Label-Free Identification Of Lymphocyte Subtypes Using Three-Dimensional Quantitative Phase Imaging And Machine Learning
JOVE-JOURNAL OF VISUALIZED EXPERIMENTS(2018)
摘要
We describe here a protocol for the label-free identification of lymphocyte subtypes using quantitative phase imaging and machine learning. Identification of lymphocyte subtypes is important for the study of immunology as well as diagnosis and treatment of various diseases. Currently, standard methods for classifying lymphocyte types rely on labeling specific membrane proteins via antigen-antibody reactions. However, these labeling techniques carry the potential risks of altering cellular functions. The protocol described here overcomes these challenges by exploiting intrinsic optical contrasts measured by 3D quantitative phase imaging and a machine learning algorithm. Measurement of 3D refractive index (RI) tomograms of lymphocytes provides quantitative information about 3D morphology and phenotypes of individual cells. The biophysical parameters extracted from the measured 3D RI tomograms are then quantitatively analyzed with a machine learning algorithm, enabling labelfree identification of lymphocyte types at a single-cell level. We measure the 3D RI tomograms of B, CD4+ T, and CD8+ T lymphocytes and identified their cell types with over 80% accuracy. In this protocol, we describe the detailed steps for lymphocyte isolation, 3D quantitative phase imaging, and machine learning for identifying lymphocyte types.
更多查看译文
关键词
Immunology and Infection,Issue 141,Quantitative phase imaging,optical diffraction tomography,holotomography,holographic microscopy,lymphocyte identification,immune cell,immunology,machine learning,label-free imaging
AI 理解论文
溯源树
样例
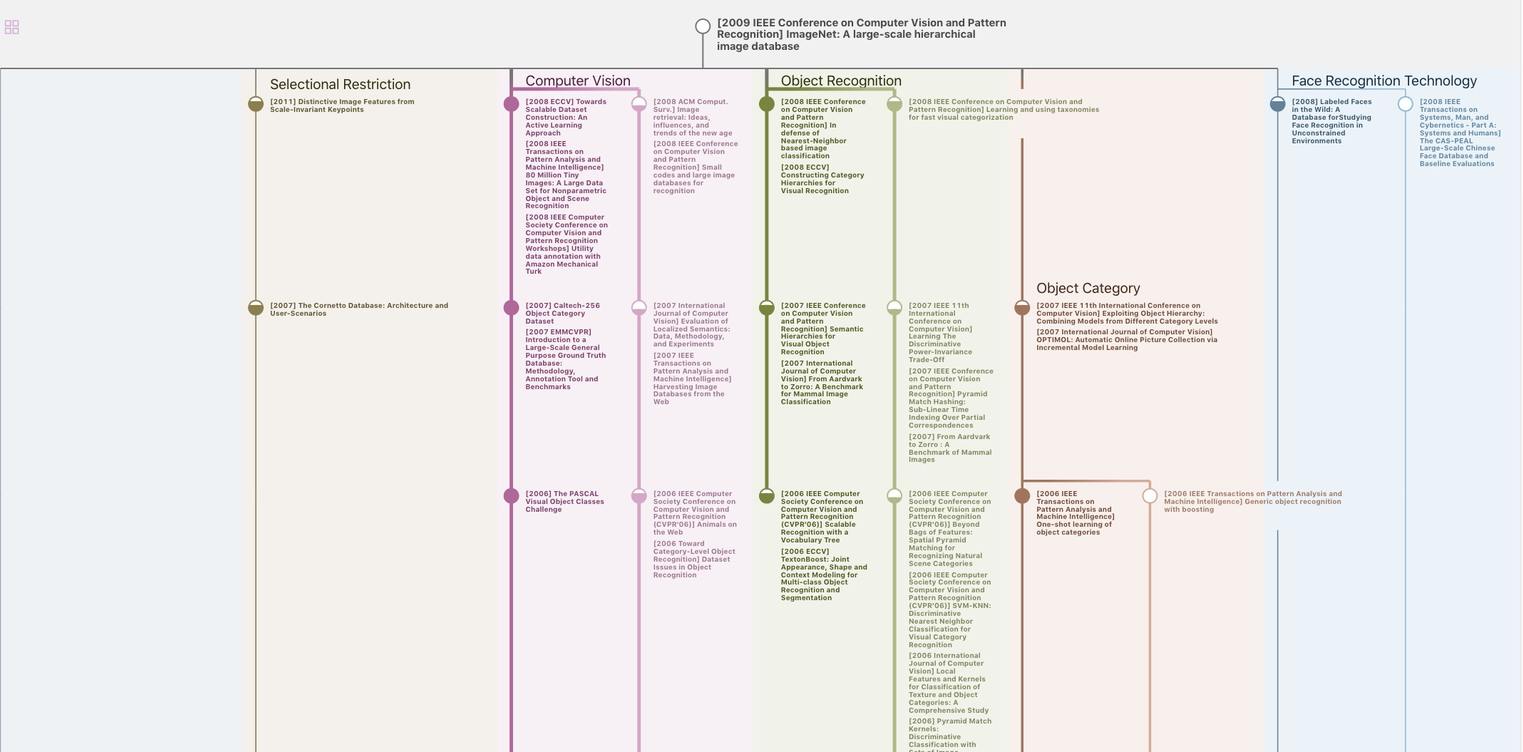
生成溯源树,研究论文发展脉络
Chat Paper
正在生成论文摘要