Impact of Dense and Frequent Surface Observations on 1-Minute-Update Severe Rainstorm Prediction: A Simulation Study
JOURNAL OF THE METEOROLOGICAL SOCIETY OF JAPAN(2019)
摘要
This study aims to investigate the potential impact of surface observations with a high spatial and temporal density on a local heavy rainstorm prediction. A series of Observing System Simulation Experiments (OSSEs) are performed using the Local Ensemble Transform Kalman Filter with the Japan Meteorological Agency non-hydrostatic model at 1-km resolution and with 1-minute update cycles. For the nature run of the OSSEs, a 100-m resolution simulation is performed for the heavy rainstorm case that caused five fatalities in Kobe, Japan on July 28, 2008. Synthetic radar observation data, both reflectivity and Doppler velocity, are generated at 1-km resolution every minute from the 100-m resolution nature run within a 60-km range, simulating the phased array weather radar (PAWR) at Osaka University. The control experiment assimilates only the radar data, and two sensitivity experiments are performed to investigate the impact of additional surface observations obtained every minute at 8 and 167 stations in Kobe. The results show that the dense and frequent surface observations have a significant positive impact on the analyses and forecasts of the local heavy rainstorm, although the number of assimilated observations is three orders of magnitude less than the PAWR data. Equivalent potential temperature and convergence at the low levels are improved, contributing to intensified convective cells and local heavy rainfalls.
更多查看译文
关键词
surface data assimilation,local ensemble transform Kalman filter,rainfall prediction
AI 理解论文
溯源树
样例
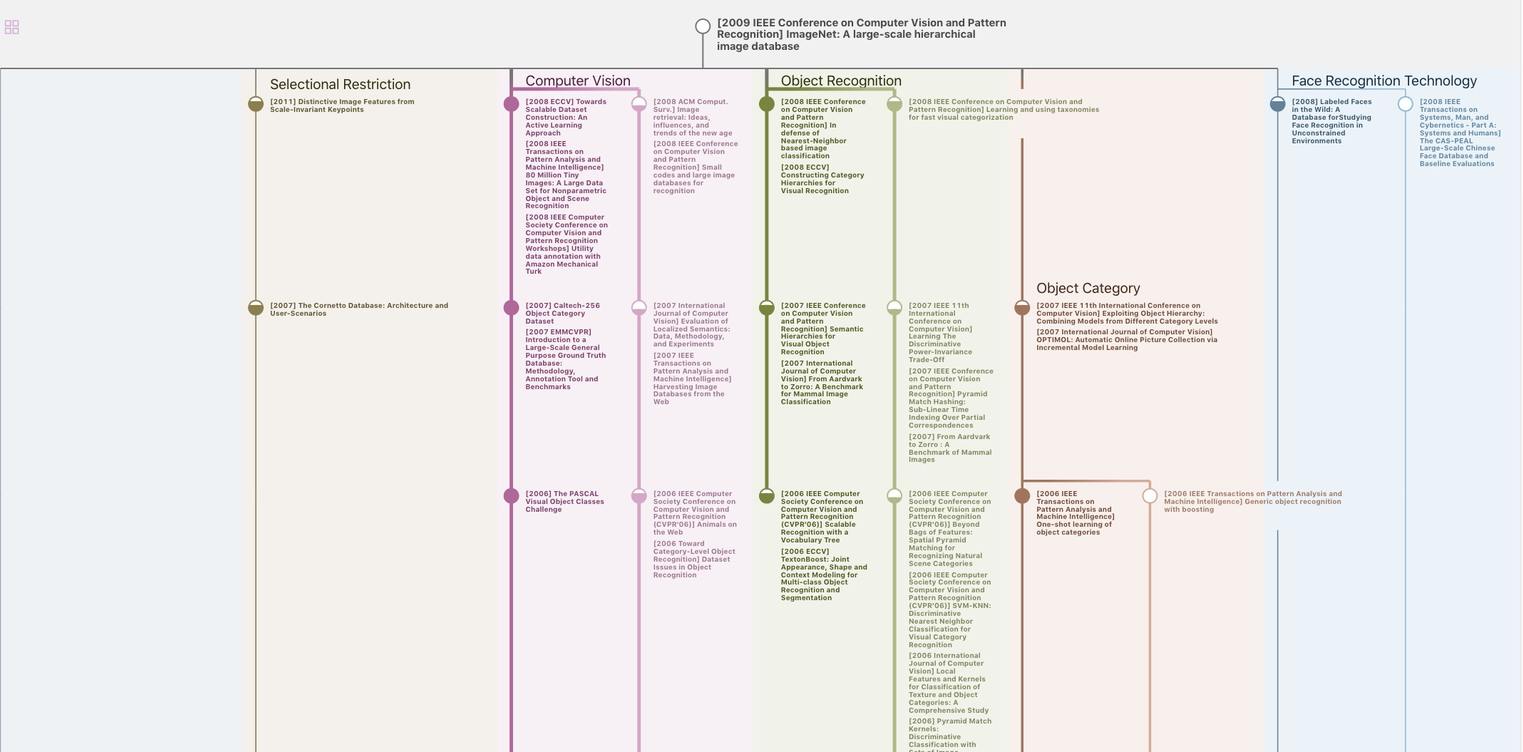
生成溯源树,研究论文发展脉络
Chat Paper
正在生成论文摘要