Counting Strategies for the Probabilistic Description Logic ALC\mathsf ME Under the Principle of Maximum Entropy.
Lecture Notes in Artificial Intelligence(2019)
摘要
We present ALC(ME), a probabilistic variant of the Description Logic ALC that allows for representing and processing conditional statements of the form "if E holds, then F follows with probability p" under the principle of maximum entropy. Probabilities are understood as degrees of belief and formally interpreted by the aggregating semantics. We prove that both checking consistency and drawing inferences based on approximations of the maximum entropy distribution is possible in ALC(ME) in time polynomial in the domain size. A major problem for probabilistic reasoning from such conditional knowledge bases is to count models and individuals. To achieve our complexity results, we develop sophisticated counting strategies on interpretations aggregated with respect to the so-called conditional impacts of types, which refine their conditional structure.
更多查看译文
关键词
Probabilistic description logics,Aggregating semantics,Principle of maximum entropy,Domain-lifted inference
AI 理解论文
溯源树
样例
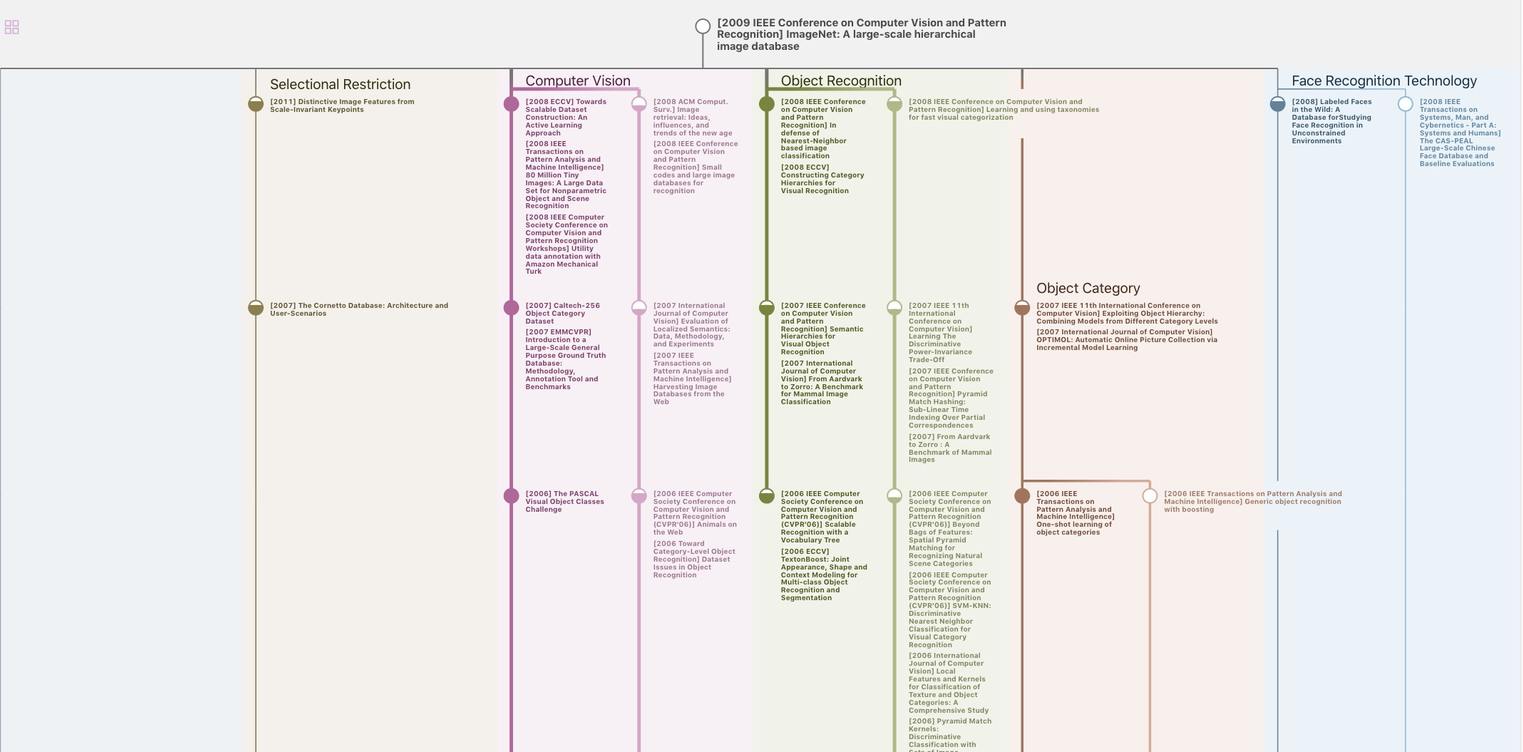
生成溯源树,研究论文发展脉络
Chat Paper
正在生成论文摘要