Image Classification Using Deep Autoencoders
2017 IEEE INTERNATIONAL CONFERENCE ON COMPUTATIONAL INTELLIGENCE AND COMPUTING RESEARCH (ICCIC)(2017)
摘要
Deep learning refers to computational models comprising of several processing layers that permit display of data with a compound level of abstraction. Till date, several deep learning architectures have been developed, and notable results are attained. The best result often involves an unsupervised pre-training phase followed by supervised learning task. In this work, a particular implementation of deep autoencoders with SVM (Support Vector Machine) layer as a classification layer on the top of the encoding layer is explored. A comparison is made on MNIST dataset with softmax regression function layer and SVM layer as a classification layer with 2 layers and 3 layers SAE (Stack Autoencoders) respectively. Experimental results are evaluated using SAE. It is observed that SVM as classification layer obtains 99.8% accuracy with 0.2% error rate and outperforms softmax regression layer as a classification layer in autoencoders. Further, affect of varying number of neurons in the hidden layers of the autoencoders on the network performance with regard to classification accuracy is also studied.
更多查看译文
关键词
Deep Learning,Stack Autoencoders,Support Vector Machine
AI 理解论文
溯源树
样例
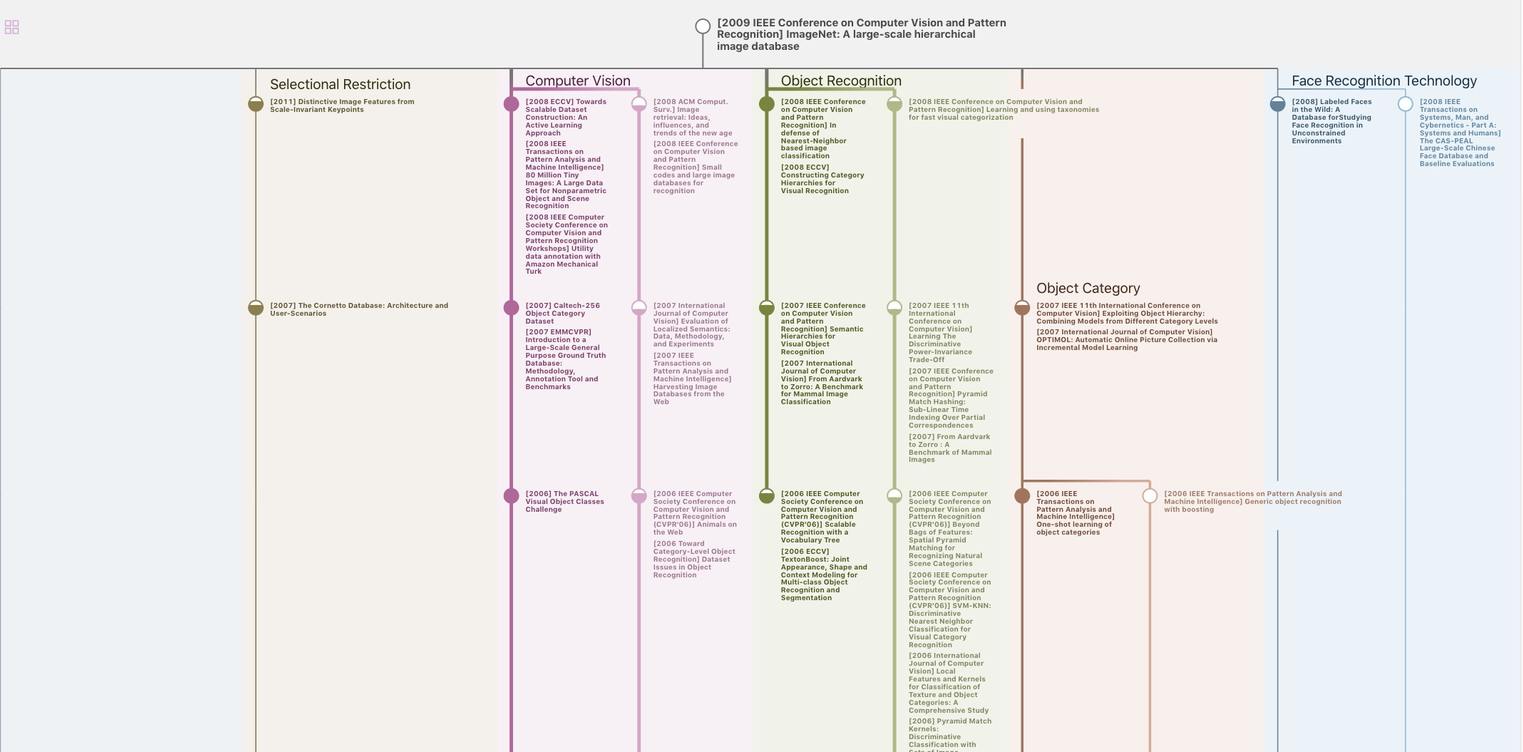
生成溯源树,研究论文发展脉络
Chat Paper
正在生成论文摘要