Learning Compressible 360 Degrees Video Isomers
2018 IEEE/CVF CONFERENCE ON COMPUTER VISION AND PATTERN RECOGNITION (CVPR)(2018)
摘要
Standard video encoders developed for conventional narrow field-of-view video are widely applied to 360 degrees video as well, with reasonable results. However while this approach commits arbitrarily to a projection of the spherical frames, we observe that some orientations of a 360 degrees video, once projected, are more compressible than others. We introduce an approach to predict the sphere rotation that will yield the maximal compression rate. Given video clips in their original encoding, a convolutional neural network learns the association between a clip's visual content and its compressibility at different rotations of a cubemap projection. Given a novel video, our learning-based approach efficiently infers the most compressible direction in one shot, without repeated rendering and compression of the source video. We validate our idea on thousands of video clips and multiple popular video codecs. The results show that this untapped dimension of 360 degrees compression has substantial potential-"good" rotations are typically 8-10% more compressible than bad ones, and our learning approach can predict them reliably 82% of the time.
更多查看译文
AI 理解论文
溯源树
样例
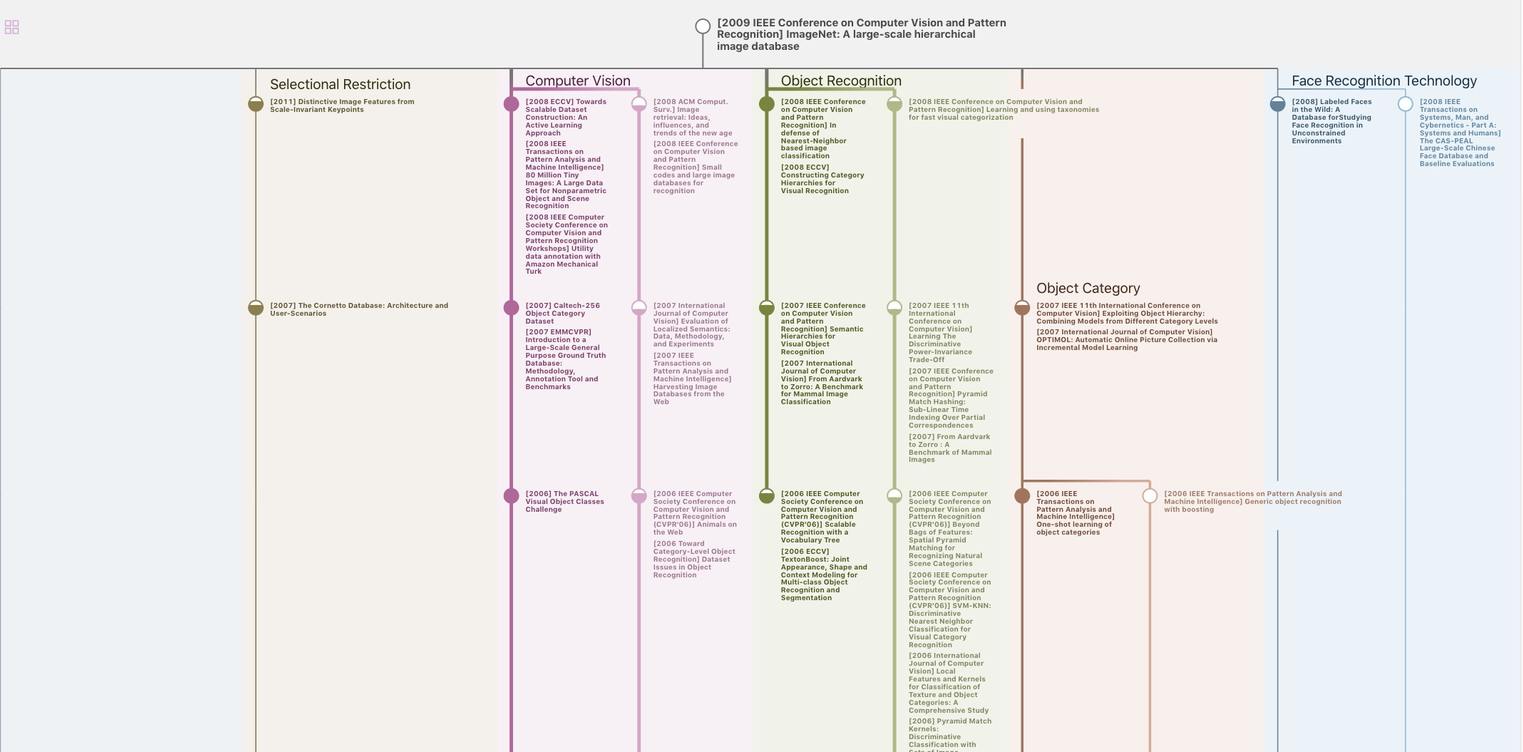
生成溯源树,研究论文发展脉络
Chat Paper
正在生成论文摘要