A Framework to Incorporate D-trace Loss into Compositional Data Analysis
bioRxiv(2018)
摘要
The development of high-throughput sequencing technologies for 16S rRNA geneprofiling provides higher quality compositional data for microbe communities. Inferringthe direct interaction network under a specific condition and understanding how thenetwork structure changes between two different environmental or genetic conditions aretwo important topics in biological studies. However, the compositional nature and highdimensionality of the data are challenging in the context of network and differentialnetwork recovery. To address this problem in the present paper, we proposed aframework to incorporate the data transformations developed for compositional dataanalysis into D-trace loss for network and differential network estimation, respectively.The sparse matrix estimators are defined as the minimizer of the corresponding lassopenalized loss. This framework is characterized by its straightforward application basedon the ADMM algorithm for numerical solution. Simulations show that the proposedmethod outperforms other state-of-the-art methods in network and differential networkinference under different scenarios. Finally, as an illustration, our method is applied toa mouse skin microbiome data.
更多查看译文
AI 理解论文
溯源树
样例
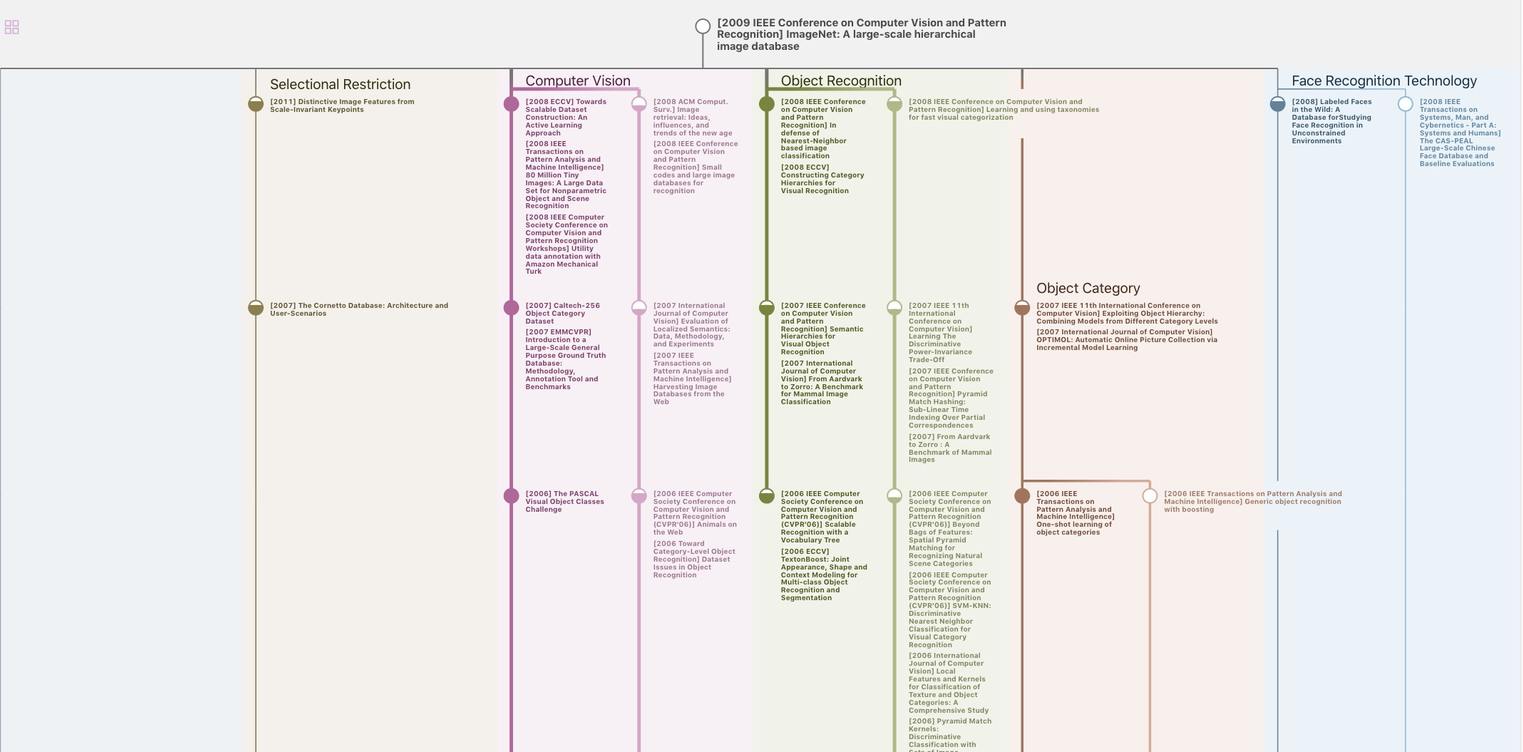
生成溯源树,研究论文发展脉络
Chat Paper
正在生成论文摘要