Directed Lovász local lemma and Shearer’s lemma
Annals of Mathematics and Artificial Intelligence(2019)
摘要
Moser and Tardos (J. ACM (JACM) 57 (2), 11 2010 ) gave an algorithmic proof of the lopsided Lovász local lemma (LLL) in the variable framework, where each of the undesirable events is assumed to depend on a subset of a collection of independent random variables. For the proof, they define a notion of a lopsided dependency between the events suitable for this framework. In this work, we strengthen this notion, defining a novel directed notion of dependency and prove the LLL for the corresponding graph. We show that this graph can be strictly sparser (thus the sufficient condition for the LLL weaker) compared with graphs that correspond to other extant lopsided versions of dependency. Thus, in a sense, we address the problem “find other simple local conditions for the constraints (in the variable framework) that advantageously translate to some abstract lopsided condition” posed by Szegedy ( 2013 ). We also give an example where our notion of dependency graph gives better results than the classical Shearer lemma. Finally, we prove Shearer’s lemma for the dependency graph we define. For the proofs, we perform a direct probabilistic analysis that yields an exponentially small upper bound for the probability of the algorithm that searches for the desired assignment to the variables not to return a correct answer within n steps. In contrast, the method of proof that became known as the entropic method, gives an estimate of only the expectation of the number of steps until the algorithm returns a correct answer, unless the probabilities are tinkered with.
更多查看译文
关键词
Lovász local lemma,Shearer’s lemma,Lopsidependency
AI 理解论文
溯源树
样例
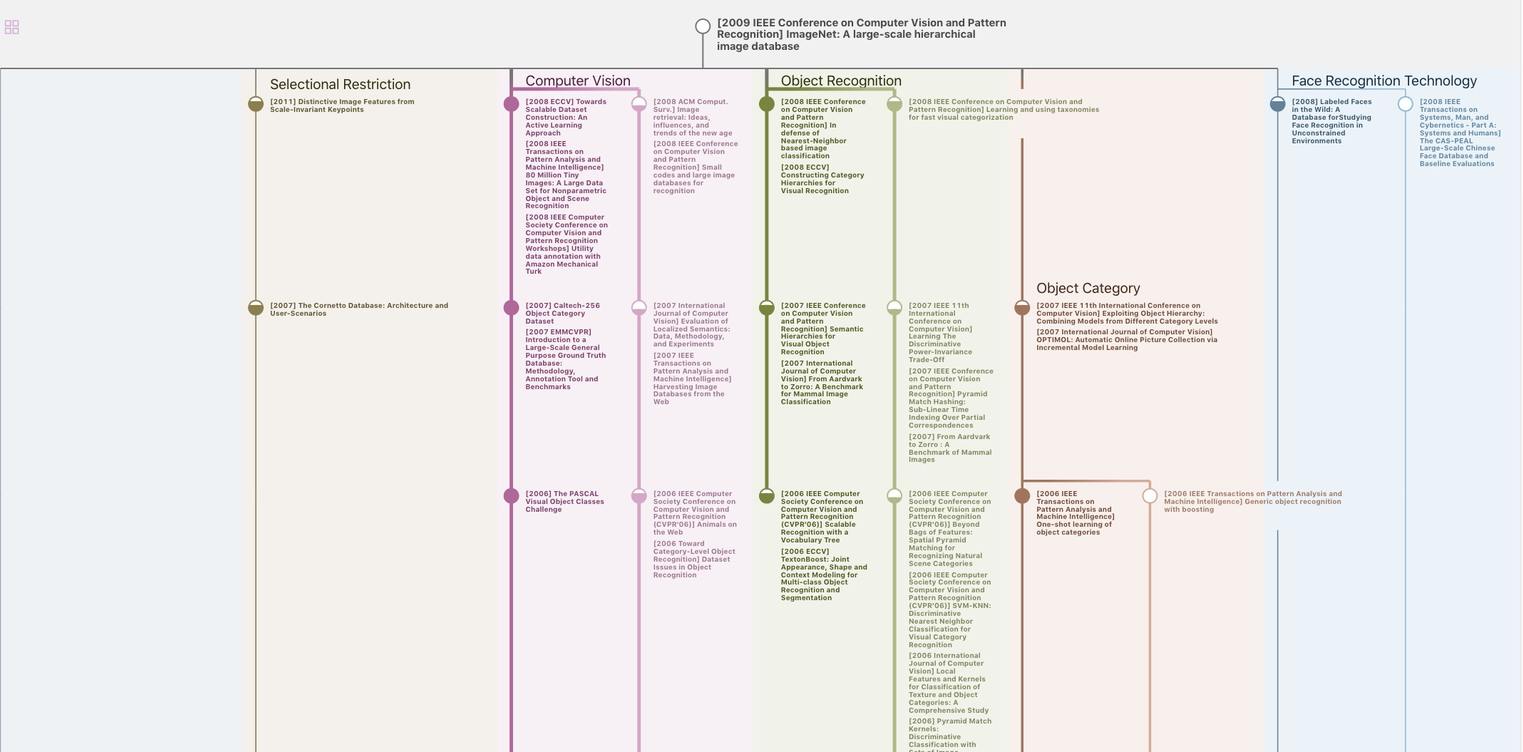
生成溯源树,研究论文发展脉络
Chat Paper
正在生成论文摘要