A Reconfigurable Architecture For Implementing Locally Connected Neural Arrays
INTELLIGENT COMPUTING, VOL 2(2019)
摘要
Moore's law is rapidly approaching a long-predicted decline, and with it the performance gains of conventional processors are becoming ever more marginal. Cognitive computing systems based on neural networks have the potential to provide a solution to the decline of Moore's law. Identifying common traits in neural systems can lead to the design of more efficient, robust and adaptable processors. Despite the potentials, large-scale neural systems remain difficult to implement due to constraints on scalability. Here we introduce a new hardware architecture for implementing locally connected neural networks that can model biological systems with a high level of scalability. We validate our architecture using a full model of the locomotion system of the Caenorhabditis elegans. Further, we show that our proposed architecture archives a ninefold increase in clock speed over existing hardware models. Importantly the clock speed for our architecture is found to be independent of system size, providing an unparalleled level of scalability. Our approach can be applied to the modelling of large neural networks, with greater performance, easier configuration and a high level of scalability.
更多查看译文
关键词
Neural network, Neuromorphic, PNAA, Caenorhabditis elegans, Reconfigurable hardware
AI 理解论文
溯源树
样例
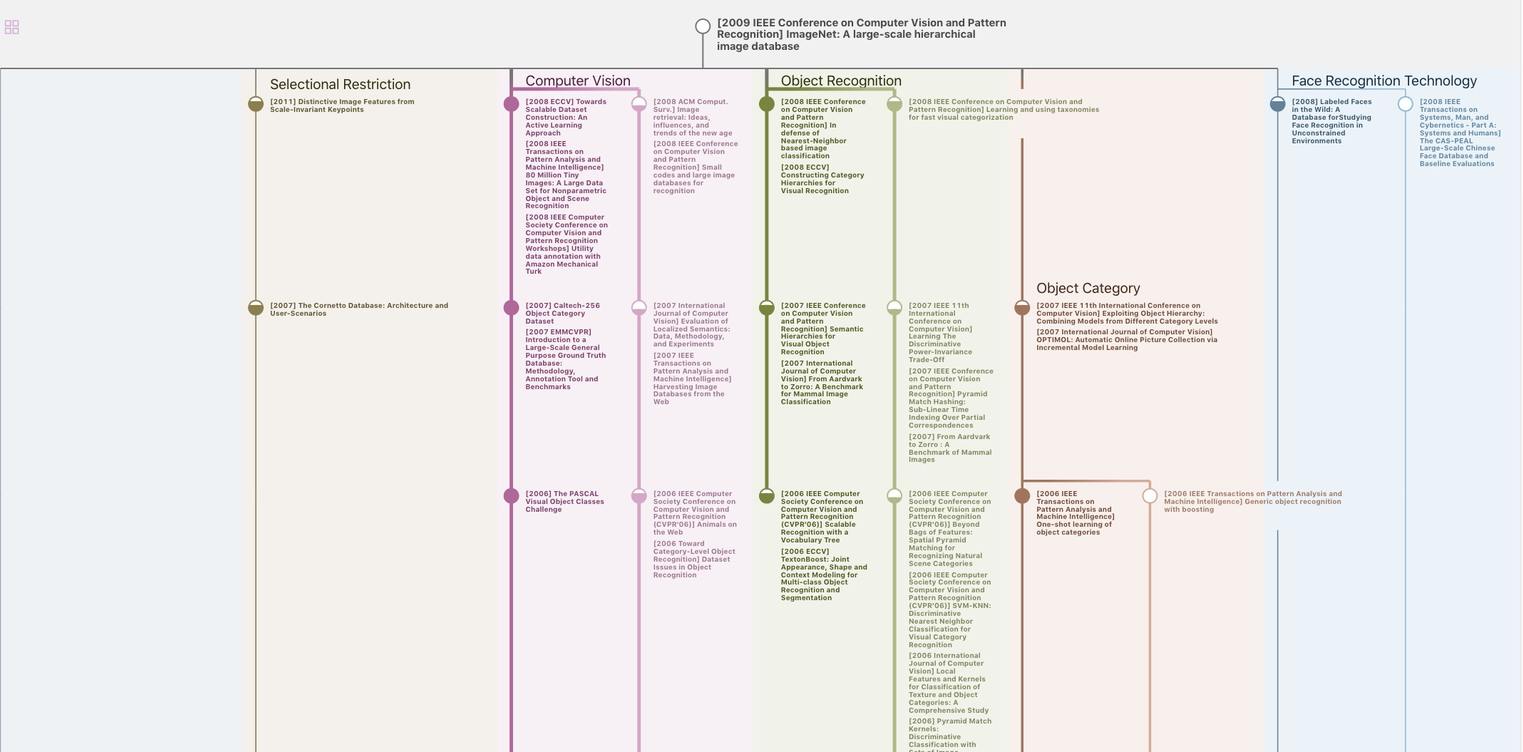
生成溯源树,研究论文发展脉络
Chat Paper
正在生成论文摘要