A statistical approach for identifying single nucleotide variants that affect transcription factor binding
iScience(2024)
摘要
Non-coding variants located within regulatory elements may alter gene expression by modifying Transcription Factor (TF) binding sites, thereby leading to functional consequences. Different TF models are being used to assess the effect of DNA sequence variants, such as Single Nucleotide Variants (SNVs). Often existing methods are slow and do not assess statistical significance of results. We investigated the distribution of absolute maximal differential TF binding scores for general computational models that affect TF binding. We find that a modified Laplace distribution can adequately approximate the empirical distributions. A benchmark on in vitro and in vivo datasets showed that our approach improves upon an existing method in terms of performance and speed. Applications on eQTLs and on a genome-wide association study illustrate the usefulness of our statistic by highlighting cell type-specific regulators and target genes. An implementation of our approach is freely available at https://github.com/SchulzLab/SNEEP and as bioconda package.
更多查看译文
AI 理解论文
溯源树
样例
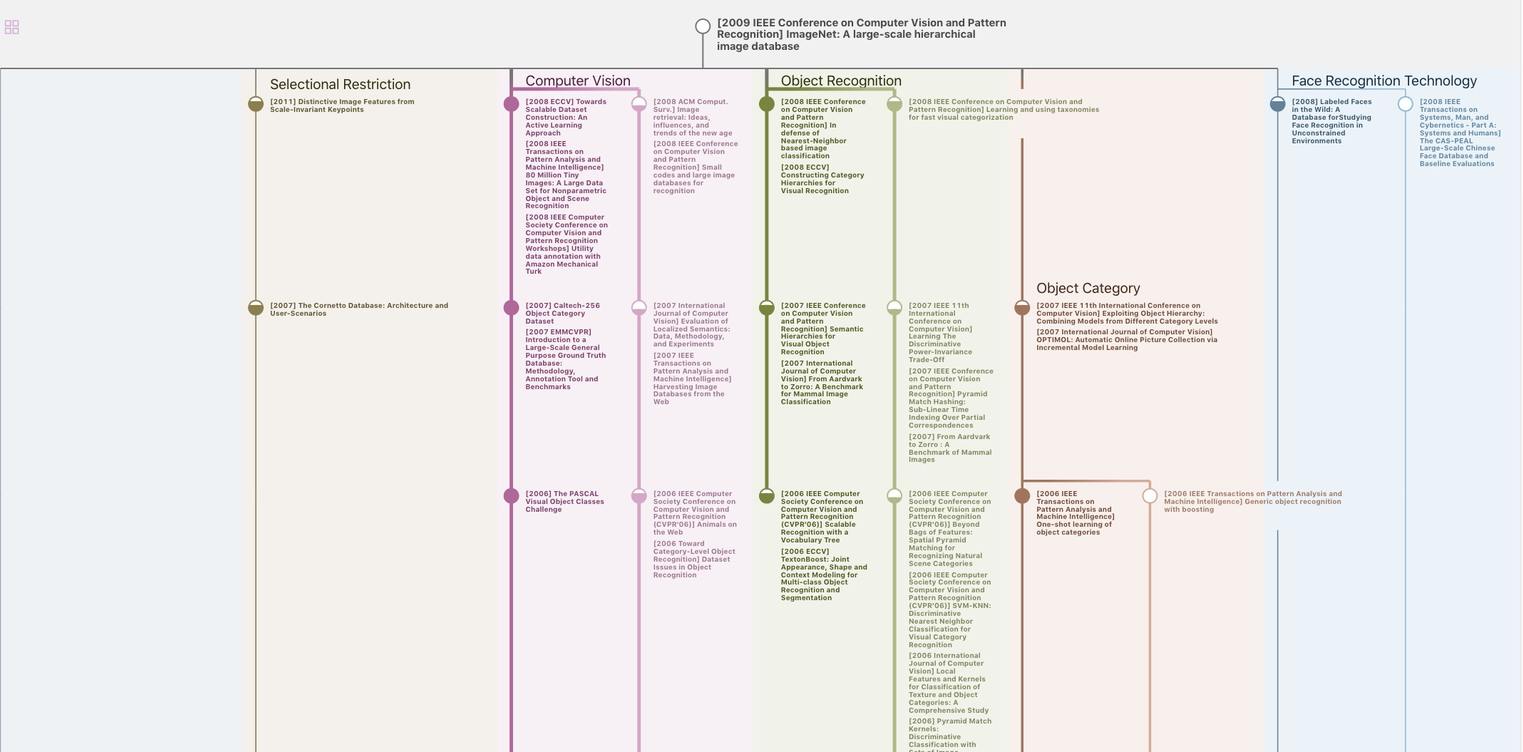
生成溯源树,研究论文发展脉络
Chat Paper
正在生成论文摘要