Tractography and machine learning: Current state and open challenges
Magnetic Resonance Imaging(2019)
摘要
Supervised machine learning (ML) algorithms have recently been proposed as an alternative to traditional tractography methods in order to address some of their weaknesses. They can be path-based and local-model-free, and easily incorporate anatomical priors to make contextual and non-local decisions that should help the tracking process. ML-based techniques have thus shown promising reconstructions of larger spatial extent of existing white matter bundles, promising reconstructions of less false positives, and promising robustness to known position and shape biases of current tractography techniques. But as of today, none of these ML-based methods have shown conclusive performances or have been adopted as a de facto solution to tractography. One reason for this might be the lack of well-defined and extensive frameworks to train, evaluate, and compare these methods.
更多查看译文
关键词
Diffusion MRI,Tractography,Machine learning,Benchmark
AI 理解论文
溯源树
样例
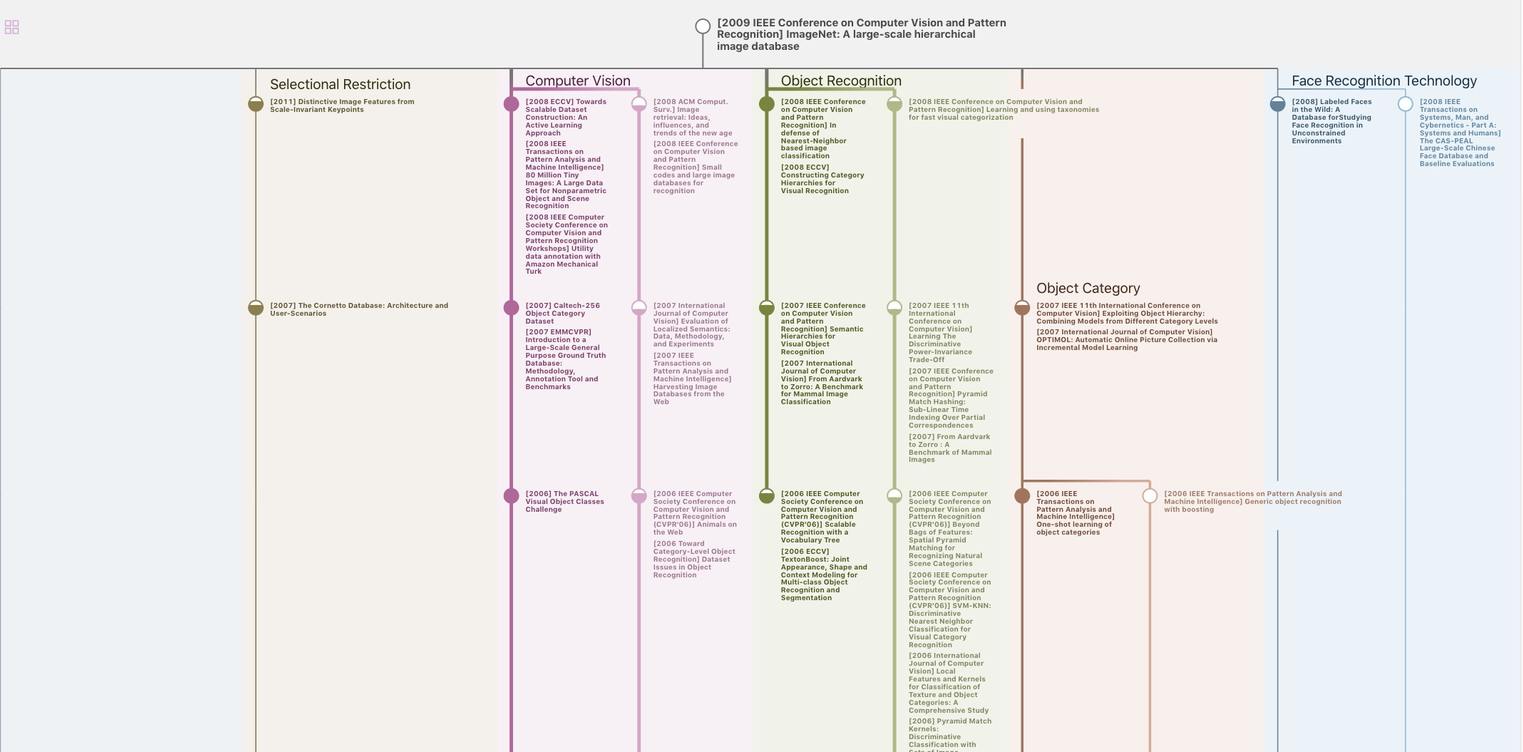
生成溯源树,研究论文发展脉络
Chat Paper
正在生成论文摘要