Sparse Representations for the Spectral–Spatial Classification of Hyperspectral Image
Journal of the Indian Society of Remote Sensing(2018)
摘要
In this paper, we propose a new sparsity-based approach for the spectral–spatial classification of hyperspectral imagery. The proposed approach exploits the sparse representations of the spectral and spatial information contained in the data to generate an accurate classification map; specifically, we use all the spectral information (reflectance registered in the bands) and extended multiattribute profiles to extract spatial features. Hyperspectral image classification with sparse representations is based on the study that a pixel can be sparsely represented by a linear combination of a few learning examples from a structured dictionary. Then, by giving the set of training samples, any given sample may be sparsely represented by solving a sparsity-constrained optimization problem and thus classified in the class that minimizes a residual function. In this paper, we propose a new residual function which combines the sparse representations of the spectral features and the sparse representations of the spatial features to determine the class label of the test sample. Experiments are conducted on the familiar AVIRIS “Indian Pines” data set. It was found that the proposed method provided more accurate classification results than SVM with composite kernel.
更多查看译文
关键词
Classification, Hyperspectral images, Spectral features, Spatial features, EMAPs, Sparse representation
AI 理解论文
溯源树
样例
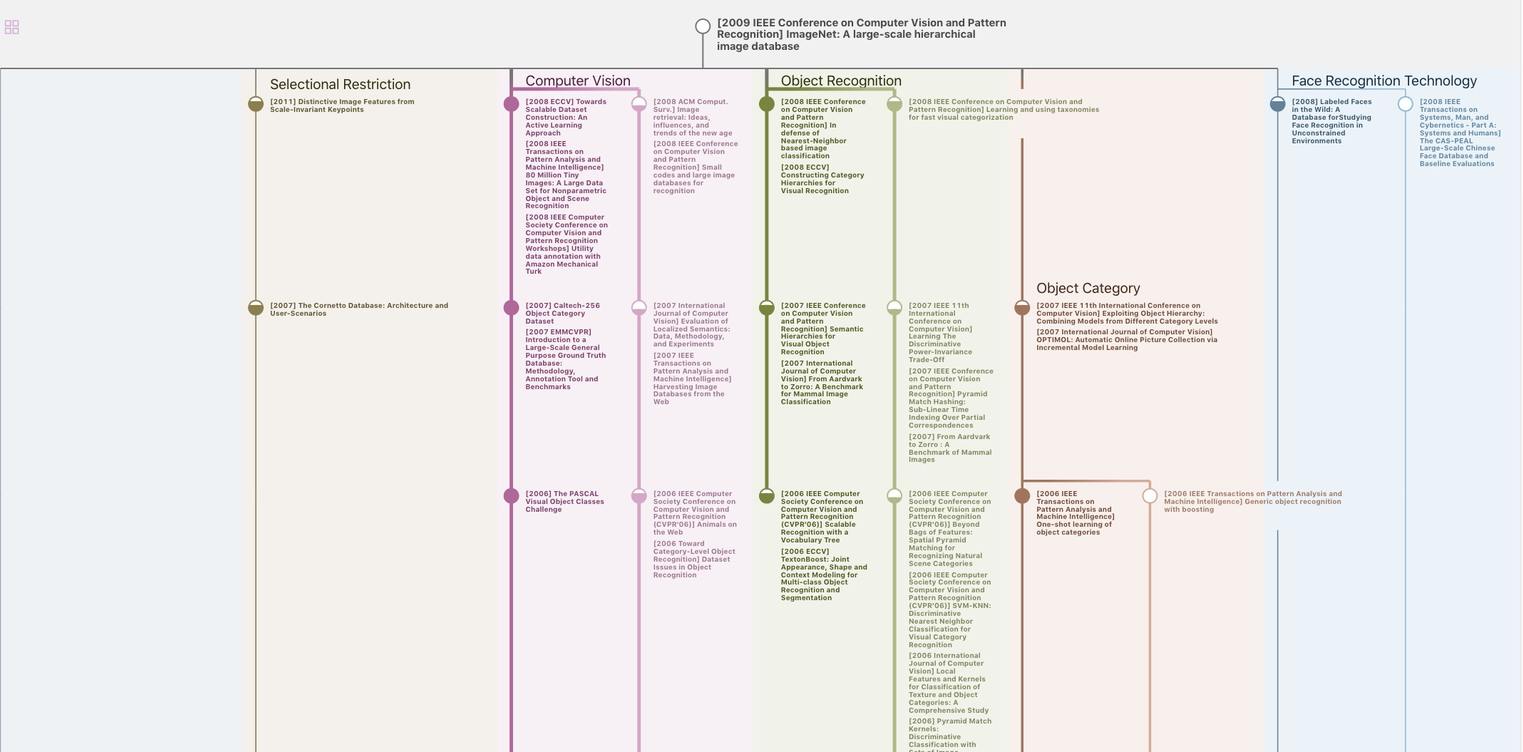
生成溯源树,研究论文发展脉络
Chat Paper
正在生成论文摘要