Perceptually Inspired Normalized Conditional Compression Distance
CONFERENCE RECORD OF THE 2019 FIFTY-THIRD ASILOMAR CONFERENCE ON SIGNALS, SYSTEMS & COMPUTERS(2019)
摘要
Image similarity measurement is a common issue in a broad range of applications in image processing, recognition, classification and retrieval. Conventional image similarity measures are often limited to specific applications and cannot be applied in general scenarios. The theory of Kolmogorov complexity provides a universal framework for a generic similarity metric based on information distance between objects. Normalized Information Distance (NID) has been shown to be a valid and universal distance metric applicable in measurement of similarity of any two objects, and has been successfully applied to a wide range of applications in the past. The difficulty of NID lies in the non-computable nature of the Kolmogorov complexity, and thus approximation has to be applied in practice. Here we propose a perceptually-inspired Normalized Conditional Compression Distance (NCCD) measure by using the Divisive Normalization Transform (DNT) as a means to model the non-linear behavior of the Human Visual System (HVS) in reducing statistical dependencies of visual signals for efficient representation, and show that this perceptual extension of NID can be used in a wide range of image processing applications, including texture classification and face recognition.
更多查看译文
关键词
mage Similarity Measurement, Kolmogorov complexity, Normalized Information Distance, Compression, Divisive Normalization, Classification, Recognition mage Similarity Measurement, Kolmogorov complexity, Normalized Information Distance, Compression, Divisive Normalization, Classification, Recognition I
AI 理解论文
溯源树
样例
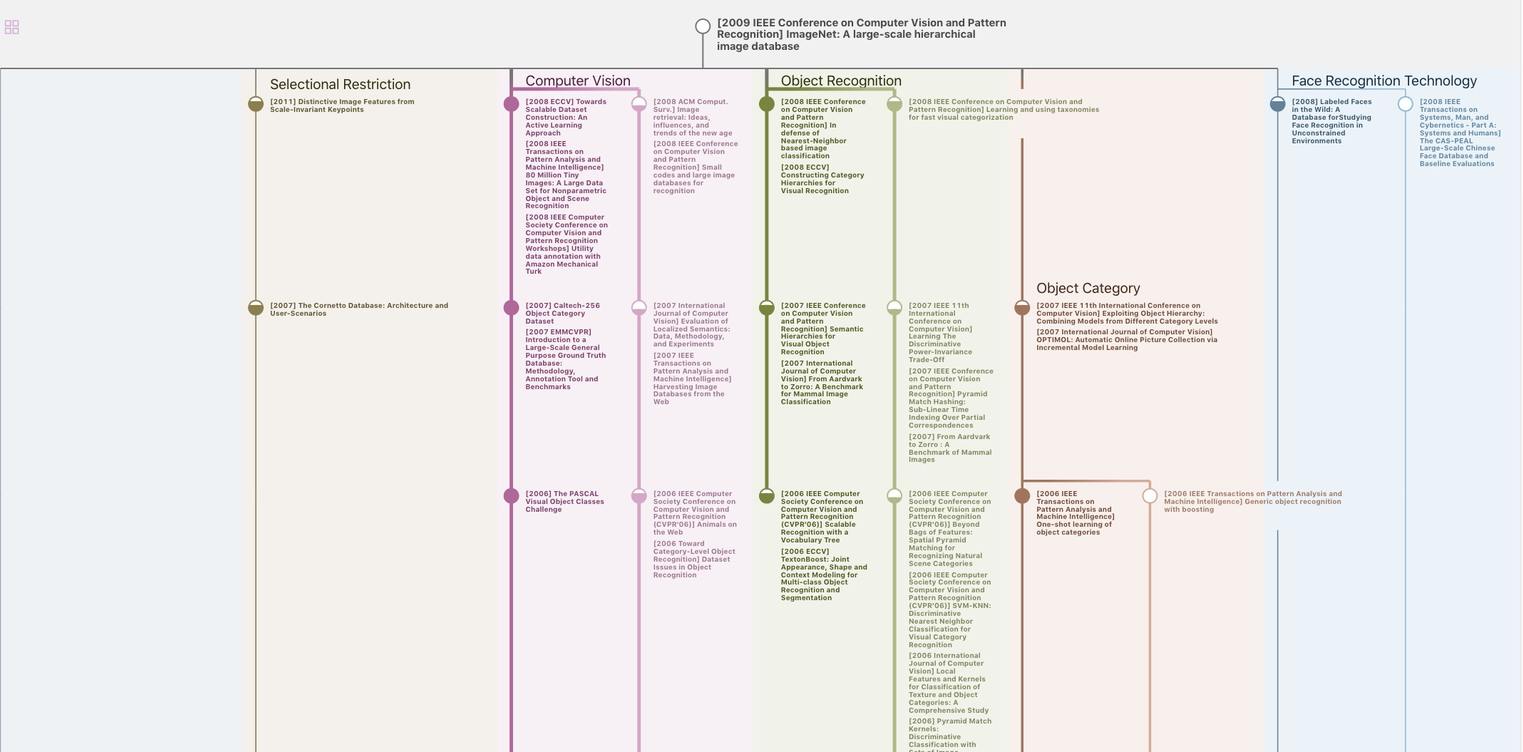
生成溯源树,研究论文发展脉络
Chat Paper
正在生成论文摘要