Neural 3D Morphable Models: Spiral Convolutional Networks for 3D Shape Representation Learning and Generation
2019 IEEE/CVF INTERNATIONAL CONFERENCE ON COMPUTER VISION (ICCV 2019)(2019)
摘要
Generative models for 3D geometric data arise in many important applications in 3D computer vision and graphics. In this paper, we focus on 3D deformable shapes that share a common topological structure, such as human faces and bodies. Morphable Models were among the first attempts to create compact representations for such shapes; despite their effectiveness and simplicity, such models have limited representation power due to their linear formulation. Recently, non-linear learnable methods have been proposed, although most of them resort to intermediate representations, such as 3D grids of voxels or 2D views. In this paper, we introduce a convolutional mesh autoencoder and a GAN architecture based on the spiral convolutional operator, acting directly on the mesh and leveraging its underlying geometric structure. We provide an analysis of our convolution operator and demonstrate state-of-the-art results on 3D shape datasets compared to the linear Morphable Model and the recently proposed COMA model.
更多查看译文
关键词
3D voxel grids,fixed underlying graph,local orderings,spiral operator,permutation invariance property,graph neural networks,deep generative architectures,3D shape datasets,graph convolutional operators,spiral convolutional networks,generative models,3D geometric data,3D deformable shapes,topological structure,human faces,linear formulation,intermediate representations,linear morphable model,topology-aware operator,graph convolutional operator,3D computer vision,3D mesh,graph vertices,3D shape representation learning
AI 理解论文
溯源树
样例
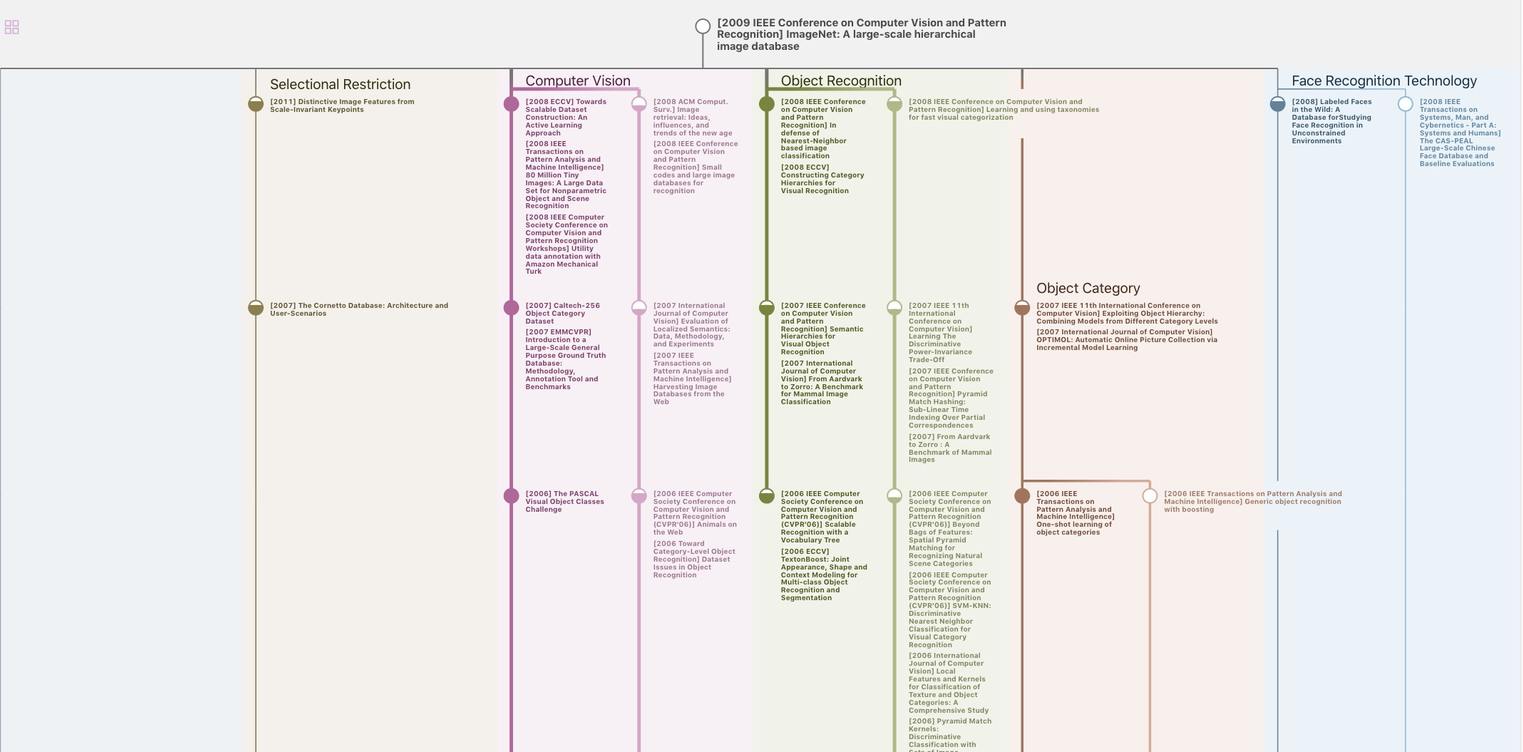
生成溯源树,研究论文发展脉络
Chat Paper
正在生成论文摘要