Multi-View Ensemble Classification For Clinically Actionable Genetic Mutations
NIPS'17 COMPETITION: BUILDING INTELLIGENT SYSTEMS(2018)
摘要
This paper presents details of our solutions to the task IV of NIPS 2017 Competition Track that is called Classifying Clinically Actionable Genetic Mutations. It aims at classifying genetic mutations based on text evidence from clinical literature. A novel multi-view machine learning framework with ensemble classification models is proposed to solve this problem. During this Challenge, feature combinations deriving from three views including document view, entity text view, and entity name view to complement each other are comprehensively explored. Finally, an ensemble of 9 basic gradient boosting models win in the comparisons. Our approach scored 0.5506 and 0.6694 in Logarithmic Loss on a fixed split of stage-1 testing phase and 5-fold cross validation respectively, which is ranked as a top-3 team in NIPS 2017 Competition Track IV.
更多查看译文
关键词
Text Entities, Entity Name, Paper Views, Gradient Boosting Decision Tree (GBDT), Paragraph Vector
AI 理解论文
溯源树
样例
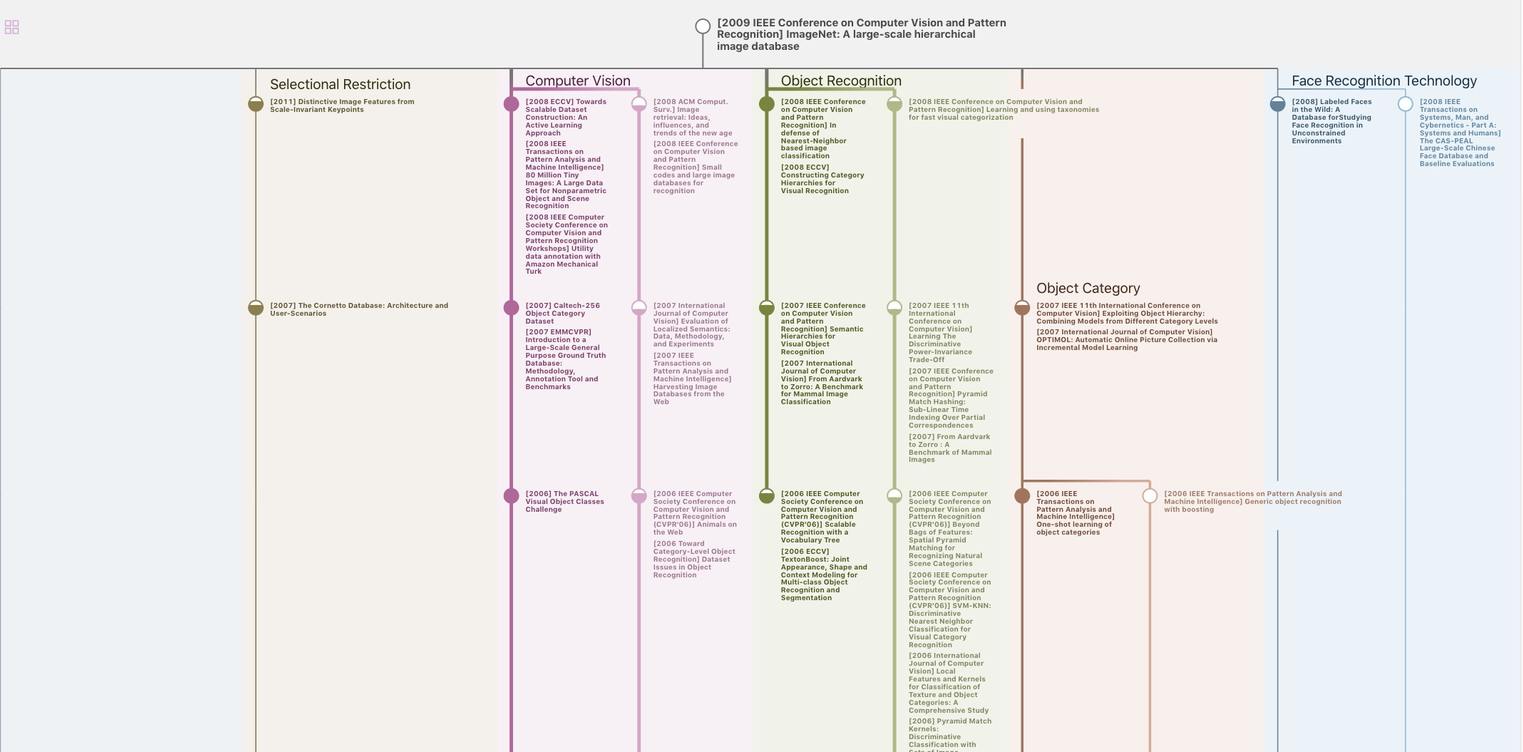
生成溯源树,研究论文发展脉络
Chat Paper
正在生成论文摘要