Hybridizing kernel‐based fuzzy c‐means with hierarchical selective neural network ensemble model for business failure prediction
JOURNAL OF FORECASTING(2019)
摘要
More and more ensemble models are used to forecast business failure. It is generally known that the performance of an ensemble relies heavily on the diversity between each base classifier. To achieve diversity, this study uses kernel-based fuzzy c-means (KFCM) to organize firm samples and designs a hierarchical selective ensemble model for business failure prediction (BFP). First, three KFCM methods-Gaussian KFCM (GFCM), polynomial KFCM (PFCM), and Hyper-tangent KFCM (HFCM)-are employed to partition the financial data set into three data sets. A neural network (NN) is then adopted as a basis classifier for BFP, and three sets, which are derived from three KFCM methods, are used to build three classifier pools. Next, classifiers are fused by the two-layer hierarchical selective ensemble method. In the first layer, classifiers are ranked based on their prediction accuracy. The stepwise forward selection method is employed to selectively integrate classifiers according to their accuracy. In the second layer, three selective ensembles in the first layer are integrated again to acquire the final verdict. This study employs financial data from Chinese listed companies to conduct empirical research, and makes a comparative analysis with other ensemble models and all its component models. It is the conclusion that the two-layer hierarchical selective ensemble is good at forecasting business failure.
更多查看译文
关键词
business failure prediction,financial data analysis,kernel-based fuzzy c-means,neural network,two-layer hierarchical selective ensemble
AI 理解论文
溯源树
样例
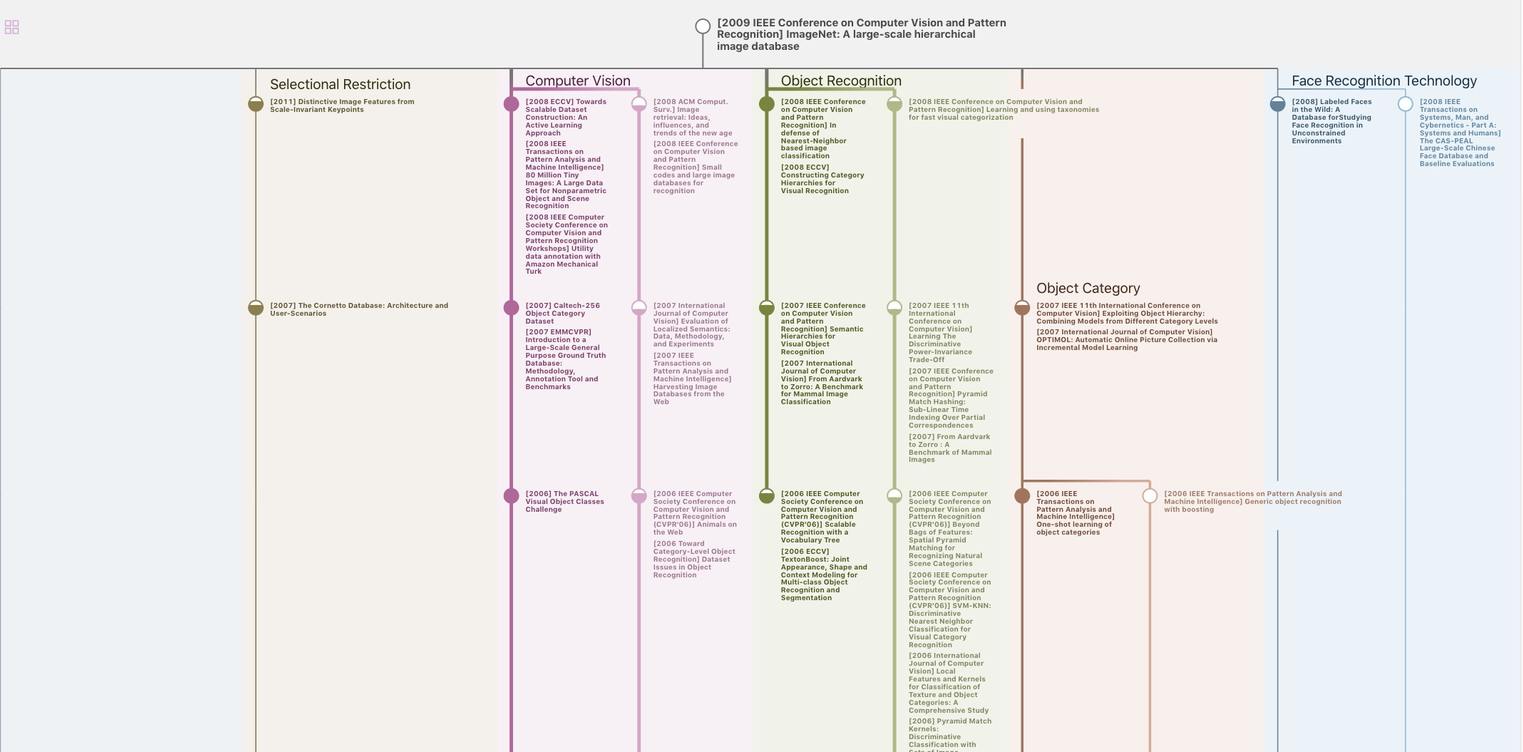
生成溯源树,研究论文发展脉络
Chat Paper
正在生成论文摘要