Dynamic Statistical Models for Pyroclastic Density Current Generation at Soufrière Hills Volcano
FRONTIERS IN EARTH SCIENCE(2018)
摘要
To mitigate volcanic hazards from pyroclastic density currents, volcanologists generate hazard maps that provide long-term forecasts of areas of potential impact. Several recent efforts in the field develop new statistical methods for application of flow models to generate fully probabilistic hazard maps that both account for, and quantify, uncertainty. However, a limitation to the use of most statistical hazard models, and a key source of uncertainty within them, is the time-averaged nature of the datasets by which the volcanic activity is statistically characterized. Where the level, or directionality, of volcanic activity frequently changes, e.g., during protracted eruptive episodes, or at volcanoes that are classified as persistently active, it is not appropriate to make short term forecasts based on longer time-averaged metrics of the activity. Thus, here we build, fit and explore dynamic statistical models for the generation of pyroclastic density currents from Soufriere Hills Volcano (SHV) on Montserrat including their respective collapse direction and flow volumes based on 1996-2008 flow datasets. The development of this approach allows for short-term behavioral changes to be taken into account in probabilistic volcanic hazard assessments. We show that collapses from the SHV lava dome follow a clear pattern, and that a series of smaller flows in a given direction often culminate in a larger collapse and thereafter directionality of the flows changes. Such models enable short term forecasting (weeks to months) that can reflect evolving conditions such as dome and crater morphology changes and non-stationary eruptive behavior such as extrusion rate variations. For example, the probability of inundation of the Belham Valley in the first 180 days of a forecast period is about twice as high for lava domes facing Northwest toward that valley as it is for domes pointing East toward the Tar River Valley. As rich multi-parametric volcano monitoring datasets become increasingly available, eruption forecasting is becoming an increasingly viable and important research field. We demonstrate an approach to utilize such data in order to appropriately tune probabilistic hazard assessments for pyroclastic flows. Our broader objective with development of this method is to help advance time-dependent volcanic hazard assessment, by bridging the gap between eruption forecasting based on monitoring time series data and development of cutting edge probabilistic volcanic hazard maps.
更多查看译文
关键词
dynamic,forecast,objective Bayesian,Pareto,von Mises,probabilistic,pyroclastic
AI 理解论文
溯源树
样例
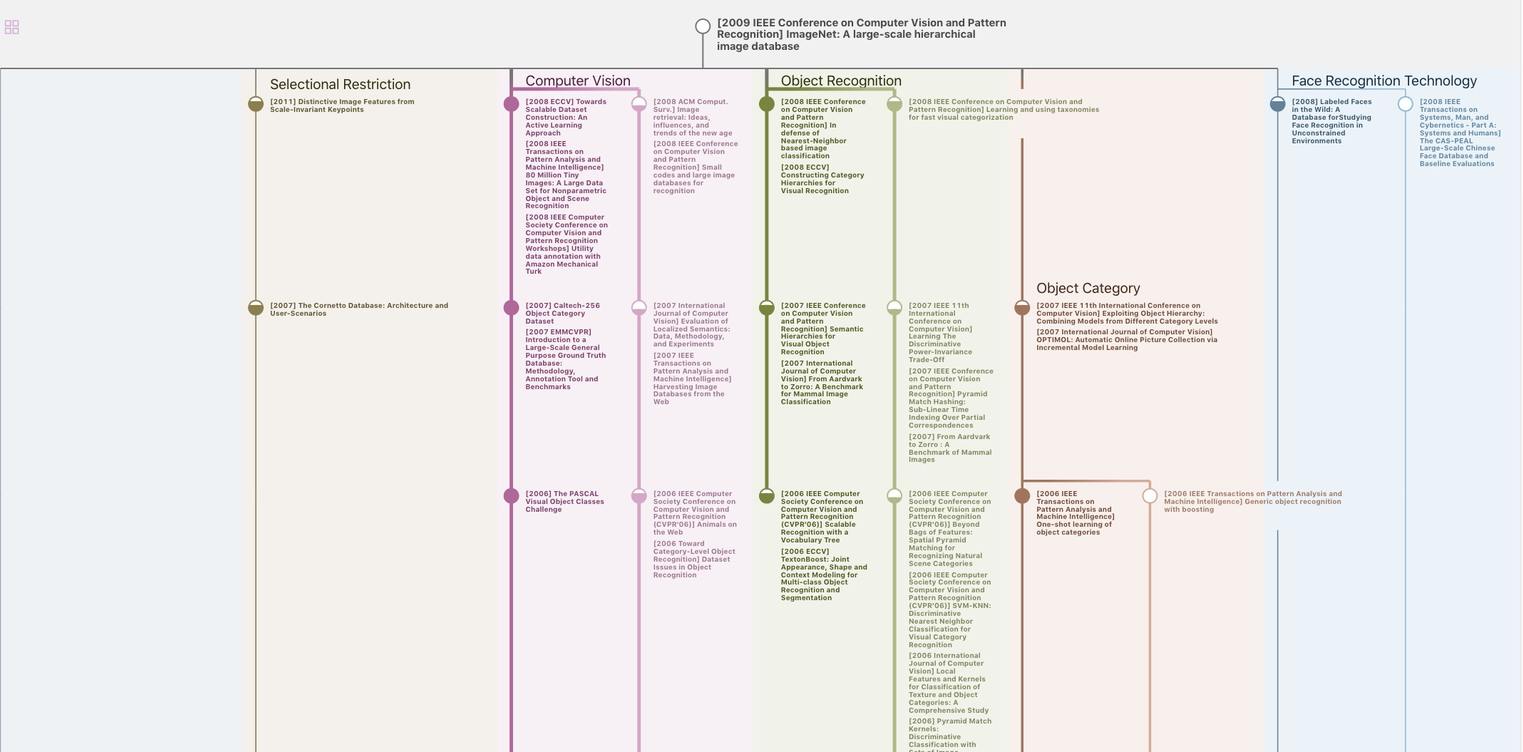
生成溯源树,研究论文发展脉络
Chat Paper
正在生成论文摘要