Rotor Position Self-Sensing of SRM Using PSO-RVM
ENERGIES(2018)
摘要
The motors' flux-linkage, current and angle obtained from the system with sensors were chosen as the sample data, and the estimation model of rotor position based on relevance vector machine (RVM) was built by training the sample data. The kernel function parameter in RVM model was optimized by the particle swarm algorithm in order to increase the fitting precision and generalization ability of RVM model. It achieved higher prediction accuracy with staying at the same on-line testing time as the RVM. And because the short on-line computation, the motor can operate at 3000 r/min in sensorless control with particle swarm optimization-relevance vector machine (PSO-RVM), which is higher than support vector machine (SVM) and neural network (NN). By simulation and experiment on the test motor, it is verified that the proposed estimation model can obtain the angle of full electrical period accurately under low speed and high speed operations in current chopped control and angle position control, which has satisfactory estimation precision.
更多查看译文
关键词
relevance vector machine (RVM),particle swarm,switched reluctance motor,estimation model,self-sensing
AI 理解论文
溯源树
样例
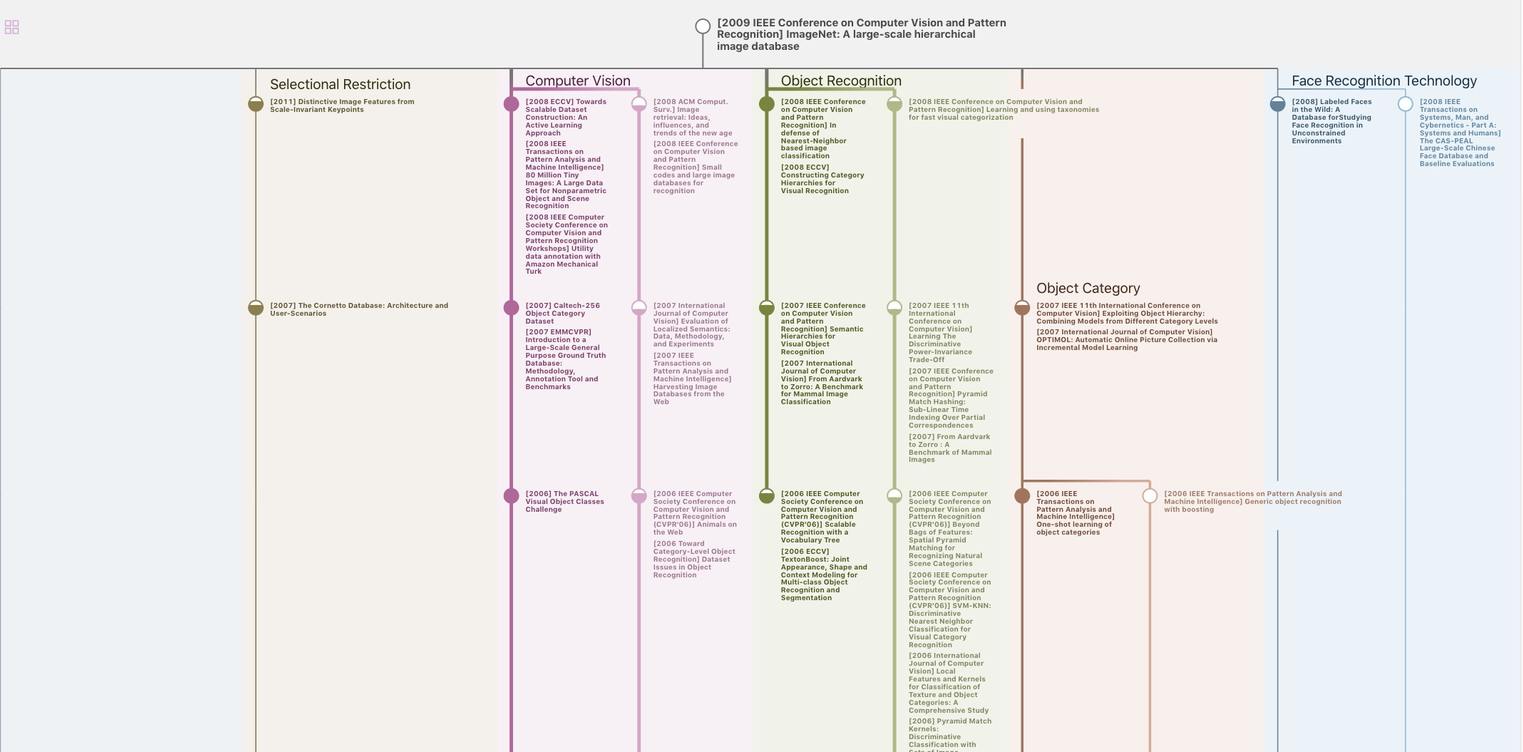
生成溯源树,研究论文发展脉络
Chat Paper
正在生成论文摘要