Applying support vector machines to predict building energy consumption in China
Energy Procedia(2018)
摘要
It is increasingly significant to predict building energy consumption (BEC) in energy-saving decision making. This paper presents the method of support vector machines (SVM) to forecast building energy consumption in China. To improve the reliability of SVM in building energy consumption prediction, multiple parameters including weather data such as yearly mean outdoor dry-bulb temperature, relative humidity and global solar radiation and economic factors such as the ratio of urbanization, gross domestic product, household consumption level and total area of structure are taken as inputs. The performance of SVM with respect to two parameters, c and g, is explored using the k-fold cross validation with grid searching method based on radial basis function kernel. Finally, the performance of the proposed model is checked by comparing the prediction model with statistic data taken from Chinese National Bureau of Statistics in 30 provinces (including autonomous regions and municipalities) of China using statistical error tests such as the mean square error (MSE) and the squared correlation coefficient (r(2)). With the analysis based on statistical error tests, the results show that the SVM method can estimate the building energy consumption with good accuracy with MSE less than 1E-3 and r(2) more than 0.991. Copyright (C) 2018 Elsevier Ltd. All rights reserved.
更多查看译文
关键词
Support vector machine,Building energy consumption,Weather data,Economic factor,Cross validation,Grid search algorithm
AI 理解论文
溯源树
样例
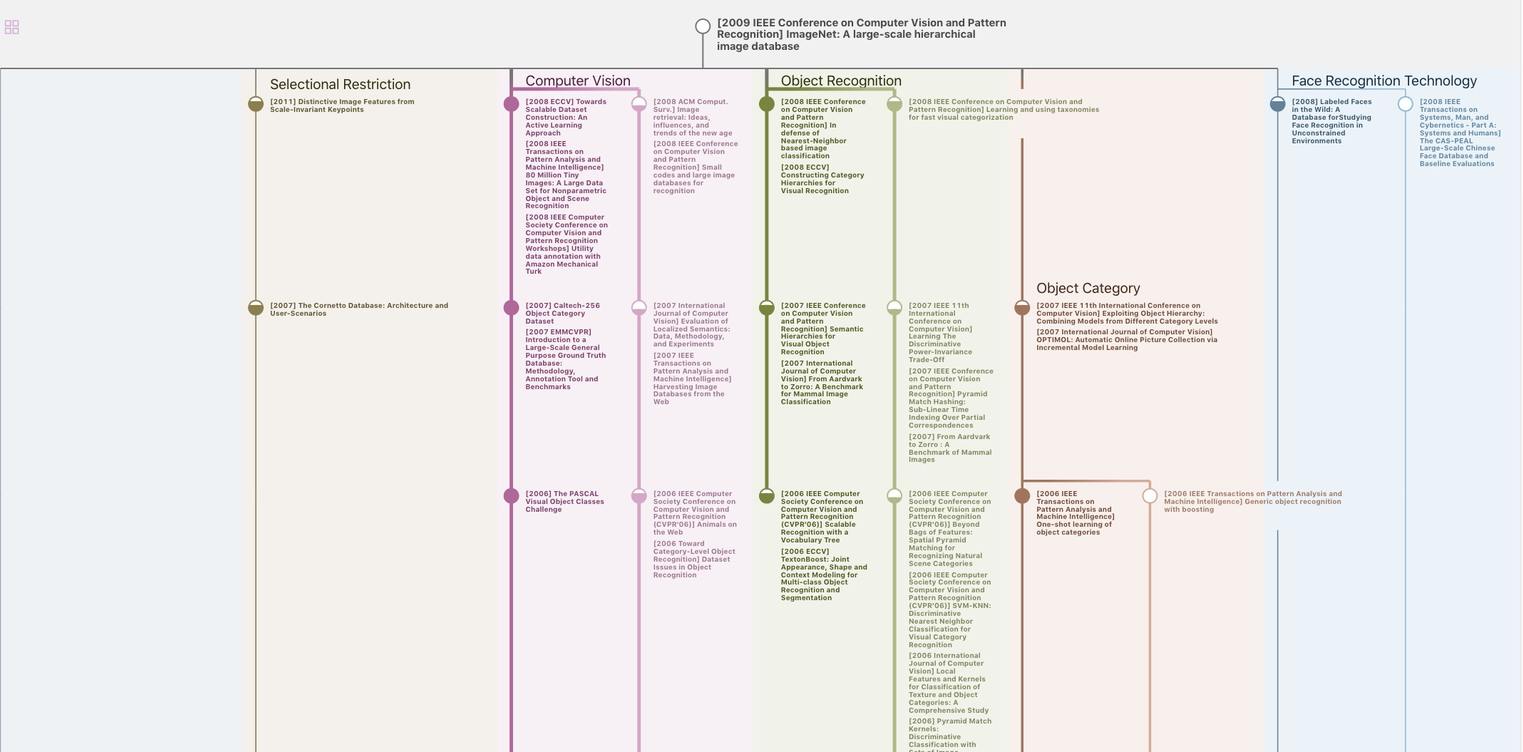
生成溯源树,研究论文发展脉络
Chat Paper
正在生成论文摘要