Reconciliation k-median: Clustering with Non-polarized Representatives
WWW '19: The Web Conference on The World Wide Web Conference WWW 2019(2019)
摘要
We propose a new variant of the k-median problem, where the objective function models not only the cost of assigning data points to cluster representatives, but also a penalty term for disagreement among the representatives. We motivate this novel problem by applications where we are interested in clustering data while avoiding selecting representatives that are too far from each other. For example, we may want to summarize a set of news sources, but avoid selecting ideologically-extreme articles in order to reduce polarization.
To solve the proposed k-median formulation we adopt the local-search algorithm of Arya et al. [2], We show that the algorithm provides a provable approximation guarantee, which becomes constant under a mild assumption on the minimum number of points for each cluster. We experimentally evaluate our problem formulation and proposed algorithm on datasets inspired by the motivating applications. In particular, we experiment with data extracted from Twitter, the US Congress voting records, and popular news sources. The results show that our objective can lead to choosing less polarized groups of representatives without significant loss in representation fidelity.
更多查看译文
关键词
Clustering, approximation algorithms., committee selection, data mining, k-median, polarization
AI 理解论文
溯源树
样例
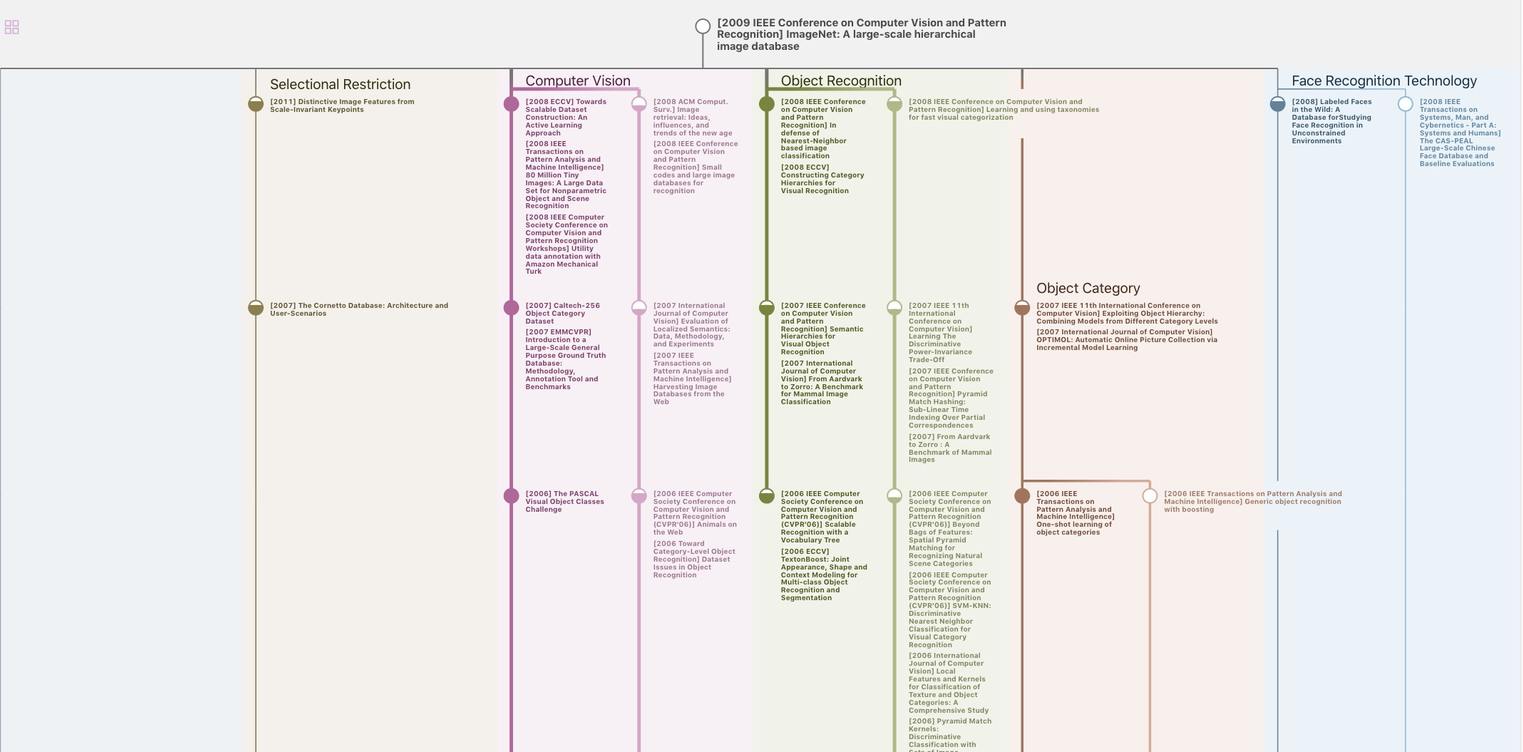
生成溯源树,研究论文发展脉络
Chat Paper
正在生成论文摘要