Learning in Structured MDPs with Convex Cost Functions: Improved Regret Bounds for Inventory Management
OPERATIONS RESEARCH(2022)
摘要
We consider a stochastic inventory control problem under censored demand, lost sales, and positive lead times. This is a fundamental problem in inventory management, with significant literature establishing near optimality of a simple class of policies called "base-stock policies" as well as the convexity of long-run average cost under those policies. We consider a relatively less studied problem of designing a learning algorithm for this problem when the underlying demand distribution is unknown. The goal is to bound the regret of the algorithm when compared with the best base-stock policy. Our main contribution is a learning algorithm with a regret bound of (O) over tilde ((L + 1)root T + D) for the inventory control problem. Here, L >= 0 is the fixed and known lead time, and D is an unknown parameter of the demand distribution described roughly as the expected number of time steps needed to generate enough demand to deplete one unit of inventory. Notably, our regret bounds depend linearly on L, which significantly improves the previously best-known regret bounds for this problem where the dependence on L was exponential. Our techniques utilize the convexity of the long-run average cost and a newly derived bound on the "bias" of base-stock policies to establish an almost black box connection between the problem of learning inMarkov decision processes (MDPs) with these properties and the stochastic convex bandit problem. The techniques presented heremay be of independent interest for other settings that involve large structuredMDPs butwith convex asymptotic average cost functions.
更多查看译文
关键词
inventory control problem,censored demand,reinforcement learning,online convex optimization,regret bounds
AI 理解论文
溯源树
样例
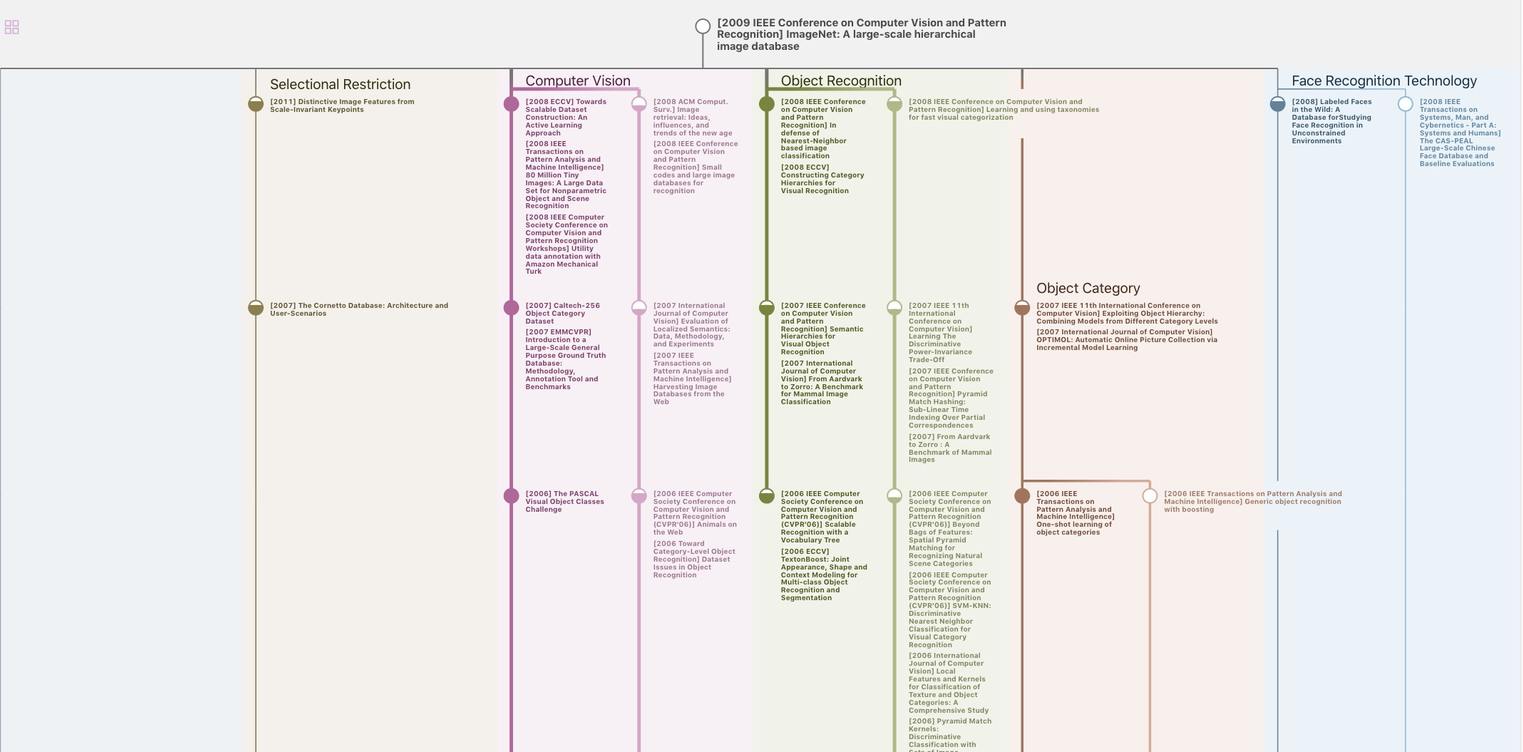
生成溯源树,研究论文发展脉络
Chat Paper
正在生成论文摘要