Semiparametric mixture regression with unspecified error distributions
TEST(2020)
摘要
In fitting a mixture of linear regression models, normal assumption is traditionally used to model the error and then regression parameters are estimated by the maximum likelihood estimators (MLE). This procedure is not valid if the normal assumption is violated. By extending the semiparametric regression estimator proposed by Hunter and Young (J Nonparametr Stat 24:19–38, 2012a) which requires the component error densities to be the same (including homogeneous variance), we propose semiparametric mixture of linear regression models with unspecified component error distributions to reduce the modeling bias. We establish a more general identifiability result under weaker conditions than existing results, construct a class of new estimators, and establish their asymptotic properties. These asymptotic results also apply to many existing semiparametric mixture regression estimators whose asymptotic properties have remained unknown due to the inherent difficulties in obtaining them. Using simulation studies, we demonstrate the superiority of the proposed estimators over the MLE when the normal error assumption is violated and the comparability when the error is normal. Analysis of a newly collected Equine Infectious Anemia Virus data in 2017 is employed to illustrate the usefulness of the new estimator.
更多查看译文
关键词
EM algorithm,Mixture of regressions,Semiparametric mixture models
AI 理解论文
溯源树
样例
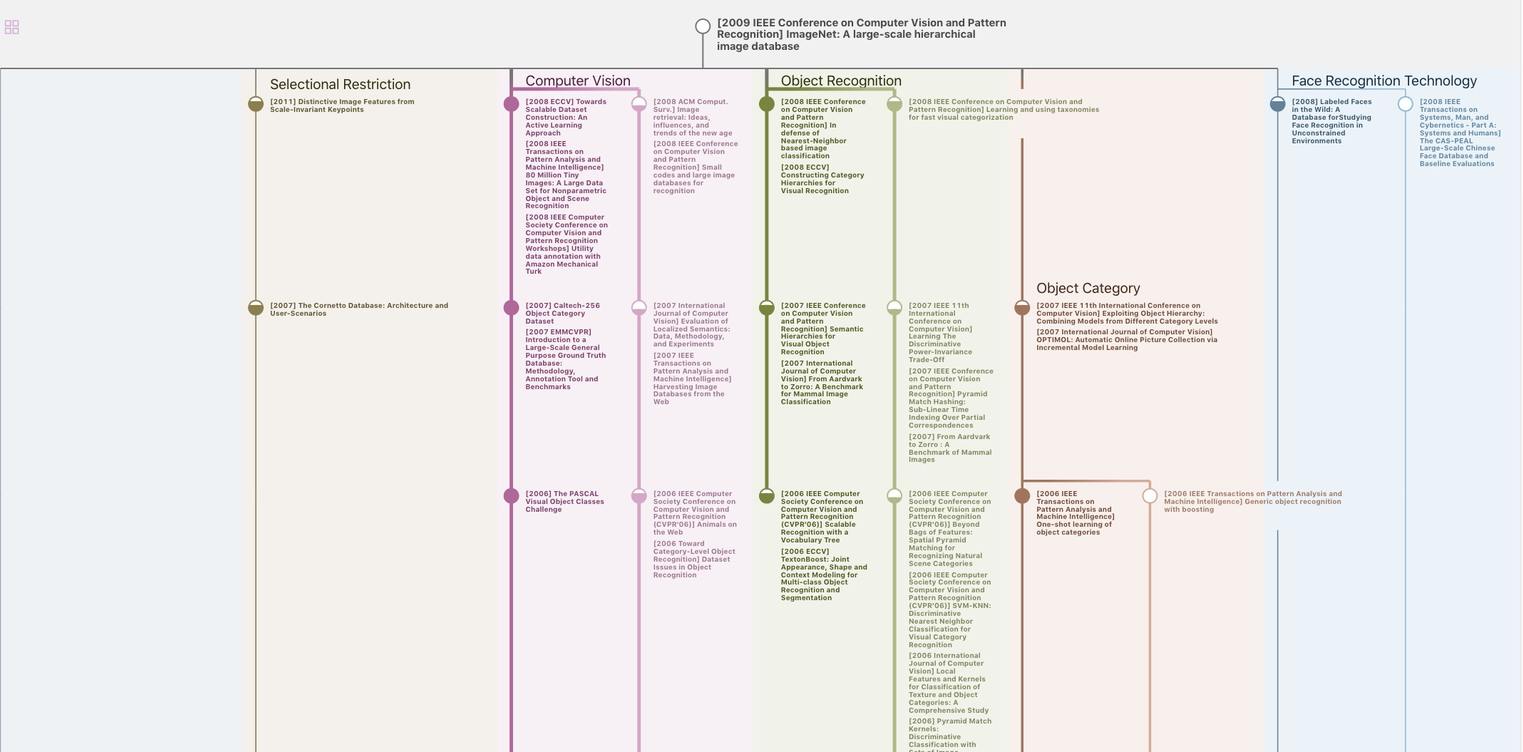
生成溯源树,研究论文发展脉络
Chat Paper
正在生成论文摘要