High‐resolution riparian vegetation mapping to prioritize conservation and restoration in an impaired desert river
RESTORATION ECOLOGY(2017)
摘要
In highly impaired watersheds, it is critical to identify both areas with desirable habitat as conservation zones and impaired areas with the highest likelihood of improvement as restoration zones. We present how detailed riparian vegetation mapping can be used to prioritize conservation and restoration sites within a riparian and instream habitat restoration program targeting 3 native fish species on the San Rafael River, a desert river in southeastern Utah, United States. We classified vegetation using a combination of object-based image analysis (OBIA) on high-resolution (0.5m), multispectral, satellite imagery with oblique aerial photography and field-based data collection. The OBIA approach is objective, repeatable, and applicable to large areas. The overall accuracy of the classification was 80% (Cohen's =0.77). We used this high-resolution vegetation classification alongside existing data on habitat condition and aquatic species' distributions to identify reaches' conservation value and restoration potential to guide management actions. Specifically, cottonwood (Populus fremontii) and tamarisk (Tamarix ramosissima) density layers helped to establish broad restoration and conservation reach classes. The high-resolution vegetation mapping precisely identified individual cottonwood trees and tamarisk thickets, which were used to determine specific locations for restoration activities such as beaver dam analogue structures in cottonwood restoration areas, or strategic tamarisk removal in high-density tamarisk sites. The site prioritization method presented here is effective for planning large-scale river restoration and is transferable to other desert river systems elsewhere in the world.
更多查看译文
关键词
GIS,habitat assessment,native fish,object-based image analysis,San Rafael River,vegetation classification
AI 理解论文
溯源树
样例
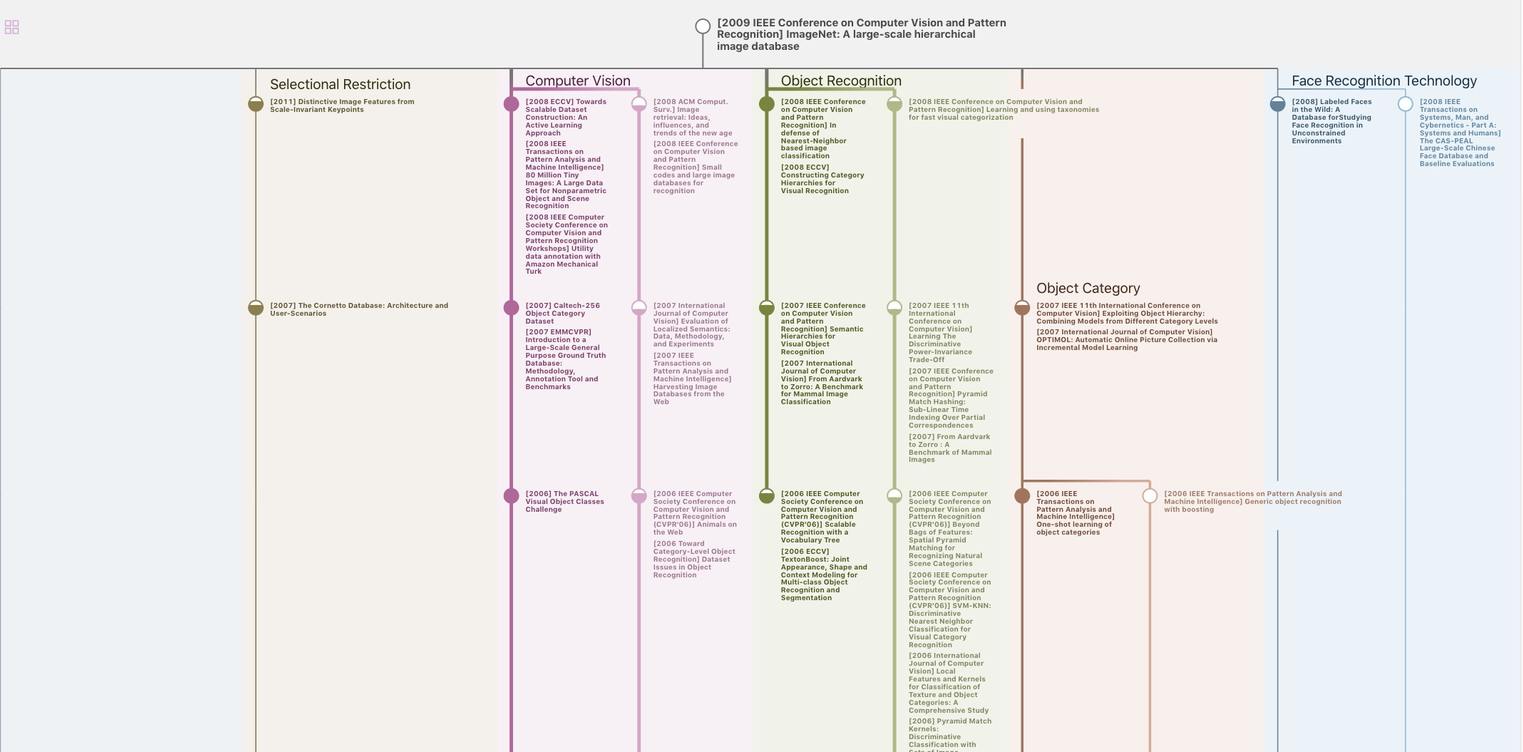
生成溯源树,研究论文发展脉络
Chat Paper
正在生成论文摘要