Nonlocal low-rank regularization for human motion recovery based on similarity analysis.
Information Sciences(2019)
摘要
Human motion capture(mocap) data records movement information from markers attached to human joints and has been widely used in animation productions. However, due to lack of accuracy of the motion capture system and the occlusion of the markers, the original mocap data may be missing over a period of time. Low-rank matrix completion(LRMC) has recently provided remarkable results in the human motion recovery. These LRMC methods assume that the entire data is of low-rank, ignoring the rich similarity among human poses in the space-time dimension, which may result in an inability to recover the details of human motion with complex and diverse structures.Therefore, we propose a novel nonlocal low-rank regularization(NLR) method to model the structured sparsity according to the similarity of human motion, and we explore its application in human motion recovery tasks. Moreover, in order to better exploit the low-rank properties, weighted Schatten p-norm(WSN) is proposed to treat each rank component differently. Then, the recovery process also effectively utilizes kinematic information(i.e., bone-length constraint) to maintain the visual naturalness of the recovery results. Extensive experiments compared with other state-of-the-art methods demonstrate the superiority of the proposed model in terms of recovery accuracy and visualization results.
更多查看译文
关键词
Motion capture data,Motion recovery,Low-rank matrix completion,Nonlocal regularization
AI 理解论文
溯源树
样例
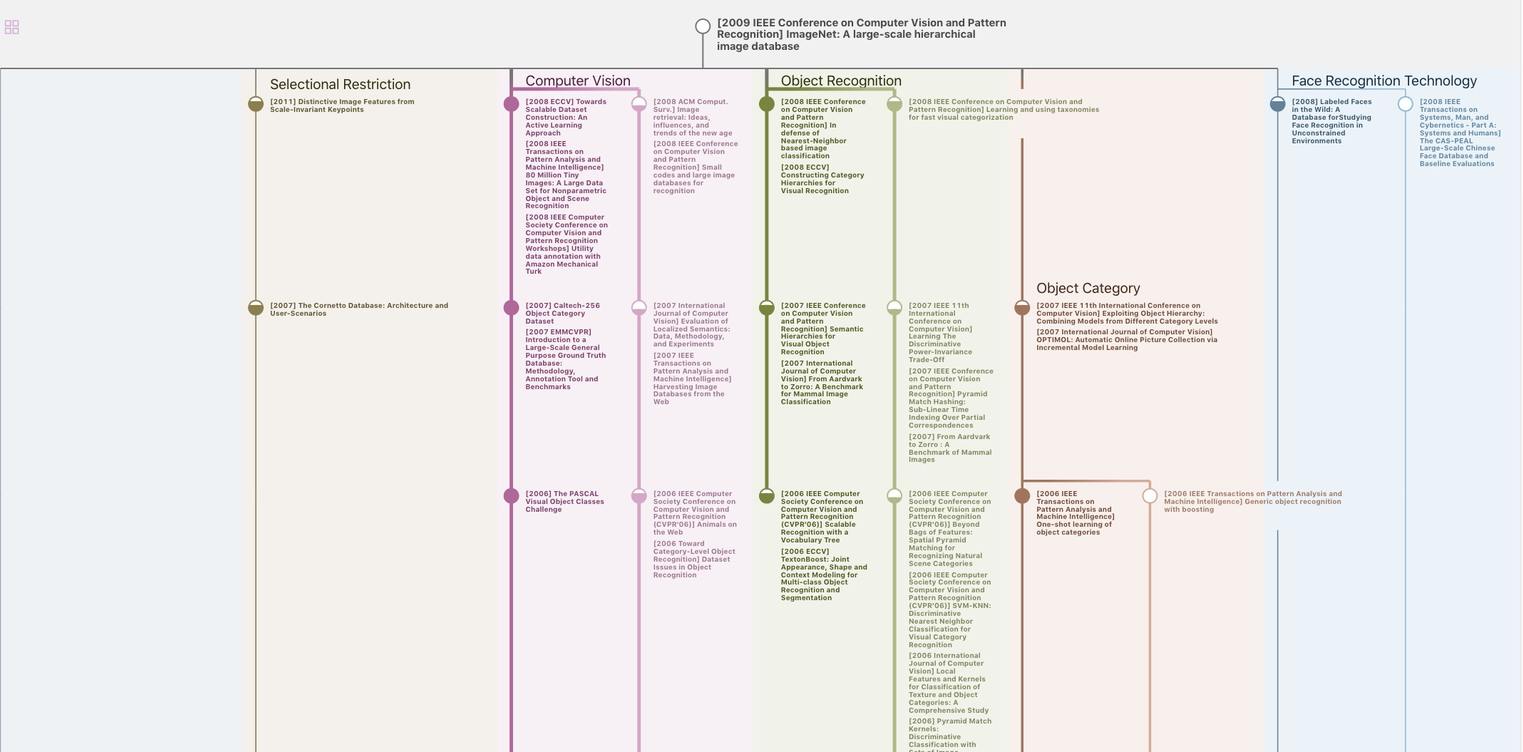
生成溯源树,研究论文发展脉络
Chat Paper
正在生成论文摘要