A Novel Unsupervised Approach for Precise Temporal Slot Filling from Incomplete and Noisy Temporal Contexts
WWW '19: The Web Conference San Francisco CA USA May, 2019(2019)
摘要
The task of temporal slot filling (TSF) is to extract the values (or called facts) of specific attributes for a given entity from text data and find the time points when the values were valid. It is challenging to find precise time points with incomplete and noisy temporal contexts in the text. In this work, we propose an unsupervised approach of two modules that mutually enhance each other: one is a reliability estimator on fact extractors conditionally to the temporal contexts; the other is a fact trustworthiness estimator based on the extractor's reliability. The iterative learning process reduces the noise of the extractions. Experiments demonstrate that our approach, with the novel design, can accurately and efficiently extract precise temporal facts from newspaper corpora.
更多查看译文
关键词
Incompleteness, Information extraction, Temporal slot filling
AI 理解论文
溯源树
样例
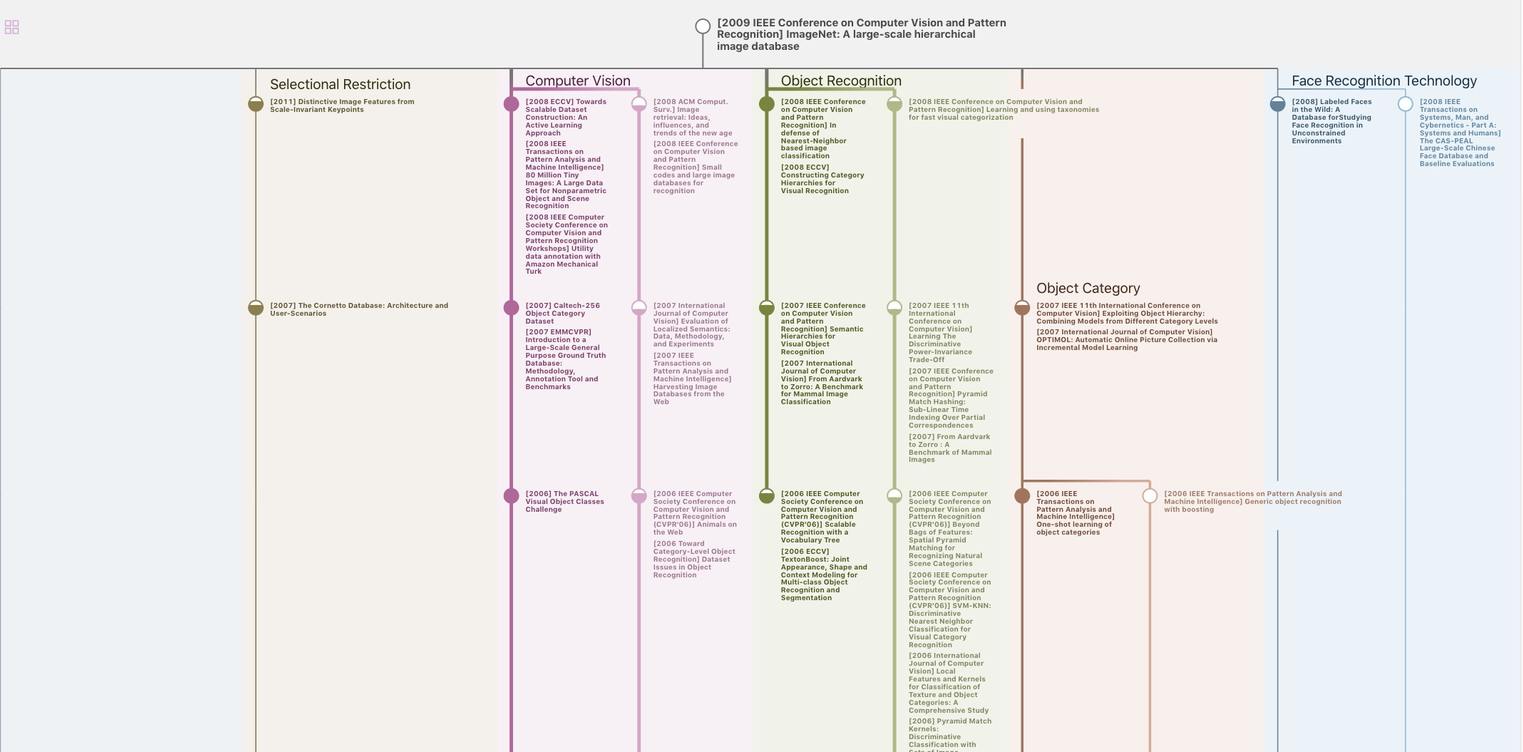
生成溯源树,研究论文发展脉络
Chat Paper
正在生成论文摘要