An ODE method to prove the geometric convergence of adaptive stochastic algorithms
Stochastic Processes and their Applications(2022)
摘要
We consider stochastic algorithms derived from methods for solving deterministic optimization problems, especially comparison-based algorithms derived from stochastic approximation algorithms with a constant step-size. We develop a methodology for proving geometric convergence of the parameter sequence {θn}n⩾0 of such algorithms. We employ the ordinary differential equation (ODE) method, which relates a stochastic algorithm to its mean ODE, along with a Lyapunov-like function Ψ such that the geometric convergence of Ψ(θn) implies – in the case of an optimization algorithm – the geometric convergence of the expected distance between the optimum and the search point generated by the algorithm. We provide two sufficient conditions for Ψ(θn) to decrease at a geometric rate: Ψ should decrease “exponentially” along the solution to the mean ODE, and the deviation between the stochastic algorithm and the ODE solution (measured by Ψ) should be bounded by Ψ(θn) times a constant. We also provide practical conditions under which the two sufficient conditions may be verified easily without knowing the solution of the mean ODE. Our results are any-time bounds on Ψ(θn), so we can deduce not only the asymptotic upper bound on the convergence rate, but also the first hitting time of the algorithm. The main results are applied to a comparison-based stochastic algorithm with a constant step-size for optimization on continuous domains.
更多查看译文
关键词
Adaptive stochastic algorithm,Comparison-based algorithm,Geometric convergence,Ordinary differential equation method,Lyapunov stability,Optimization
AI 理解论文
溯源树
样例
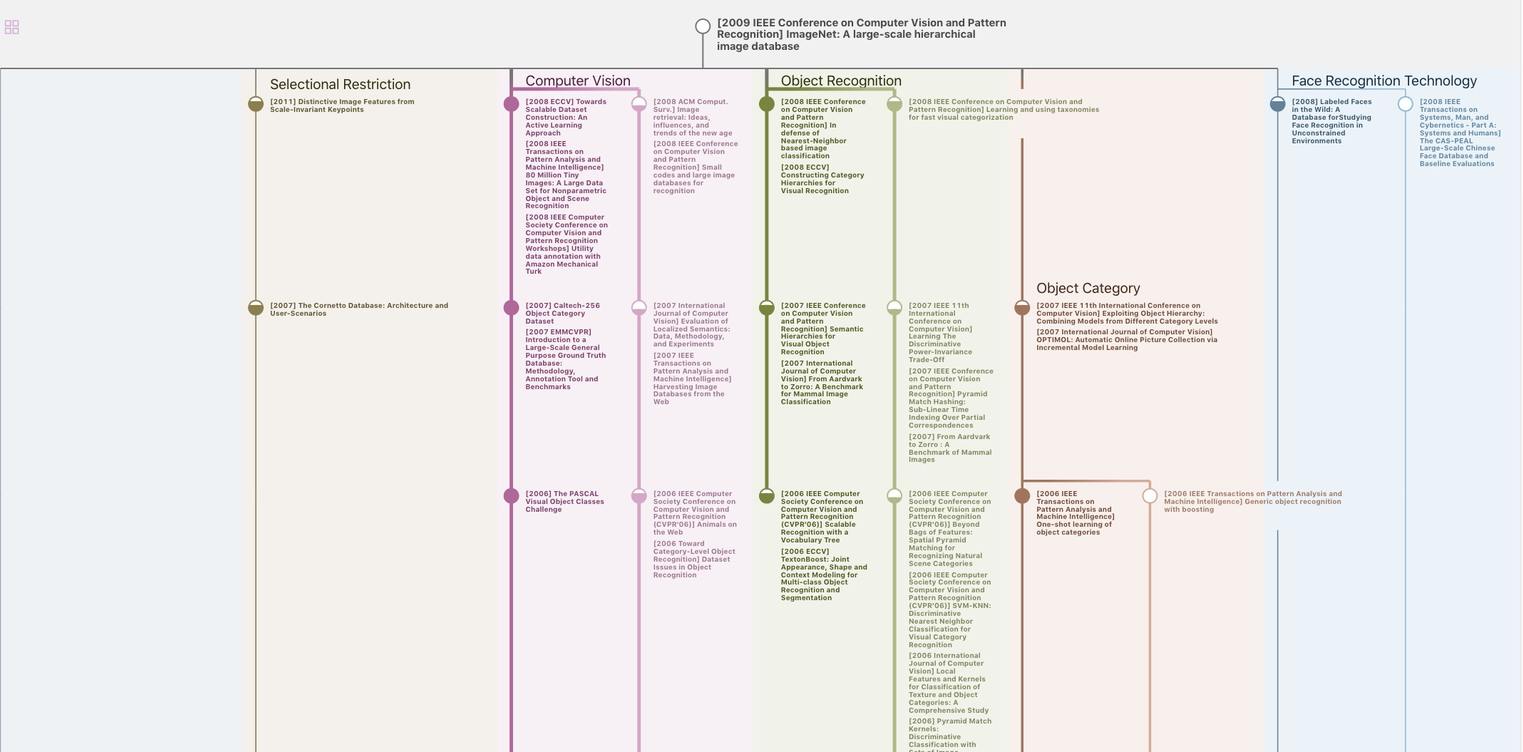
生成溯源树,研究论文发展脉络
Chat Paper
正在生成论文摘要