Integrating Bayesian and Neural Networks for Discourse Coherence
Companion Proceedings of The 2019 World Wide Web Conference(2019)
摘要
In dialogue systems, discourse coherence is an important concept that measures semantic relevance between an utterance and its context. It plays a critical role in determining the inappropriate reply of dialogue systems with regard to a given dialogue context. In this paper, we present a novel framework for evaluating discourse coherence by seamlessly integrating Bayesian and neural networks. The Bayesian network corresponds to Coherence-Pivoted Latent Dirichlet Allocation (cpLDA). cpLDA concentrates on generating the fine-grained topics from dialogue data and takes both local and global semantics into account. The neural network corresponds to Multi-Hierarchical Coherence Network (MHCN). Coupled with cpLDA, MHCN quantifies discourse coherence between an utterance and its context by comprehensively utilizing original texts, topic distribution and topic embedding. Extensive experiments show that the proposed framework yields superior performance comparing with the state-of-the-art methods.
更多查看译文
关键词
Bayesian Network, Dialogue systems, Discourse Coherence, Global Semantics, Multi-Hierarchical Coherence Network
AI 理解论文
溯源树
样例
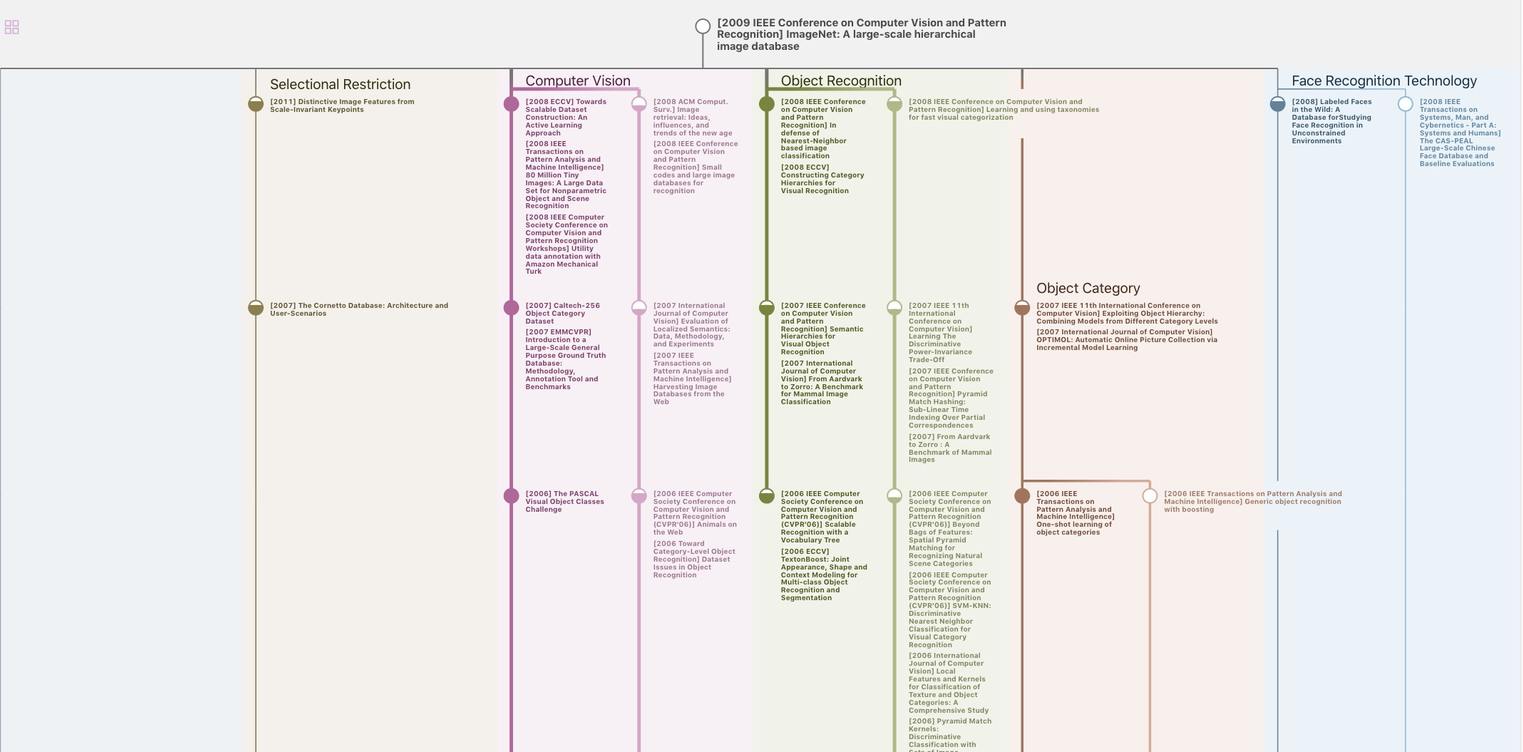
生成溯源树,研究论文发展脉络
Chat Paper
正在生成论文摘要