Prediction of task-based, surgeon efficiency metrics during robotic-assisted minimally invasive surgery
2019 International Symposium on Medical Robotics (ISMR)(2019)
摘要
We present a machine learning algorithm for predicting surgeon performance on future tasks given performance on previous tasks during robotic-assisted surgery. Performance is estimated via six heuristics, called metrics, which are derived from da Vinci Surgical System data streams and which have previously demonstrated significance in differentiating expertise. We use a boosted ensemble of regression trees, which learns a regression from 193 system data features to one of the six target metrics. Predictions from our algorithm are evaluated on whether they achieve significantly lower error than two intelligent guesses: (1) guessing a surgeon performs the future task exactly as how she performed the previous task (GUESS PREV) and (2) guessing a surgeon will have average performance on the future task (GUESS MEAN). We show that our algorithm can predict metrics of future tasks, significantly (p <; 0.05) outperforming GUESS PREV on 19 out of 36 metrics and task pairs and GUESS MEAN on 8 out of 36 metrics and task pairs. This work serves as a first step towards pre-operative performance estimation, where a surgeon can proactively improve performance rather than relying solely on post-operative feedback.
更多查看译文
关键词
machine learning,skills assessment,robotic-assisted surgery,surgeon training
AI 理解论文
溯源树
样例
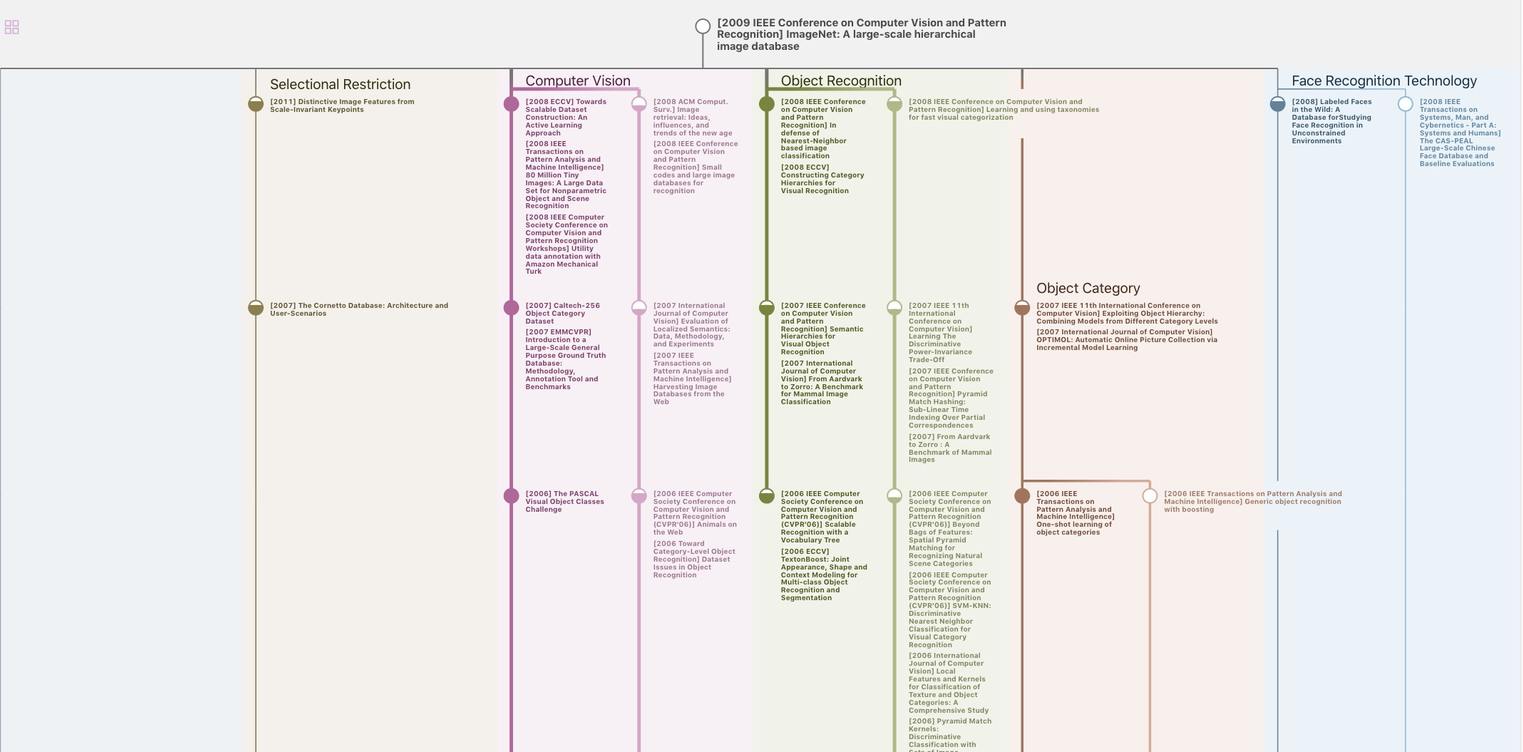
生成溯源树,研究论文发展脉络
Chat Paper
正在生成论文摘要