Supervised Learning Of Two-Layer Perceptron Under The Existence Of External Noise - Learning Curve Of Boolean Functions Of Two Variables In Tree-Like Architecture
JOURNAL OF THE PHYSICAL SOCIETY OF JAPAN(2016)
摘要
We investigate the supervised batch learning of Boolean functions expressed by a two-layer perceptron with a tree-like structure. We adopt continuous weights (spherical model) and the Gibbs algorithm. We study the Parity and And machines and two types of noise, input and output noise, together with the noiseless case. We assume that only the teacher suffers from noise. By using the replica method, we derive the saddle point equations for order parameters under the replica symmetric (RS) ansatz. We study the critical value alpha(C) of the loading rate a above which the learning phase exists for cases with and without noise. We find that alpha(C) is nonzero for the Parity machine, while it is zero for the And machine. We derive the exponents (beta) over bar of order parameters expressed as (alpha - alpha C)((beta) over bar) when alpha is near to alpha(C). Furthermore, in the Parity machine, when noise exists, we find a spin glass solution, in which the overlap between the teacher and student vectors is zero but that between student vectors is nonzero. We perform Markov chain Monte Carlo simulations by simulated annealing and also by exchange Monte Carlo simulations in both machines. In the Parity machine, we study the de Almeida-Thouless stability, and by comparing theoretical and numerical results, we find that there exist parameter regions where the RS solution is unstable, and that the spin glass solution is metastable or unstable. We also study asymptotic learning behavior for large alpha and derive the exponents (beta) over cap of order parameters expressed as alpha(-(beta) over cap) when alpha is large in both machines. By simulated annealing simulations, we confirm these results and conclude that learning takes place for the input noise case with any noise amplitude and for the output noise case when the probability that the teacher's output is reversed is less than one-half.
更多查看译文
AI 理解论文
溯源树
样例
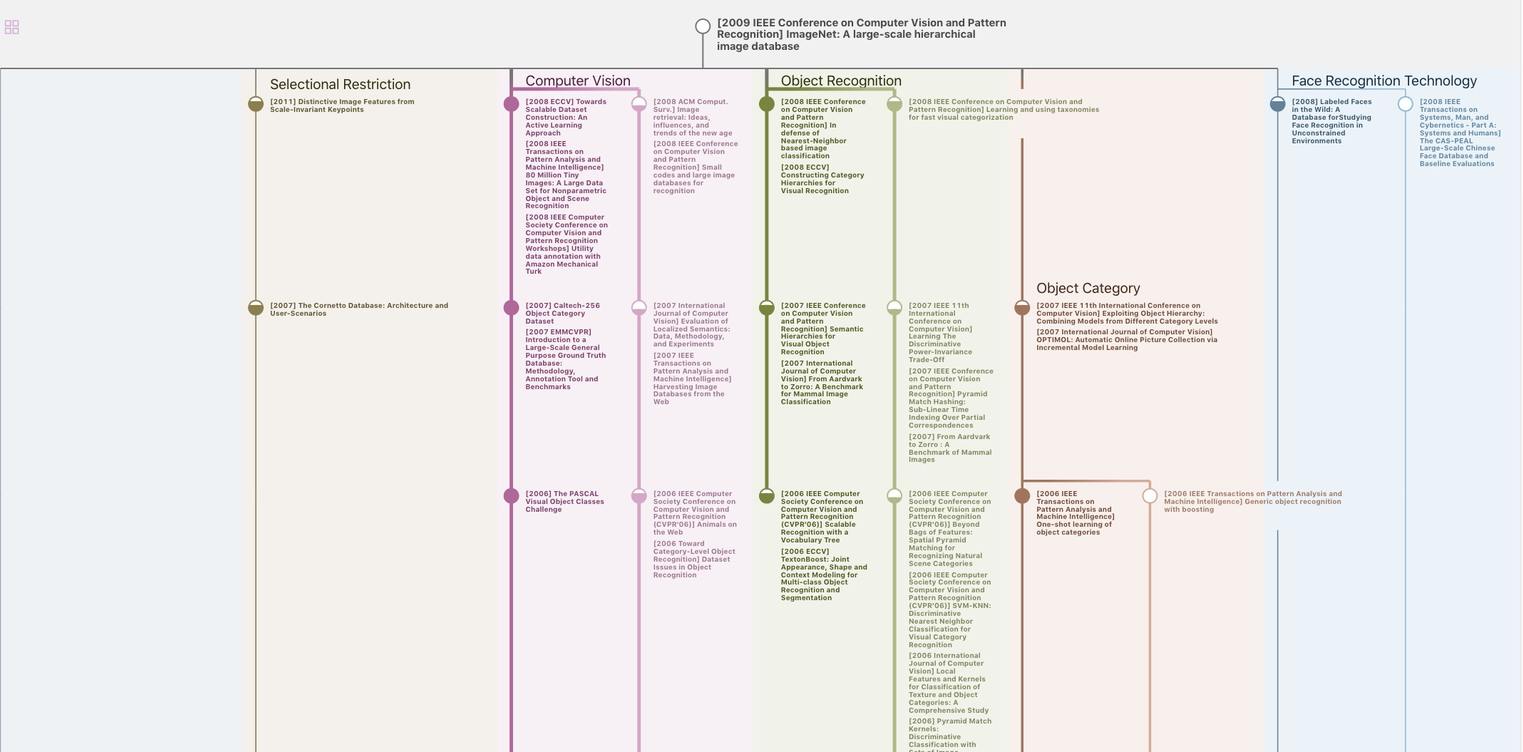
生成溯源树,研究论文发展脉络
Chat Paper
正在生成论文摘要