Extending Gaussian process emulation using cluster analysis and artificial neural networks to fit big training sets
JOURNAL OF SIMULATION(2019)
摘要
Gaussian process (GP) emulation is a relatively recent statistical technique that provides a fast-running approximation to a complex computer model, given training data generated by the considered model. Despite its sound theoretical foundation, GP emulation falls short in practical applications where the training dataset is very large, due to numerical instabilities in inverting the correlation matrix. We show how GP emulation can be extended to handle large training sets by first dividing the training set into smaller subsets using cluster analysis, then training an emulator for each subset, and finally combining the emulators using an artificial neural network (ANN). Our work has also conceptual relevance, as it shows how to solve a big data problem by introducing a local level in input space, where each emulator specialises in a certain subregion, and a global level, where the identified local features of the computer model are combined into a global view.
更多查看译文
关键词
Gaussian process emulation,artificial neural networks,cluster analysis,inverse distance weighting,agent-based models
AI 理解论文
溯源树
样例
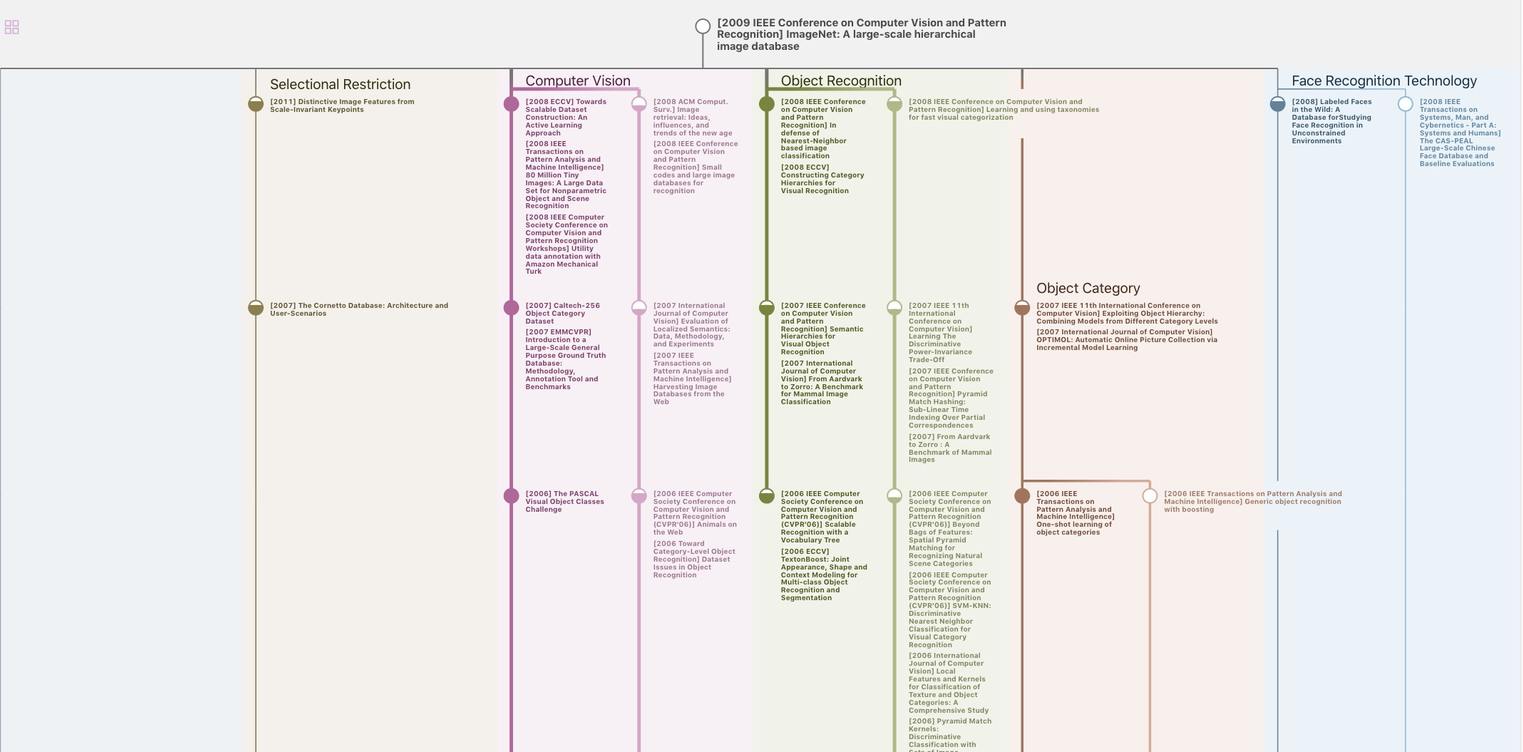
生成溯源树,研究论文发展脉络
Chat Paper
正在生成论文摘要