Toward automated left ventricle segmentation of whole-cardiac-cycle MR images via Contextual-Feature-Induced Semantic Flow Propagation Network
Measurement(2023)
摘要
This article proposes an automated left ventricle (LV) segmentation model named Contextual-Feature-Induced Semantic Flow Propagation Network (CFISFPNet). The CFISFPNet leverages the convolutional GRU (ConvGRU) to extract contextual features and incorporates the optical flow technique to learn semantic flow information between adjacent MRI feature-maps. By propagating high-level semantic features into high-resolution feature-maps, CFISFPNet achieves accurate LV region segmentation at any phase in a cardiac cycle. Extensive confirmatory and comparative experiments have been conducted on three benchmark datasets: the Automated Cardiac Diagnosis Challenge, the Multi-Centre, Multi-Vendor & Multi-Disease Cardiac Image Segmentation Challenge, and the Toronto Children’s dataset. The experimental results show that the CFISFPNet achieves Dice scores of 88.09%, 85.85%, and 86.99%, as well as Hausdorff distance metrics of 2.04, 2.27, and 2.07 on the respective datasets. These results outperform state-of-the-art approaches and approach manual segmentation performance of clinical experts. CFISFPNet exhibits significant potential in enhancing cardiovascular disease clinical diagnosis and treatment.
更多查看译文
关键词
left ventricle segmentation,left ventricle,flow,whole-cardiac-cycle,contextual-feature-induced
AI 理解论文
溯源树
样例
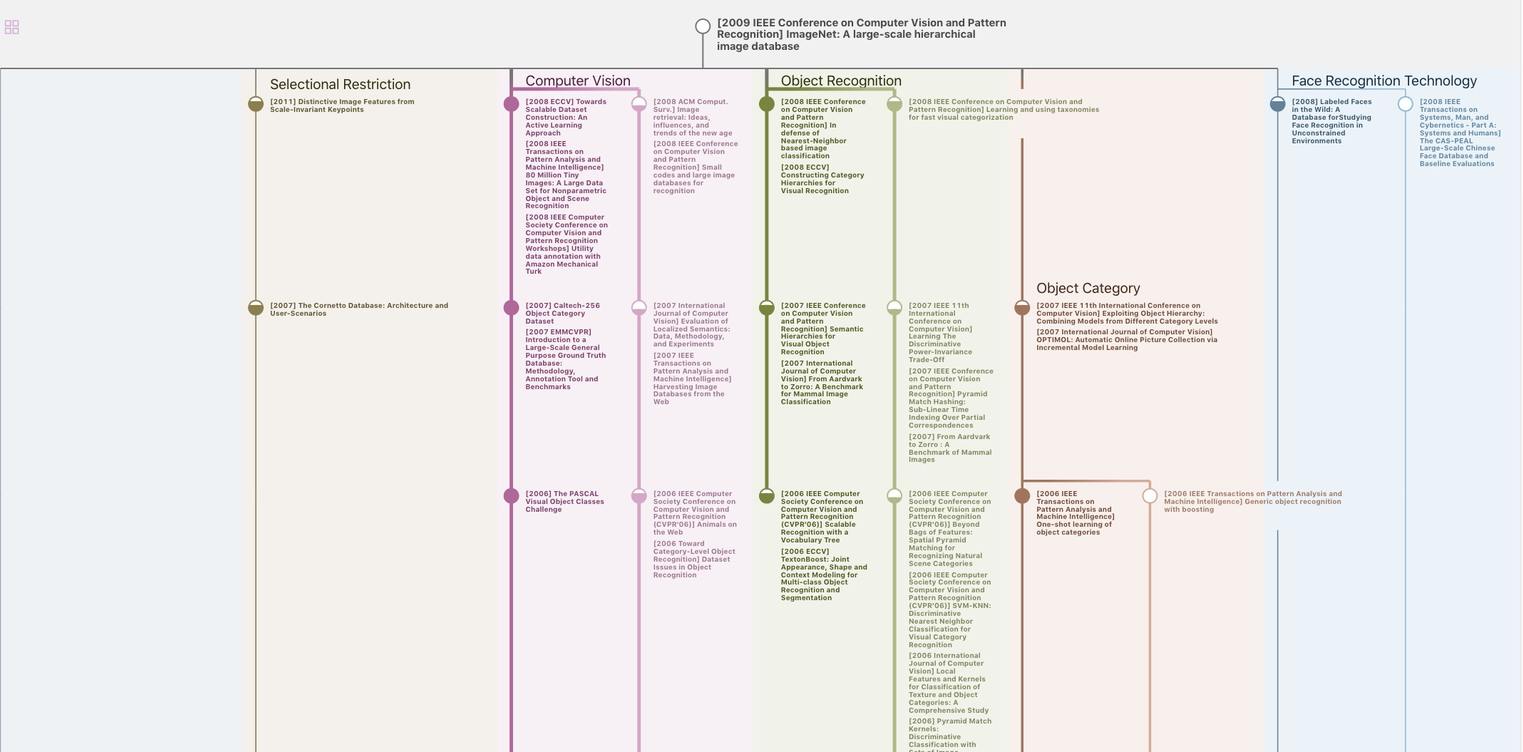
生成溯源树,研究论文发展脉络
Chat Paper
正在生成论文摘要