Joint Learning of Self-Representation and Indicator for Multi-View Image Clustering
2019 IEEE INTERNATIONAL CONFERENCE ON IMAGE PROCESSING (ICIP)(2019)
摘要
Multi-view subspace clustering aims to divide a set of multisource data into several groups according to their underlying subspace structure. Although the spectral clustering based methods achieve promotion in multi-view clustering, their utility is limited by the separate learning manner in which affinity matrix construction and cluster indicator estimation are isolated. In this paper, we propose to jointly learn the self-representation, continue and discrete cluster indicators in an unified model. Our model can explore the subspace structure of each view and fusion them to facilitate clustering simultaneously. Experimental results on two benchmark datasets demonstrate that our method outperforms other existing competitive multi-view clustering methods.
更多查看译文
关键词
Multi-view Clustering,Subspace Clustering,Self-representation Learning
AI 理解论文
溯源树
样例
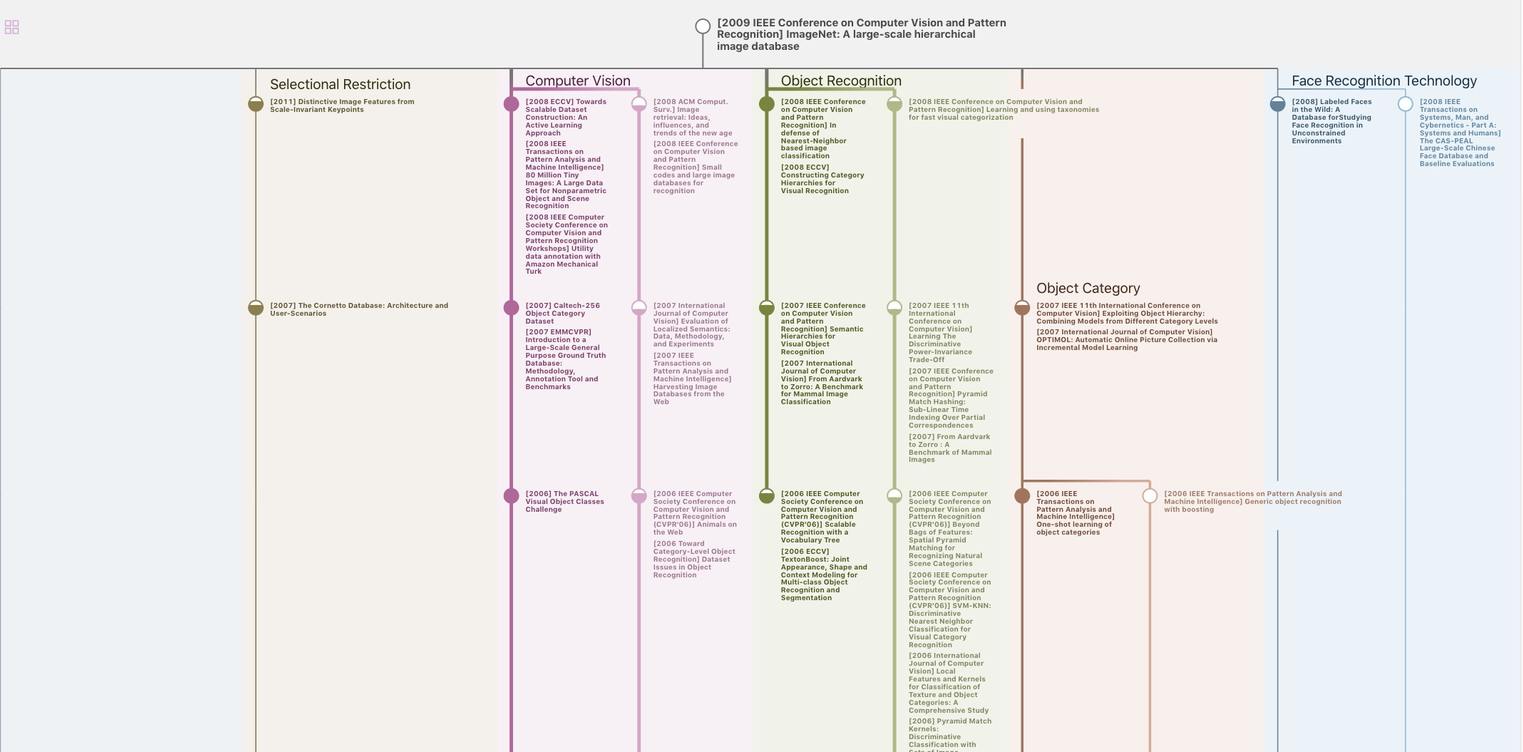
生成溯源树,研究论文发展脉络
Chat Paper
正在生成论文摘要