Data-driven diagnosis for compressed sensing with cross validation
PHYSICAL REVIEW E(2018)
摘要
We propose a data-driven procedure for diagnosing the results of compressed sensing as success or failure. Compressed sensing is an efficient data-acquisition method widely used in experimental physics. Many previous studies demonstrated that compressed sensing works well if sparse modeling can be applied; that is, physical phenomena of interest are assumed to be described by only a few explanatory factors. However, it is difficult to confirm the assumption of sparse modeling for respective instances in advance because we do not know the true representation or its sparseness of the instance being investigated. To overcome this difficulty, we examined a statistical tool called cross validation, in which all available data are randomly divided into two subsets and the accuracy of describing data in one subset by a sparse representation estimated from only data in the other subset is evaluated as an error. In particular, we focused on the dependence of the cross-validation error on the size ratio of the two subsets. Our analysis, inspired by statistical mechanics, showed that the cross-validation error asymptotically follows a power law of the size ratio when the total amount of available data is exactly at the critical point between success and failure. Hence, compressed-sensing results can be diagnosed in a data-driven manner by comparing the behavior of the cross-validation error with the power law.
更多查看译文
AI 理解论文
溯源树
样例
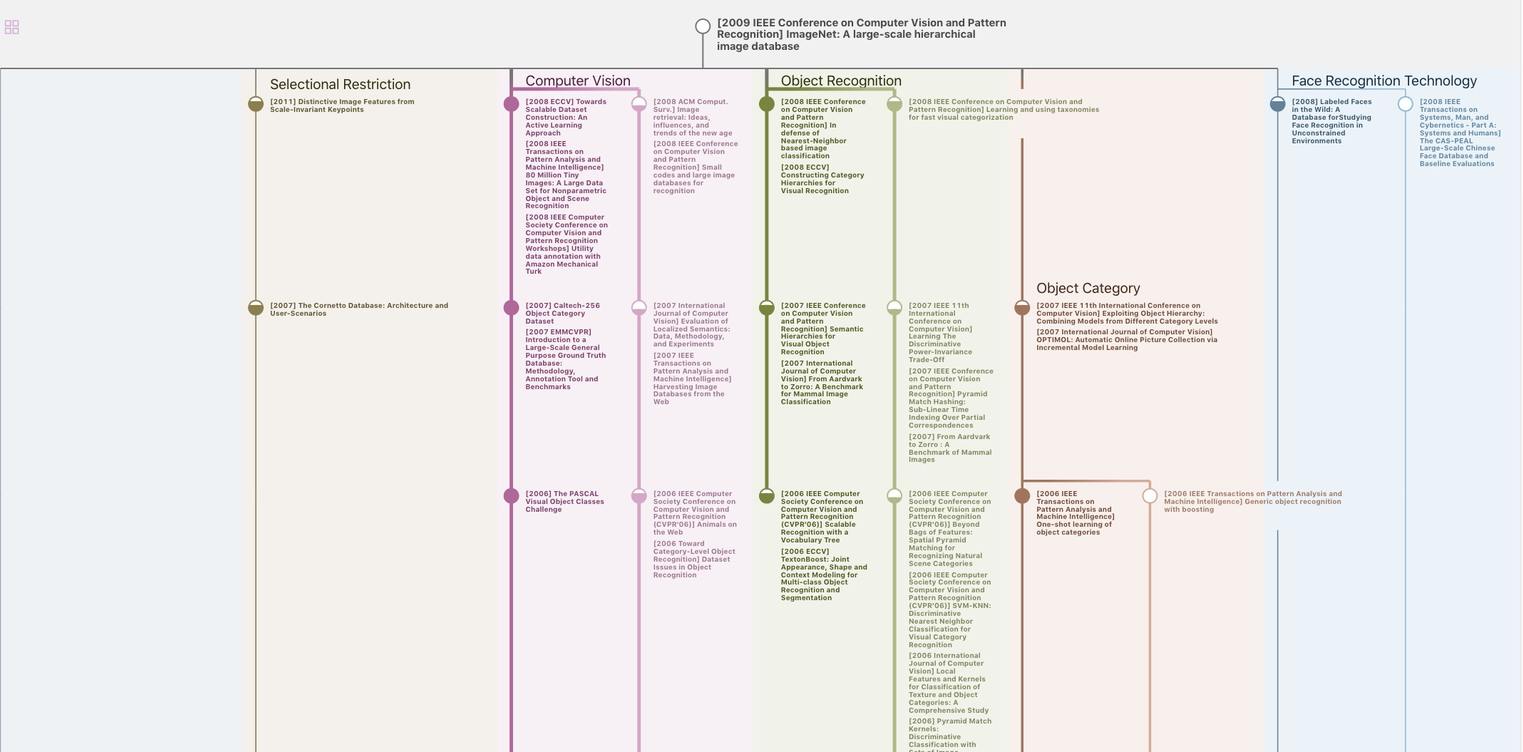
生成溯源树,研究论文发展脉络
Chat Paper
正在生成论文摘要