Online latent semantic hashing for cross-media retrieval.
Pattern Recognition(2019)
摘要
Hashing based cross-media method has been become an increasingly popular technique in facilitating large-scale multimedia retrieval task, owing to its effectiveness and efficiency. Most existing cross-media hashing methods learn hash functions in a batch based mode. However, in practical applications, data points often emerge in a streaming manner, which makes batch based hashing methods loss their efficiency. In this paper, we propose an Online Latent Semantic Hashing (OLSH) method to address this issue. Only newly arriving multimedia data points are utilized to retrain hash functions efficiently and meanwhile preserve the semantic correlations in old data points. Specifically, for learning discriminative hash codes, discrete labels are mapped to a continuous latent semantic space where the relative semantic distances in data points can be measured more accurately. And then, we propose an online optimization scheme towards the challenging task of learning hash functions efficiently on streaming data points, and the computational complexity and memory cost are much less than the size of training dataset at each round. Extensive experiments across many real-world datasets, e.g. Wiki, Mir-Flickr25K and NUS-WIDE, show the effectiveness and efficiency of the proposed method.
更多查看译文
关键词
Cross-media retrieval,Online learning,Hashing,Latent semantic concept
AI 理解论文
溯源树
样例
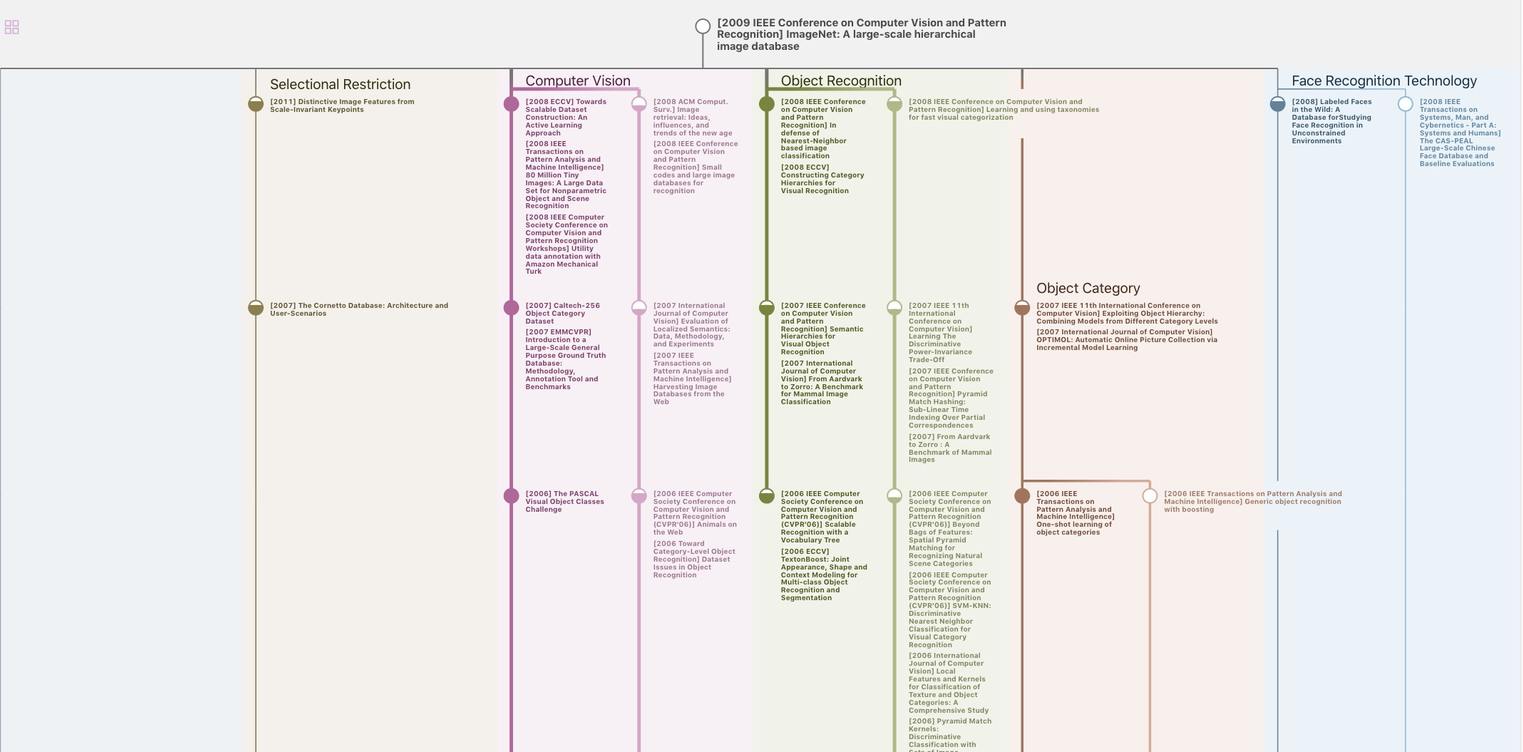
生成溯源树,研究论文发展脉络
Chat Paper
正在生成论文摘要