Movie genome: alleviating new item cold start in movie recommendation
User Modeling and User-Adapted Interaction(2019)
摘要
As of today, most movie recommendation services base their recommendations on collaborative filtering (CF) and/or content-based filtering (CBF) models that use metadata (e.g., genre or cast). In most video-on-demand and streaming services, however, new movies and TV series are continuously added. CF models are unable to make predictions in such a scenario, since the newly added videos lack interactions—a problem technically known as new item cold start (CS). Currently, the most common approach to this problem is to switch to a purely CBF method, usually by exploiting textual metadata. This approach is known to have lower accuracy than CF because it ignores useful collaborative information and relies on human-generated textual metadata, which are expensive to collect and often prone to errors. User-generated content, such as tags, can also be rare or absent in CS situations. In this paper, we introduce a new movie recommender system that addresses the new item problem in the movie domain by (i) integrating state-of-the-art audio and visual descriptors, which can be automatically extracted from video content and constitute what we call the movie genome ; (ii) exploiting an effective data fusion method named canonical correlation analysis , which was successfully tested in our previous works Deldjoo et al. (in: International Conference on Electronic Commerce and Web Technologies. Springer, Berlin, pp 34–45, 2016b ; Proceedings of the Twelfth ACM Conference on Recommender Systems. ACM, 2018b ), to better exploit complementary information between different modalities; (iii) proposing a two-step hybrid approach which trains a CF model on warm items (items with interactions) and leverages the learned model on the movie genome to recommend cold items (items without interactions). Experimental validation is carried out using a system-centric study on a large-scale, real-world movie recommendation dataset both in an absolute cold start and in a cold to warm transition; and a user-centric online experiment measuring different subjective aspects, such as satisfaction and diversity. Results show the benefits of this approach compared to existing approaches.
更多查看译文
关键词
Movie recommender systems,Cold start,Warm start,Semi-cold start,New item,Multimedia features,Content-based,Audio descriptors,Visual descriptors,Multimodal fusion,Hybrid recommender system,Feature weighting,Collaborative-enriched content-based filtering,Canonical correlations analysis
AI 理解论文
溯源树
样例
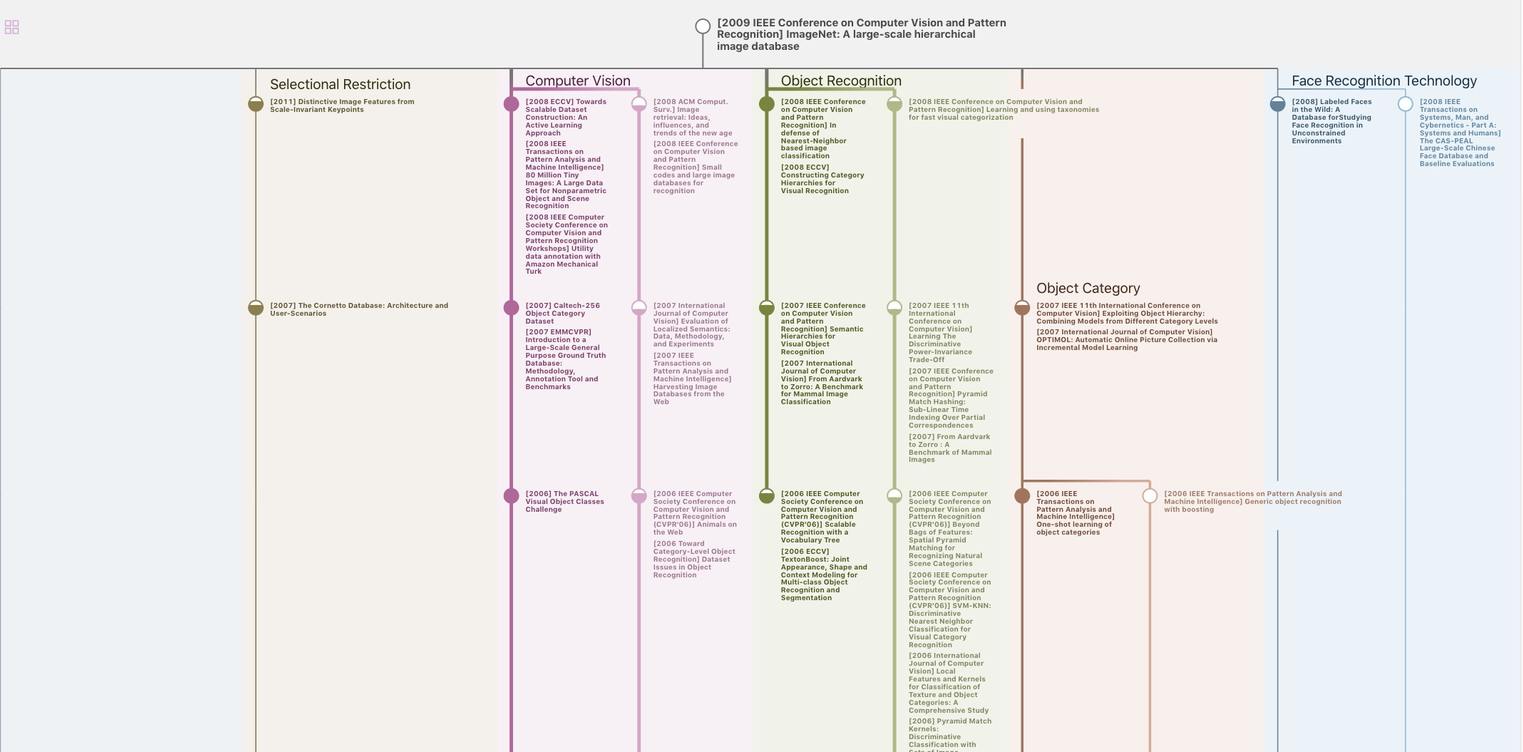
生成溯源树,研究论文发展脉络
Chat Paper
正在生成论文摘要