Click Feedback-Aware Query Recommendation Using Adversarial Examples
WWW '19: The Web Conference San Francisco CA USA May, 2019(2019)
摘要
Search engine users always endeavor to formulate proper search queries during online search. To help users accurately express their information need during search, search engines are equipped with query suggestions to refine users' follow-up search queries. The success of a query suggestion system counts on whether we can understand and model user search intent accurately. In this work, we propose Click Feedback-Aware Network (CFAN) to provide feedback-aware query suggestions. In addition to modeling sequential search queries issued by a user, CFAN also considers user clicks on previous suggested queries as the user feedback. These clicked suggestions, together with the issued search query sequence, jointly capture the underlying search intent of users. In addition, we explicitly focus on improving the robustness of the query suggestion system through adversarial training. Adversarial examples are introduced into the training of the query suggestion system, which not only improves the robustness of system to nuisance perturbations, but also enhances the generalization performance for original training data. Extensive experiments are conducted on a recent real search engine log. The experimental results demonstrate that the proposed method, CFAN, outperforms competitive baseline methods across various situations on the task of query suggestion.
更多查看译文
关键词
Query recommendation, adversarial example, context-aware
AI 理解论文
溯源树
样例
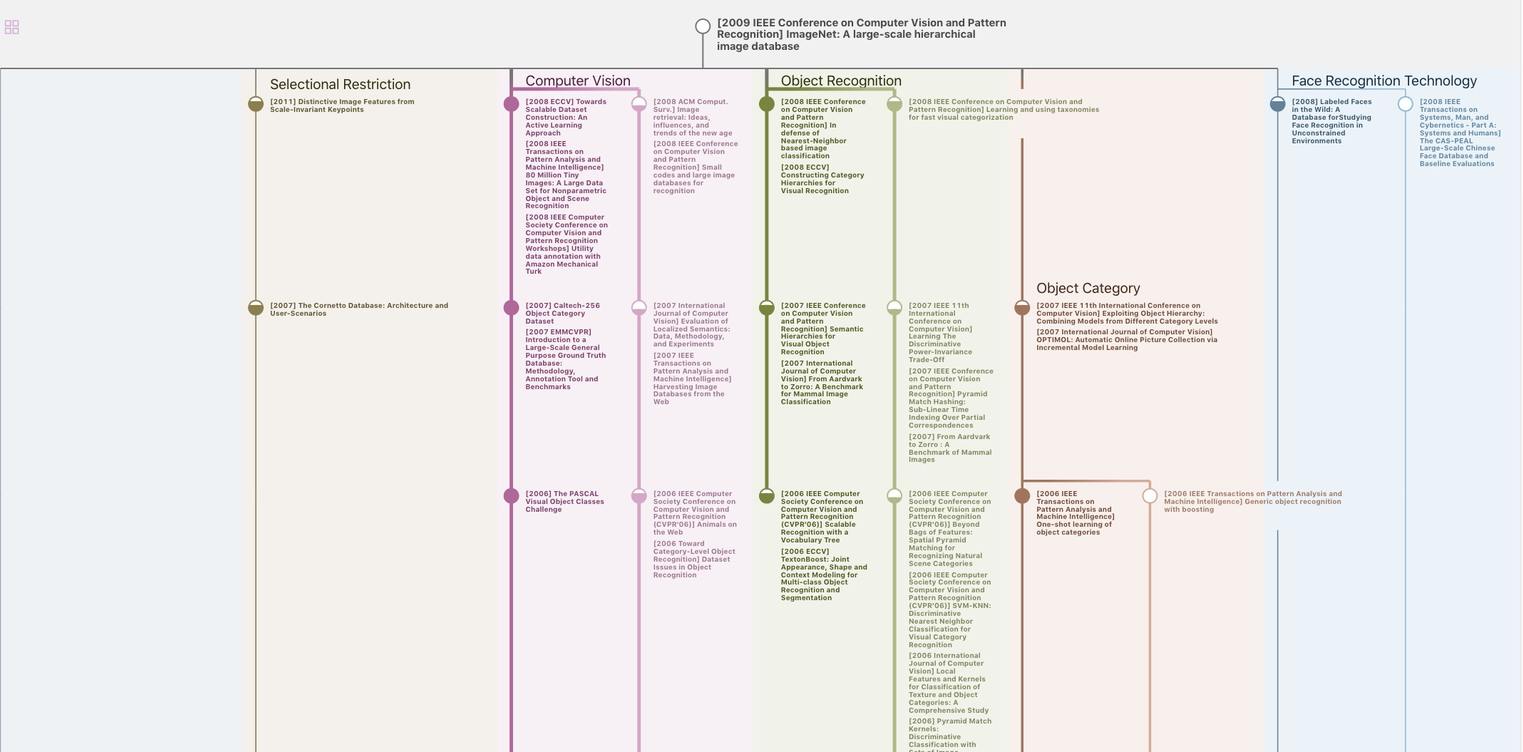
生成溯源树,研究论文发展脉络
Chat Paper
正在生成论文摘要