Quantifying The Spatial Heterogeneity Of Points
INTERNATIONAL JOURNAL OF GEOGRAPHICAL INFORMATION SCIENCE(2019)
摘要
Variation in the spatial heterogeneity of points reflects the evolutionary process or mechanism of geographical events. The key to depicting this variation is quantifying spatial heterogeneity. In this paper, the spatial heterogeneity of a point pattern is defined as the degree of aggregation-type deviation from complete spatial randomness. In such a case, a goodness-of-fit-type statistic based on the distribution of nearest-neighbor distances called the level of heterogeneity (LH*) is regarded as a standard measurement, and a normalized version called the normalized level of heterogeneity (NLH*) is proposed for datasets with different point numbers and study region areas. Considering the complex integration calculation of LH* and NLH*, simulation experiments are implemented to test the capability of some classic nearest-neighbor statistics in quantifying spatial heterogeneity. The results showed that except for the standard LH* statistic, only Clark and Evans' statistic (A-w) and Byth and Ripley's statistic (H-xw) are robust. Statistics NLH*, (A-w) and (H-xw) are validated by quantifying the spatial heterogeneity of two-dimensional crime events, three-dimensional earthquake events and four-dimensional origin-destination (OD) events. The results indicate that these statistics all have a reasonable explanation in quantifying spatial heterogeneity for real-world geographical events of different types and with different dimensions. Compared with NLH*, Clark and Evans' (A-w) statistic and Byth and Ripley's (H-xw) statistic are recommended from the perspective of accessibility.
更多查看译文
关键词
Point pattern, spatial heterogeneity, spatial statistics, nearest-neighbor statistics
AI 理解论文
溯源树
样例
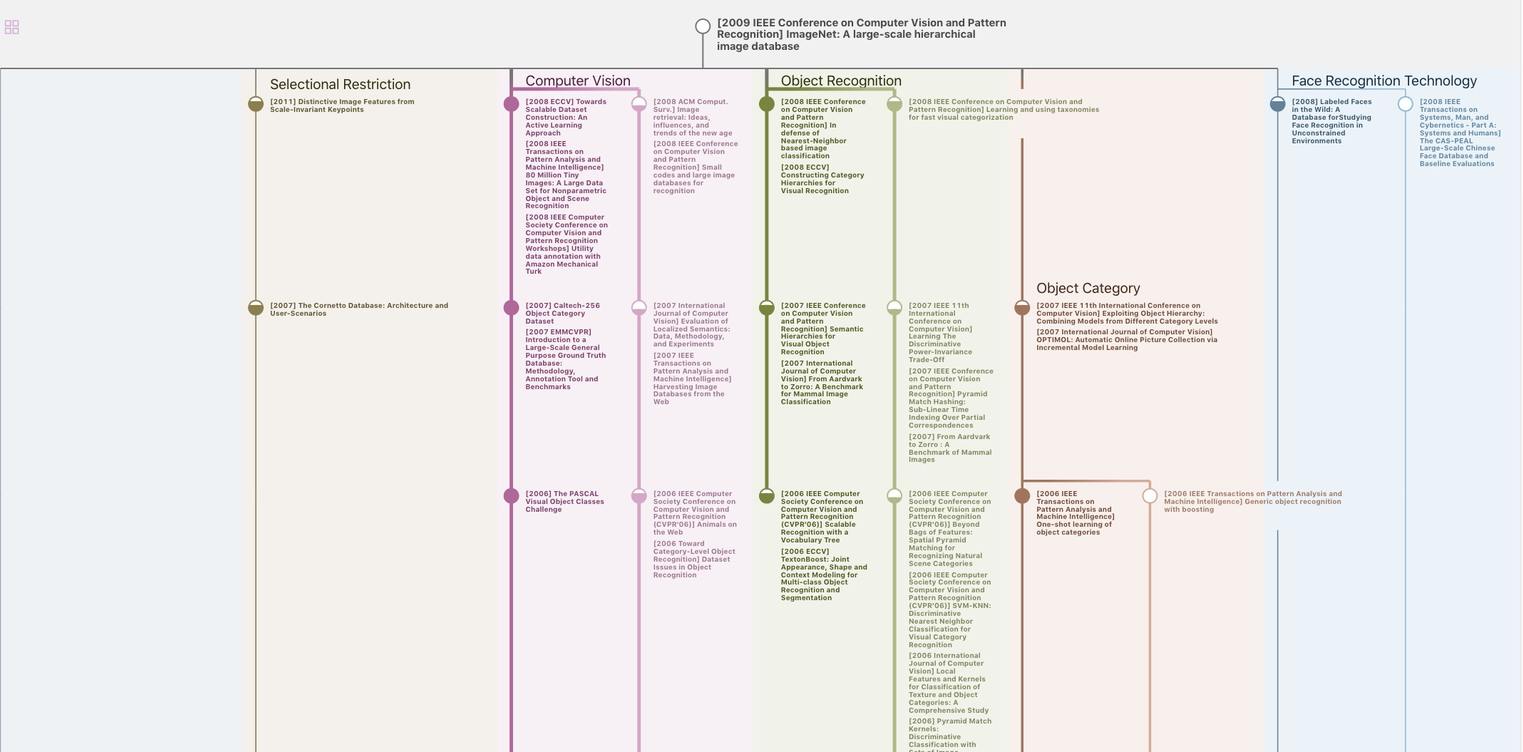
生成溯源树,研究论文发展脉络
Chat Paper
正在生成论文摘要