financial time series, whatever the simulation method could be, with the aim of discover unknown characteristics that are not being properly reproduced by such methods in order to improve them. For that purpose, the evaluation framework is posed as a machine learning problem in which some given time series examples have to be classified as simulated or real financial time series. The challenge is proposed as an open competition, similar to those published at the Kaggle platform, in which participants must send their classification results along with a description of the features and the classifiers used. The results of these challenges have revealed some interesting properties of financial data, and have lead to substantial improvements in our simulation methods under research, some of which will be described in this work.
更多查看译文
AI 理解论文
溯源树
样例
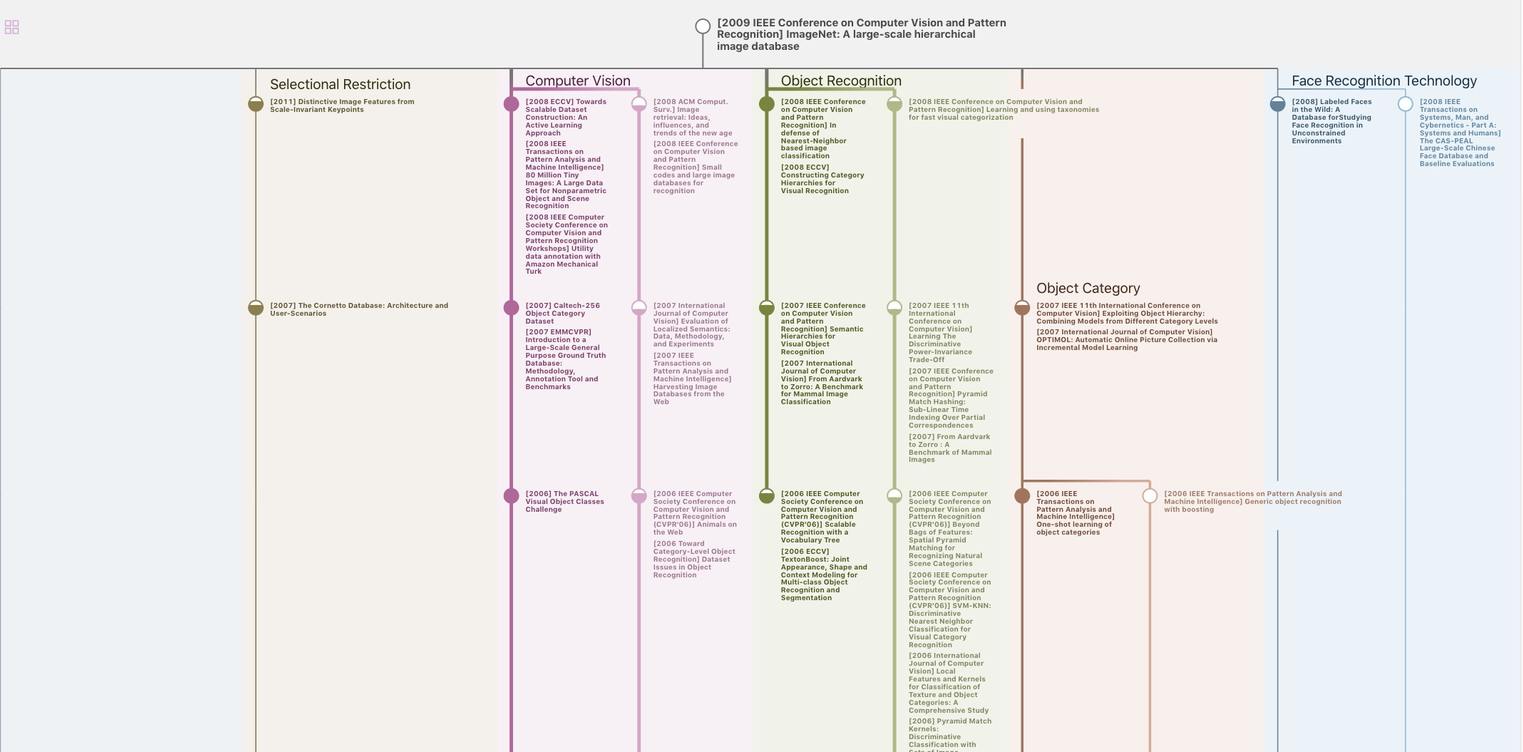
生成溯源树,研究论文发展脉络
Chat Paper
正在生成论文摘要