Hessian barrier algorithms for linearly constrained optimization problems
SIAM JOURNAL ON OPTIMIZATION(2019)
摘要
In this paper, we propose an interior-point method for linearly constrained and possibly nonconvex-optimization problems. The method which we call the Hessian barrier algorithm (HBA)-combines a forward Euler discretization of Hessian-Riemannian gradient flows with an Armijo backtracking step-size policy. In this way, HBA can be seen as an alternative to mirror descent, and contains as special cases the affine scaling algorithm, regularized Newton processes, and several other iterative solution methods. Our main result is that, modulo a nondegeneracy condition, the algorithm converges to the problem's critical set; hence, in the convex case, the algorithm converges globally to the problem's minimum set. In the case of linearly constrained quadratic programs (not necessarily convex), we also show that the method's convergence rate is O(1/k rho) for some rho is an element of (0, 1] that depends only on the choice of kernel function (i.e., not on the problem's primitives). These theoretical results are validated by numerical experiments in standard nonconvex test functions and large-scale traffic assignment problems.
更多查看译文
关键词
Hessian-Riemannian gradient descent,interior-point methods,mirror descent,nonconvex optimization,traffic assignment
AI 理解论文
溯源树
样例
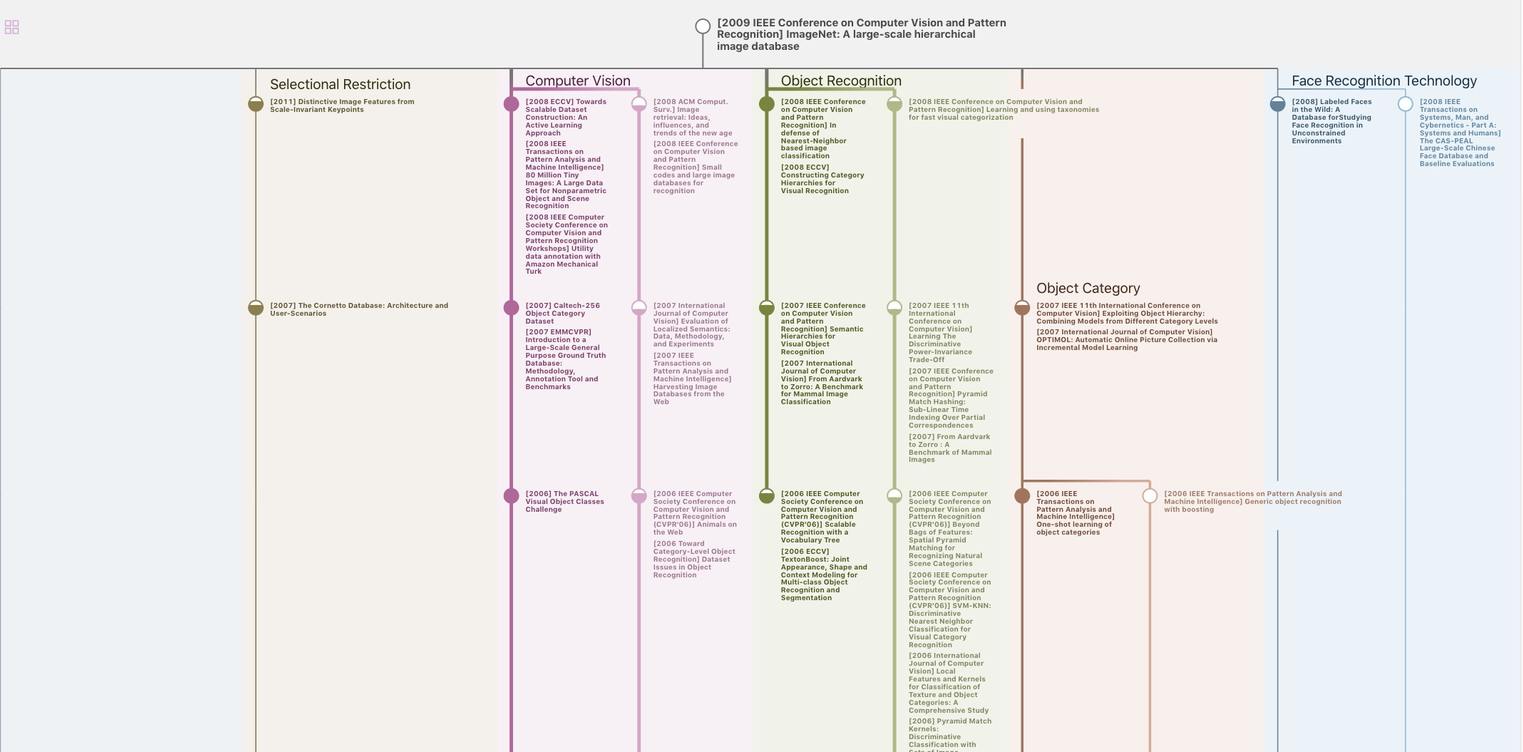
生成溯源树,研究论文发展脉络
Chat Paper
正在生成论文摘要