Arabic named entity recognition via deep co-learning
Artificial Intelligence Review(2019)
摘要
Named entity recognition (NER) is an important natural language processing (NLP) task with many applications. We tackle the problem of Arabic NER using deep learning based on Arabic word embeddings that capture syntactic and semantic relationships between words. Deep learning has been shown to perform significantly better than other approaches for various NLP tasks including NER. However, deep-learning models also require a significantly large amount of training data, which is highly lacking in the case of the Arabic language. To remedy this, we adopt the semi-supervised co-training approach to the realm of deep learning, which we refer to as deep co-learning . Our deep co-learning approach makes use of a small amount of labeled data, which is augmented with partially labeled data that is automatically generated from Wikipedia. Our approach relies only on word embeddings as features and does not involve any additional feature engineering. Nonetheless, when tested on three different Arabic NER benchmarks, our approach consistently outperforms state-of-the-art Arabic NER approaches, including ones that employ carefully-crafted NLP features. It also consistently outperforms various baselines including purely-supervised deep-learning approaches as well as semi-supervised ones that make use of only unlabeled data such as self-learning and the traditional co-training approach.
更多查看译文
关键词
NER, Arabic NLP, Co-training, Deep Learning
AI 理解论文
溯源树
样例
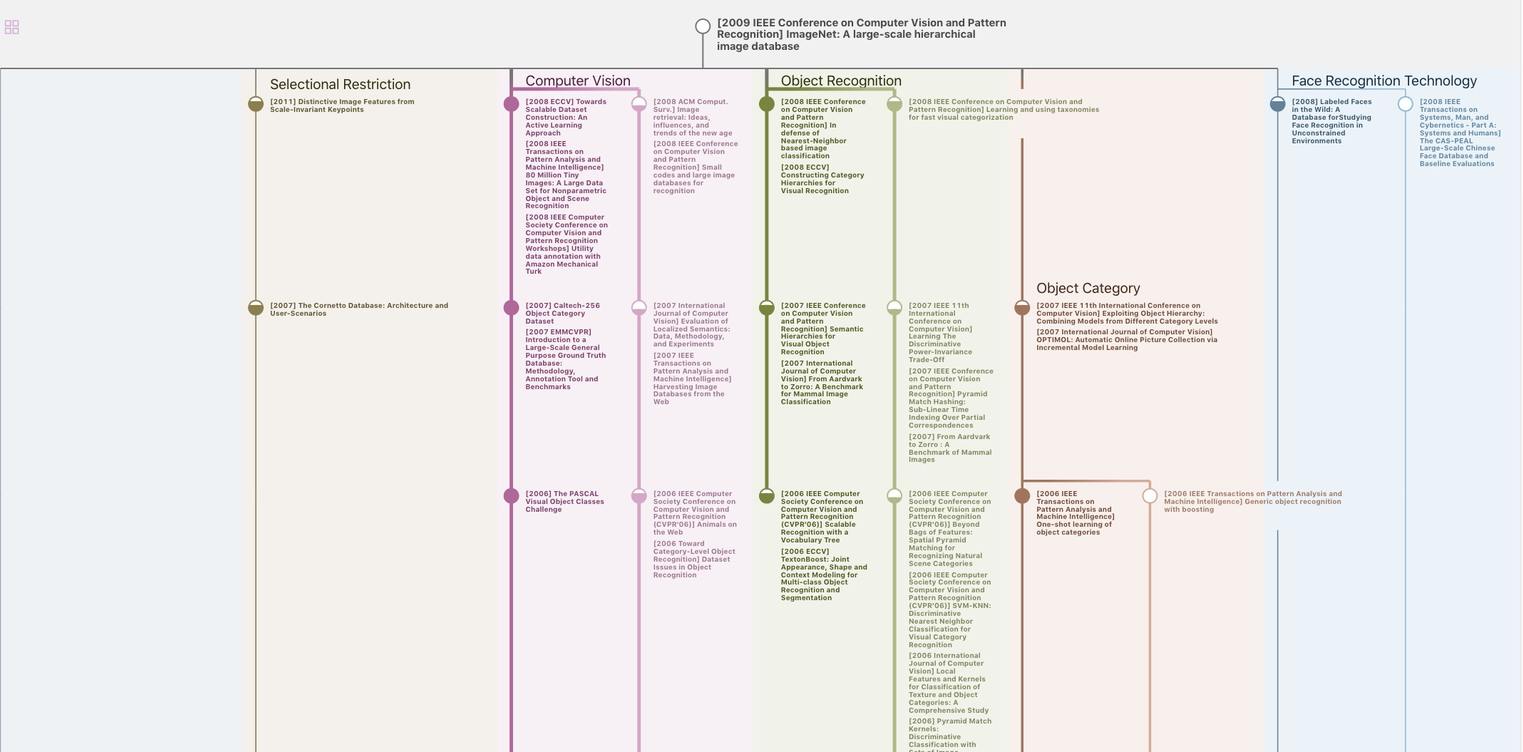
生成溯源树,研究论文发展脉络
Chat Paper
正在生成论文摘要