Another look at matrix correlations.
BIOINFORMATICS(2019)
摘要
Motivation: High throughput technologies are widely employed in modern biomedical research. They yield measurements of a large number of biomolecules in a single experiment. The number of experiments usually is much smaller than the number of measurements in each experiment. The simultaneous measurements of biomolecules provide a basis for a comprehensive, systems view for describing relevant biological processes. Often it is necessary to determine correlations between the data matrices under different conditions or pathways. However, the techniques for analyzing the data with a low number of samples for possible correlations within or between conditions are still in development. Earlier developed correlative measures, such as the RV coefficient, use the trace of the product of data matrices as the most relevant characteristic. However, a recent study has shown that the RV coefficient consistently overestimates the correlations in the case of low sample numbers. To correct for this bias, it was suggested to discard the diagonal elements of the outer products of each data matrix. In this work, a principled approach based on the matrix decomposition generates three trace-independent parts for every matrix. These components are unique, and they are used to determine different aspects of correlations between the original datasets. Results: Simulations show that the decomposition results in the removal of high correlation bias and the dependence on the sample number intrinsic to the RV coefficient. We then use the correlations to analyze a real proteomics dataset.
更多查看译文
关键词
Pathway Analysis
AI 理解论文
溯源树
样例
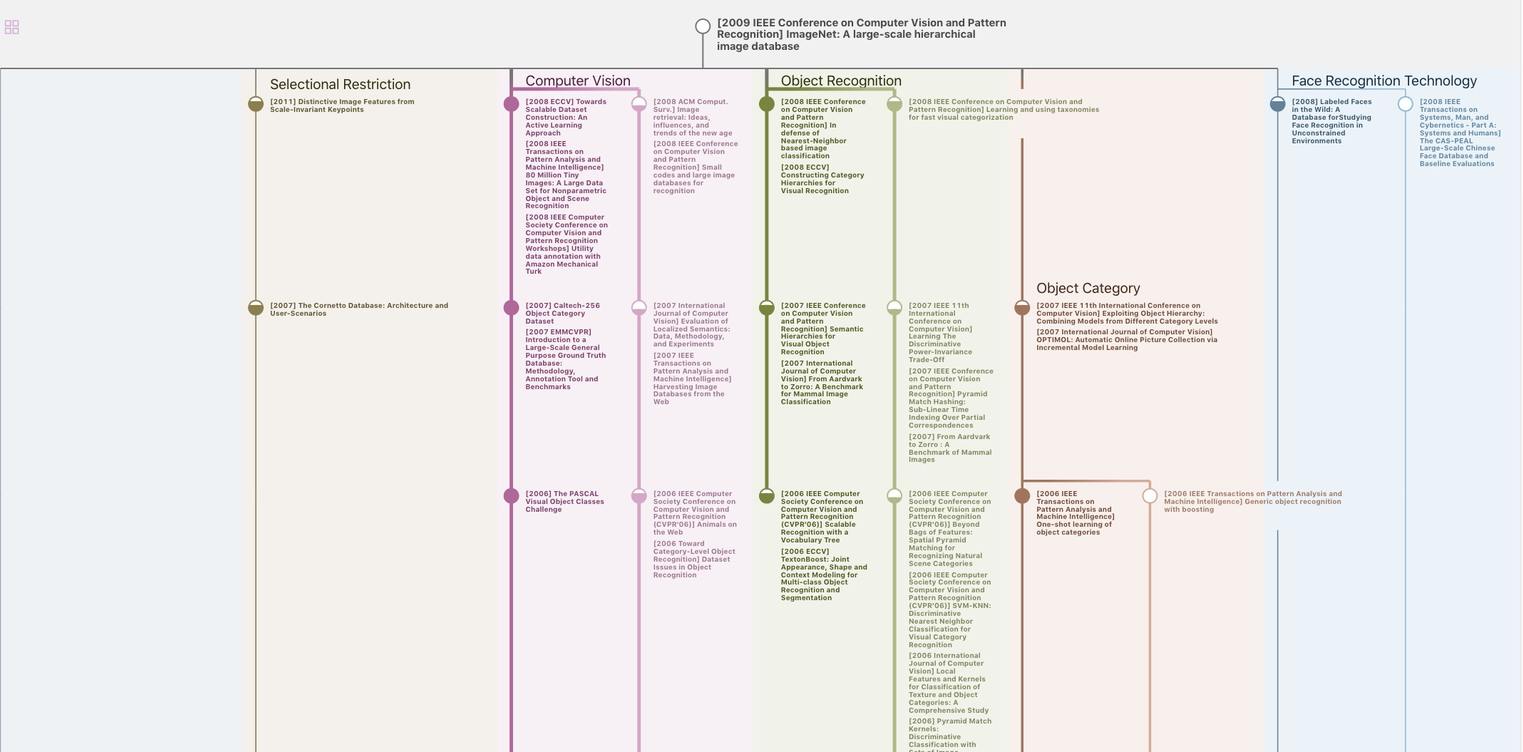
生成溯源树,研究论文发展脉络
Chat Paper
正在生成论文摘要